An efficient Spatial–Temporal model based on gated linear units for trajectory prediction
Neurocomputing(2022)
摘要
Trajectory prediction is a crucial and challenging task in many domains (e.g., autonomous driving and robot navigation). First, high-quality trajectory prediction methods need to capture the human–human interactions and human-scene interactions effectively to avoid collisions with moving agents and static obstacles. Moreover, it is indispensable for the approaches to be efficient and lightweight to reduce computing costs and economize public resources. To address these challenges, we propose a model with a Spatial–Temporal module and a heatmap module based on gated linear units. In the Spatial–Temporal module, an adaptive Graph Convolutional Network was proposed to capture the human–human interactions, which combines physical features with graph convolutional networks to speculate the agents’ implicit relationships. As for the human-scene interaction, we encode the sequential local heatmap around each agent in the heatmap module. The model includes two gated linear units to capture the correlations of the agent’s motion and dynamic changing trend of the surrounding scene, respectively. Compared with previous methods, our method is more lightweight and efficient with a smaller parameter size and shorter inference time. Meanwhile, our model achieves better experimental results on two publicly available datasets (ETH and UCY) and predicts more socially reasonable trajectories.
更多查看译文
关键词
Trajectory prediction,Spatial–Temporal correlation,Graph neural network
AI 理解论文
溯源树
样例
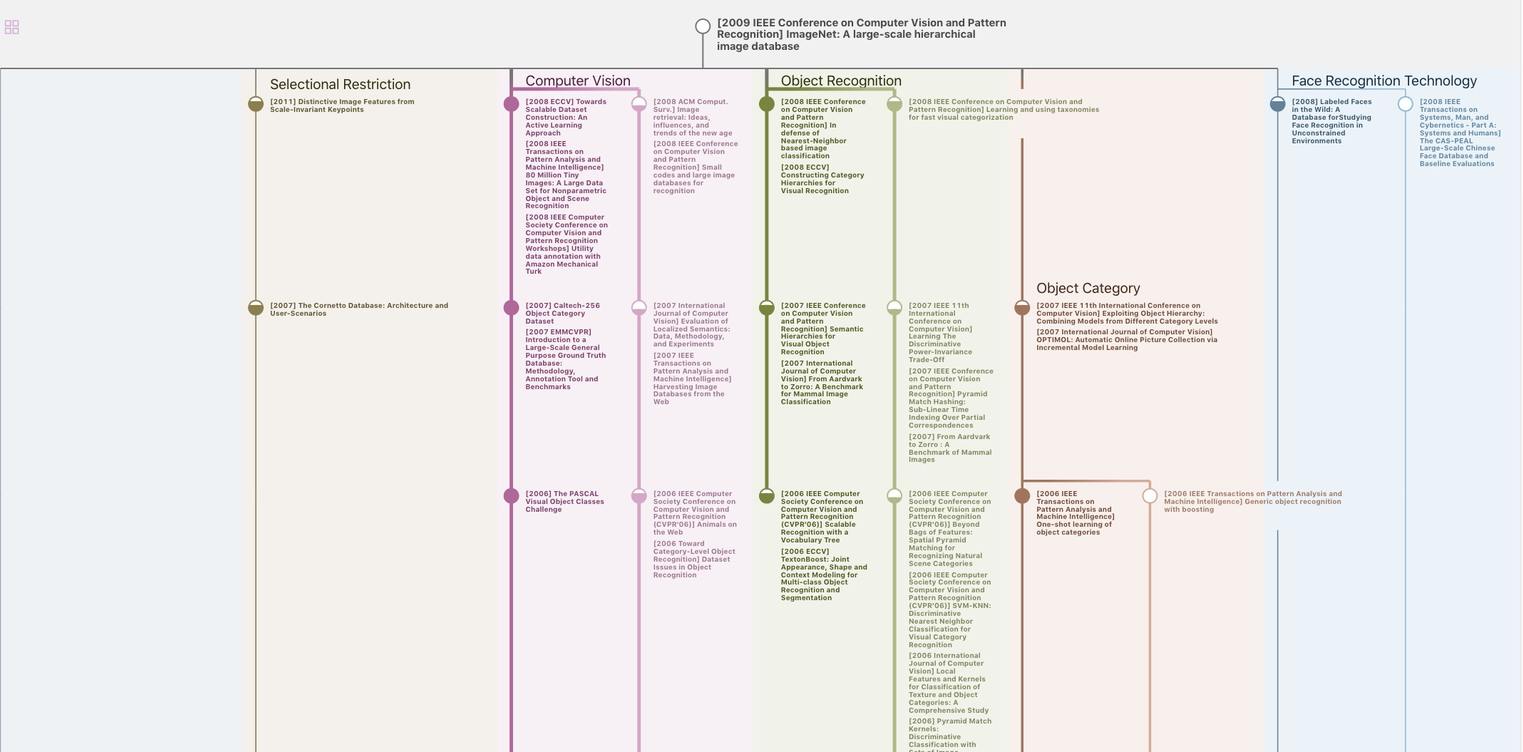
生成溯源树,研究论文发展脉络
Chat Paper
正在生成论文摘要