Forward-reverse adaptive graph convolutional networks for skeleton-based action recognition
Neurocomputing(2022)
摘要
In the latest research based on skeleton data, the graph convolutional networks (GCN) based methods have achieved excellent performance on action recognition tasks. Existing GCN-based methods commonly adopt the strategy of fusing the data flow of joints and bones to obtain better results. However, these approaches ignore the reversibility of the skeleton data in the temporal dimension. Compared with the forward sequences input, which can achieve better results in certain actions through the end-to-end networks, the reverse skeleton data has excellent discrimination and richer information for some specific actions. In this work, we propose the novel forward-reverse adaptive graph convolutional networks (FR-AGCN) for skeleton-based action recognition. The sequences of joints and bones, as well as their reverse information, are modeled in the multi-stream networks at the same time. By extracting the features of forward and reverse deep information and performing multi-stream fusion, this strategy can significantly improve the recognition accuracy. Extensive experiments on two large-scale datasets NTU 60 & 120 show that the performance of our strategies has exciting advantages. On the latest dataset UAV-Human, the proposed FR-AGCN outperforms other state-of-the-art (SOTA) methods. Concretely, compared with 4s Shift-GCN, one of the most advanced models, FR-AGCN obtains significant improvements of +6.0% on CSv1 benchmark and +2.46% on CSv2 benchmark.
更多查看译文
关键词
Skeleton-based action recognition,Graph convolutional networks,FR-AGCN,Multi-stream fusion,Forward and reverse deep information
AI 理解论文
溯源树
样例
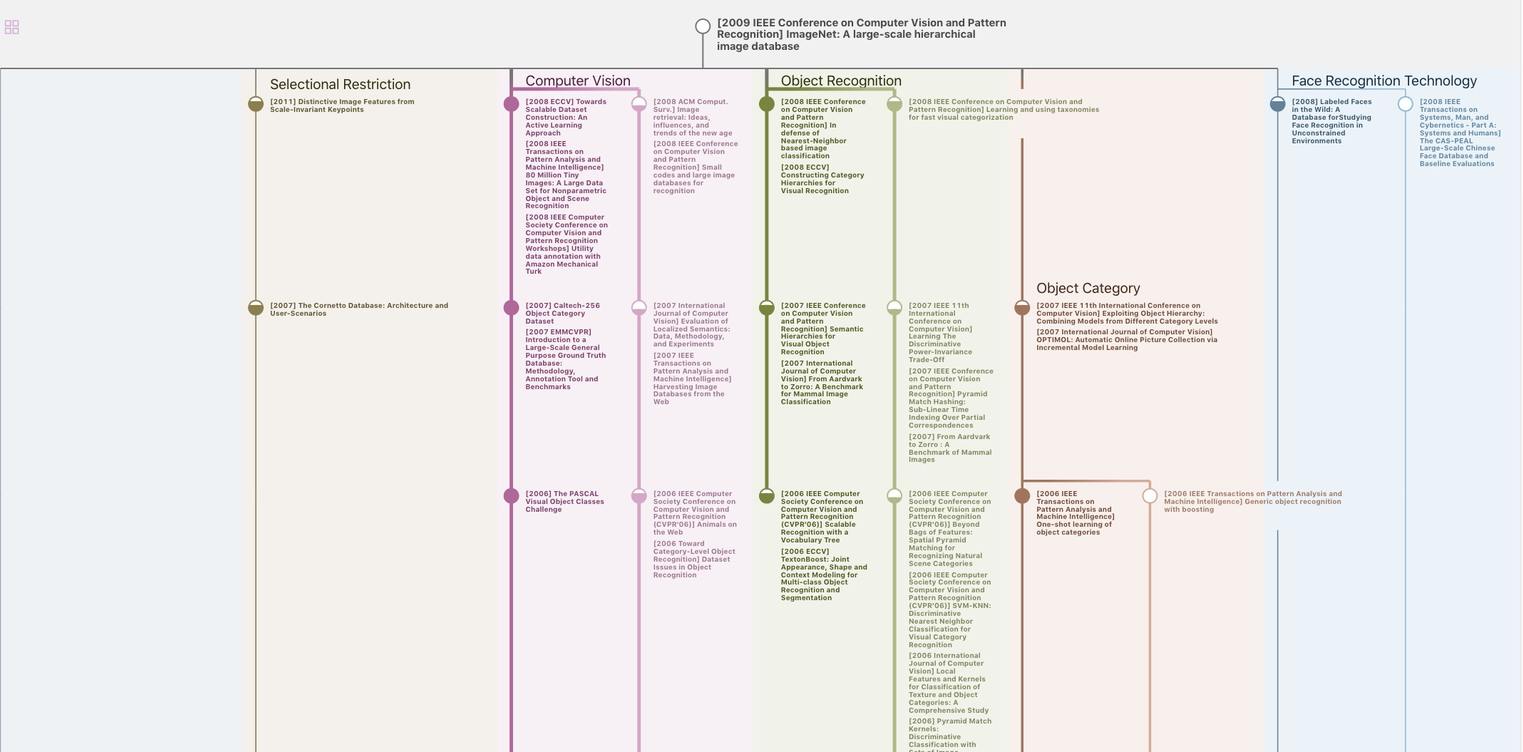
生成溯源树,研究论文发展脉络
Chat Paper
正在生成论文摘要