CATrans: Context and Affinity Transformer for Few-Shot Segmentation.
International Joint Conference on Artificial Intelligence(2022)
摘要
Few-shot segmentation (FSS) aims to segment novel categories given scarce annotated support images. The crux of FSS is how to aggregate dense correlations between support and query images for query segmentation while being robust to the large variations in appearance and context. To this end, previous Transformer-based methods explore global consensus either on context similarity or affinity map between support-query pairs. In this work, we effectively integrate the context and affinity information via the proposed novel Context and Affinity Transformer (CATrans) in a hierarchical architecture. Specifically, the Relation-guided Context Transformer (RCT) propagates context information from support to query images conditioned on more informative support features. Based on the observation that a huge feature distinction between support and query pairs brings barriers for context knowledge transfer, the Relation-guided Affinity Transformer (RAT) measures attention-aware affinity as auxiliary information for FSS, in which the self-affinity is responsible for more reliable cross-affinity. We conduct experiments to demonstrate the effectiveness of the proposed model, outperforming the state-of-the-art methods.
更多查看译文
关键词
Computer Vision: Transfer, low-shot, semi- and un- supervised learning,Computer Vision: Machine Learning for Vision
AI 理解论文
溯源树
样例
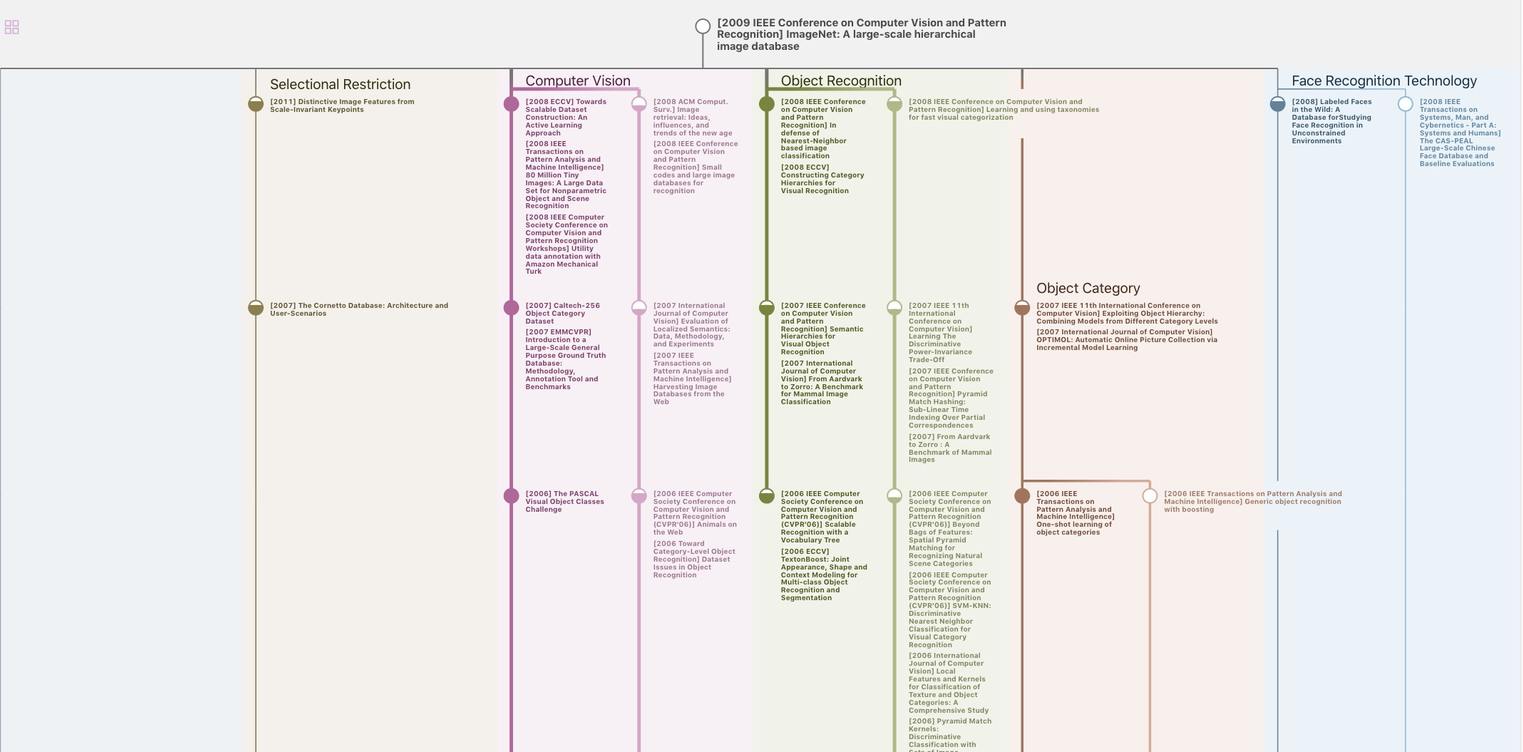
生成溯源树,研究论文发展脉络
Chat Paper
正在生成论文摘要