A Practical Two-stage Ranking Framework for Cross-market Recommendation
arxiv(2022)
摘要
Cross-market recommendation aims to recommend products to users in a resource-scarce target market by leveraging user behaviors from similar rich-resource markets, which is crucial for E-commerce companies but receives less research attention. In this paper, we present our detailed solution adopted in the cross-market recommendation contest, i.e., WSDM CUP 2022. To better utilize collaborative signals and similarities between target and source markets, we carefully consider multiple features as well as stacking learning models consisting of deep graph recommendation models (Graph Neural Network, DeepWalk, etc.) and traditional recommendation models (ItemCF, UserCF, Swing, etc.). Furthermore, We adopt tree-based ensembling methods, e.g., LightGBM, which show superior performance in prediction task to generate final results. We conduct comprehensive experiments on the XMRec dataset, verifying the effectiveness of our model. The proposed solution of our team WSDM_Coggle_ is selected as the second place submission.
更多查看译文
关键词
ranking,recommendation,two-stage,cross-market
AI 理解论文
溯源树
样例
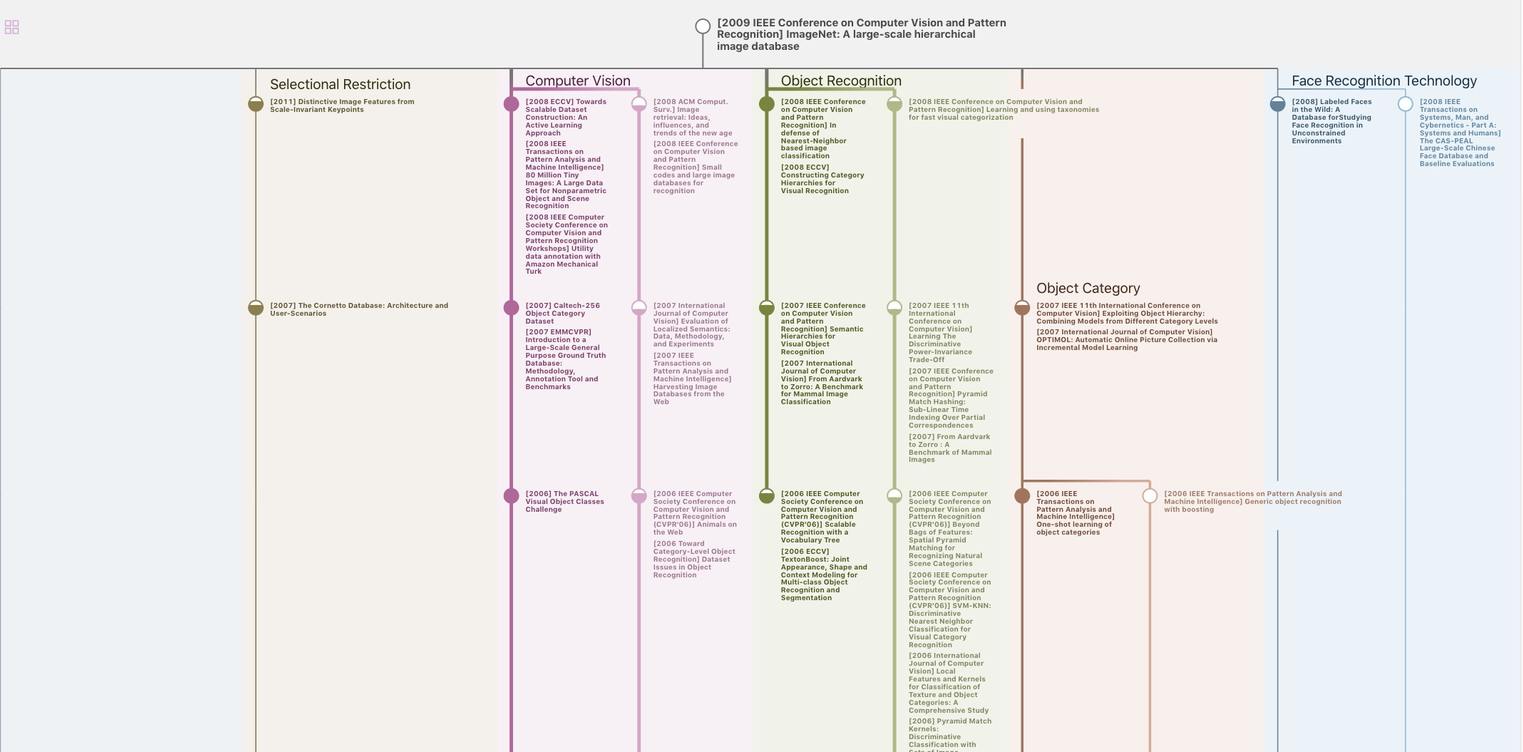
生成溯源树,研究论文发展脉络
Chat Paper
正在生成论文摘要