Understanding a Class of Decentralized and Federated Optimization Algorithms: A Multirate Feedback Control Perspective.
arxiv(2023)
摘要
Distributed algorithms have been playing an increasingly important role in many applications such as machine learning, signal processing, and control. Significant research efforts have been devoted to developing and analyzing new algorithms for various applications. In this work, we provide a fresh perspective to understand, analyze, and design distributed optimization algorithms. Through the lens of multirate feedback control, we show that a wide class of distributed algorithms, including popular decentralized/federated schemes, can be viewed as discretizing a certain continuous-time feedback control system, possibly with multiple sampling rates, such as decentralized gradient descent, gradient tracking, and federated averaging. This key observation not only allows us to develop a generic framework to analyze the convergence of the entire algorithm class, but, more importantly, it also leads to an interesting way of designing new distributed algorithms. We develop the theory behind our framework and provide examples to highlight how the framework can be used in practice.
更多查看译文
关键词
federated optimization
AI 理解论文
溯源树
样例
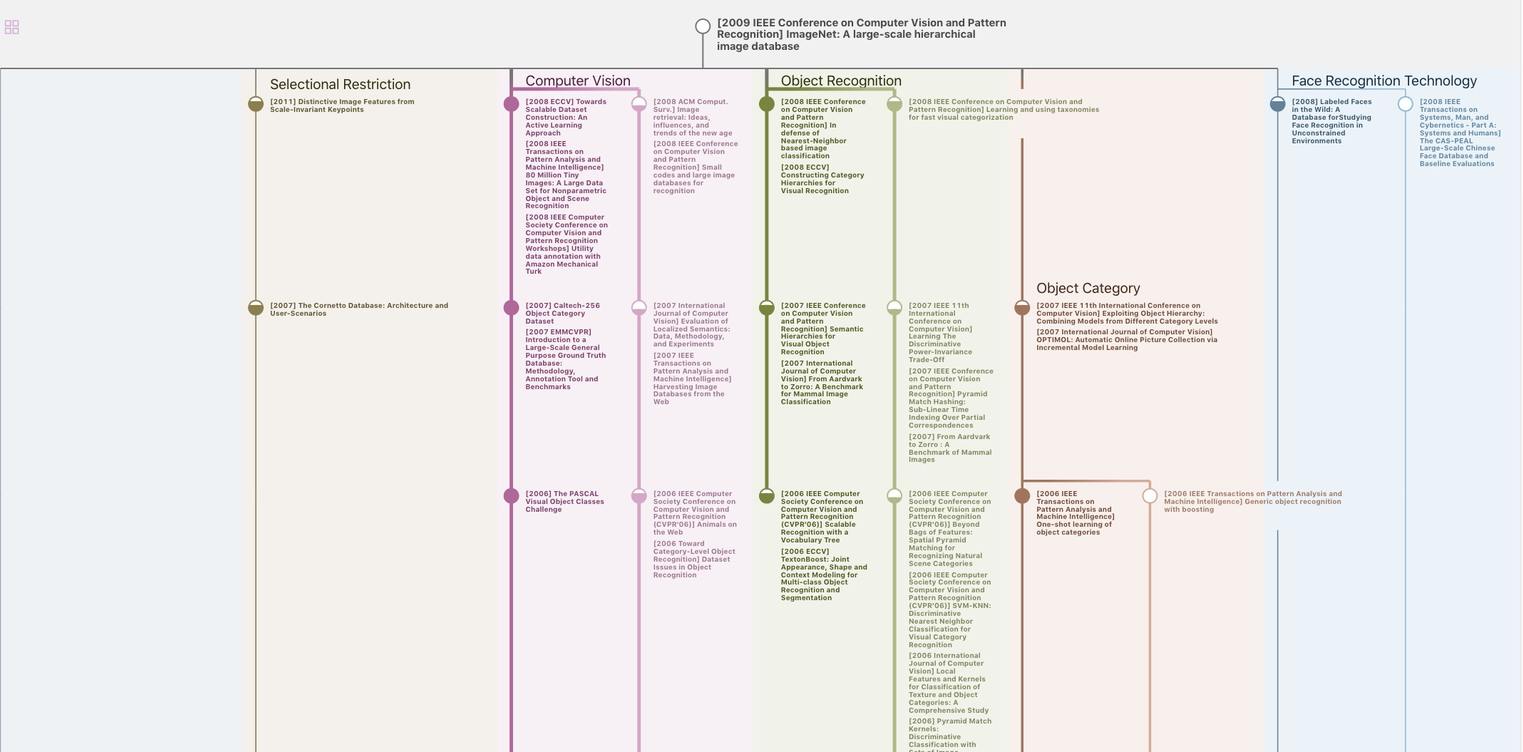
生成溯源树,研究论文发展脉络
Chat Paper
正在生成论文摘要