Analysis of ecosystem service drivers based on interpretive machine learning: a case study of Zhejiang Province, China
Environmental Science and Pollution Research(2022)
Abstract
A systematic understanding of the driving mechanisms of ecosystem services (ESs) and the relationships among them is critical for successful ecosystem management. However, the impact of driving factors on the relationships between ESs and the formation of ecosystem service bundles (ESBs) remains unclear. To address this gap, we developed a modeling process that used random forest (RF) to model the ESs and ESBs of Zhejiang Province, China, in regression and classification mode, respectively, and the Shapley Additive Explanations (SHAP) method to interpret the underlying driving forces. We first mapped the spatial distribution of seven ESs in Zhejiang Province at a 1 × 1 km spatial resolution and then used the K-means clustering algorithm to obtain four ESBs. Combining the RF models with SHAP analysis, the results showed that each ES had key driving factors, and the relationships of synergy and trade-off between ESs were determined by the driving direction and intensity of the key factors. The driving factors affect the relationships of ESs and consequently affect the formation of ESBs. Thus, managing the dominant drivers is key to improving the supply capacity of ESs.
MoreTranslated text
Key words
Ecosystem service, Ecosystem service bundles, Random forest, Driving force analysis, Shapley additive explanations
AI Read Science
Must-Reading Tree
Example
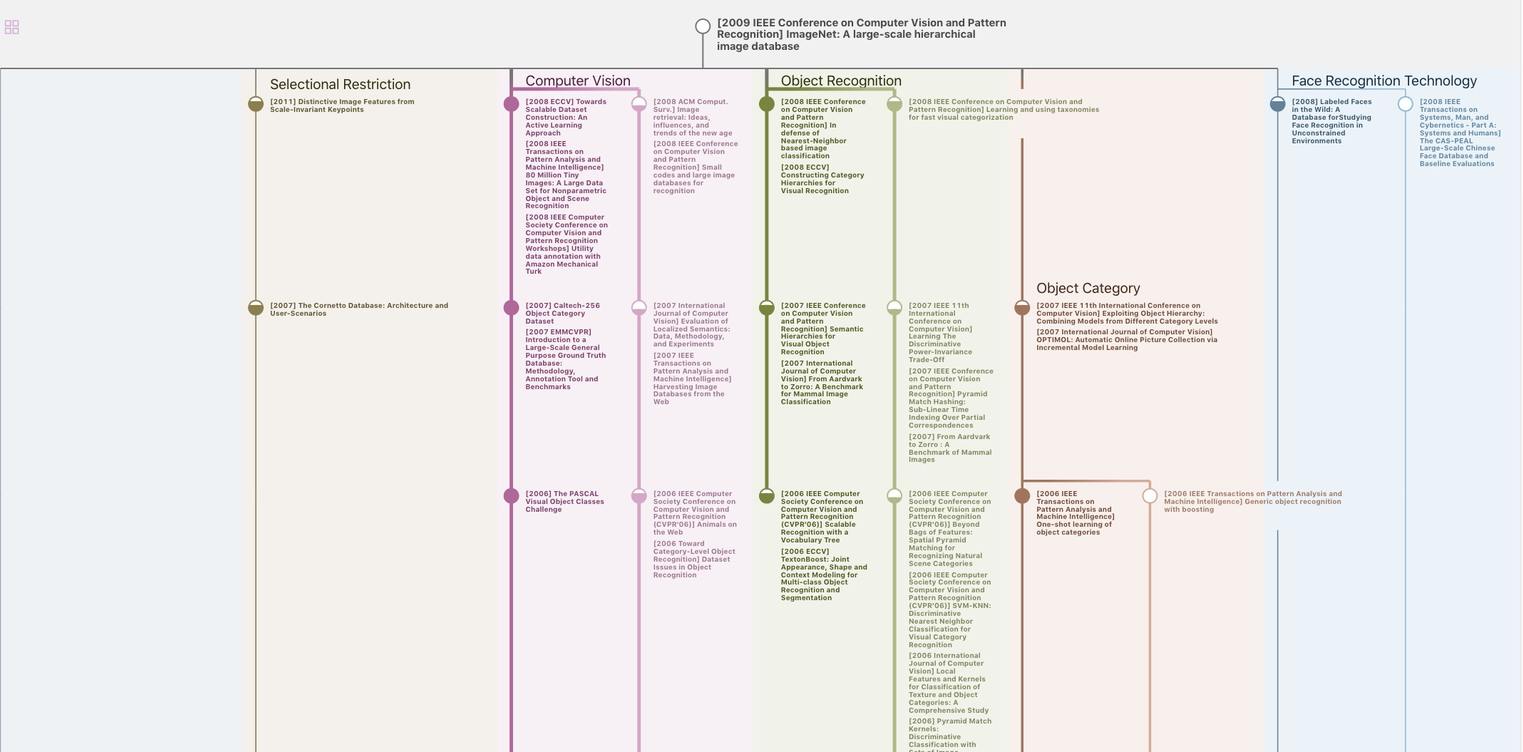
Generate MRT to find the research sequence of this paper
Chat Paper
Summary is being generated by the instructions you defined