When do Contrastive Word Alignments Improve Many-to-many Neural Machine Translation?
The Annual Conference of the North American Chapter of the Association for Computational Linguistics(2022)
摘要
Word alignment has proven to benefit many-to-many neural machine translation (NMT). However, high-quality ground-truth bilingual dictionaries were used for pre-editing in previous methods, which are unavailable for most language pairs. Meanwhile, the contrastive objective can implicitly utilize automatically learned word alignment, which has not been explored in many-to-many NMT. This work proposes a word-level contrastive objective to leverage word alignments for many-to-many NMT. Empirical results show that this leads to 0.8 BLEU gains for several language pairs. Analyses reveal that in many-to-many NMT, the encoder's sentence retrieval performance highly correlates with the translation quality, which explains when the proposed method impacts translation. This motivates future exploration for many-to-many NMT to improve the encoder's sentence retrieval performance.
更多查看译文
关键词
contrastive word alignments,machine
AI 理解论文
溯源树
样例
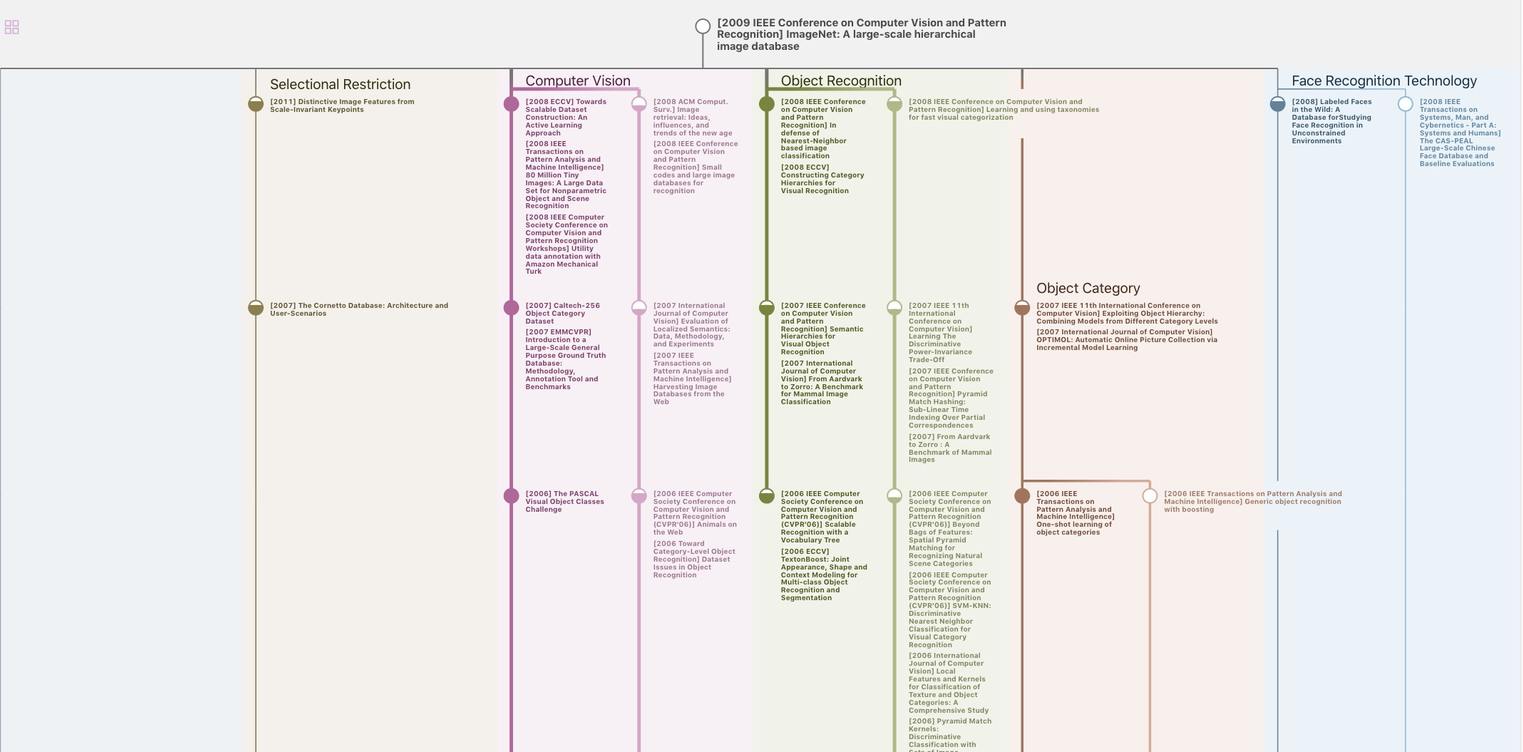
生成溯源树,研究论文发展脉络
Chat Paper
正在生成论文摘要