On-Device Next-Item Recommendation with Self-Supervised Knowledge Distillation
SIGIR '22: Proceedings of the 45th International ACM SIGIR Conference on Research and Development in Information Retrieval(2022)
摘要
Session-based recommender systems (SBR) are becoming increasingly popular because they can predict user interests without relying on long-term user profile and support login-free recommendation. Modern recommender systems operate in a fully server-based fashion. To cater to millions of users, the frequent model maintaining and the high-speed processing for concurrent user requests are required, which comes at the cost of a huge carbon footprint. Meanwhile, users need to upload their behavior data even including the immediate environmental context to the server, raising the public concern about privacy. On-device recommender systems circumvent these two issues with cost-conscious settings and local inference. However, due to the limited memory and computing resources, on-device recommender systems are confronted with two fundamental challenges: (1) how to reduce the size of regular models to fit edge devices? (2) how to retain the original capacity? Previous research mostly adopts tensor decomposition techniques to compress regular recommendation models with low compression rates so as to avoid drastic performance degradation. In this paper, we explore ultra-compact models for next-item recommendation, by loosing the constraint of dimensionality consistency in tensor decomposition. To compensate for the capacity loss caused by compression, we develop a self-supervised knowledge distillation framework which enables the compressed model (student) to distill the essential information lying in the raw data, and improves the long-tail item recommendation through an embedding-recombination strategy with the original model (teacher). The extensive experiments on two benchmarks demonstrate that, with 30x size reduction, the compressed model almost comes with no accuracy loss, and even outperforms its uncompressed counterpart. The code is released at https://github.com/xiaxin1998/OD-Rec.
更多查看译文
关键词
Next-item Recommendation, Self-supervised Learning, Knowledge Distillation, On-device Learning
AI 理解论文
溯源树
样例
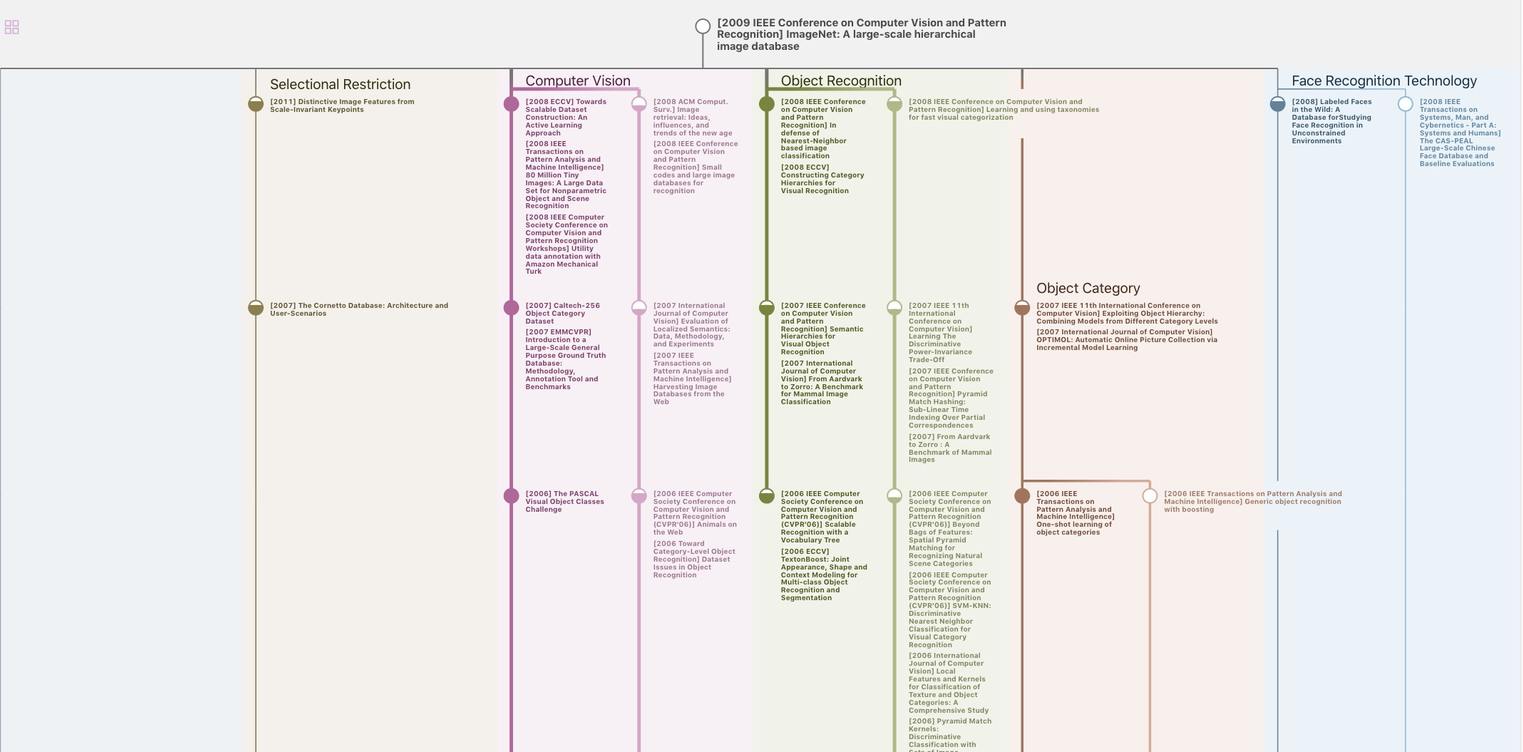
生成溯源树,研究论文发展脉络
Chat Paper
正在生成论文摘要