Dimension Free Nonasymptotic Bounds on the Accuracy of High-Dimensional Laplace Approximation
arxiv(2023)
摘要
This paper aims at revisiting the classical results on Laplace approximation in a modern nonasymptotic and dimension-free form. Such an extension is motivated by applications to high-dimensional statistical and optimization problems. The established results provide explicit nonasymptotic bounds on the quality of a Gaussian approximation of the posterior distribution in total variation distance in terms of the so-called effective dimension p(G). This value is defined as the interplay between the information contained in the data and in the prior distribution. In contrast to prominent Bernstein-von Mises results, the impact of the prior is not negligible, and it allows one to keep the effective dimension small or moderate even if the true parameter dimension is huge. We also address the issue of using a Gaussian approximation with inexact parameters, with the focus on replacing the maximum a posteriori (MAP) value by the posterior mean and designing the algorithm of Bayesian optimization based on Laplace iterations. The results are specified to the case of nonlinear regression.
更多查看译文
关键词
dimension free nonasymptotic bounds,high-dimensional
AI 理解论文
溯源树
样例
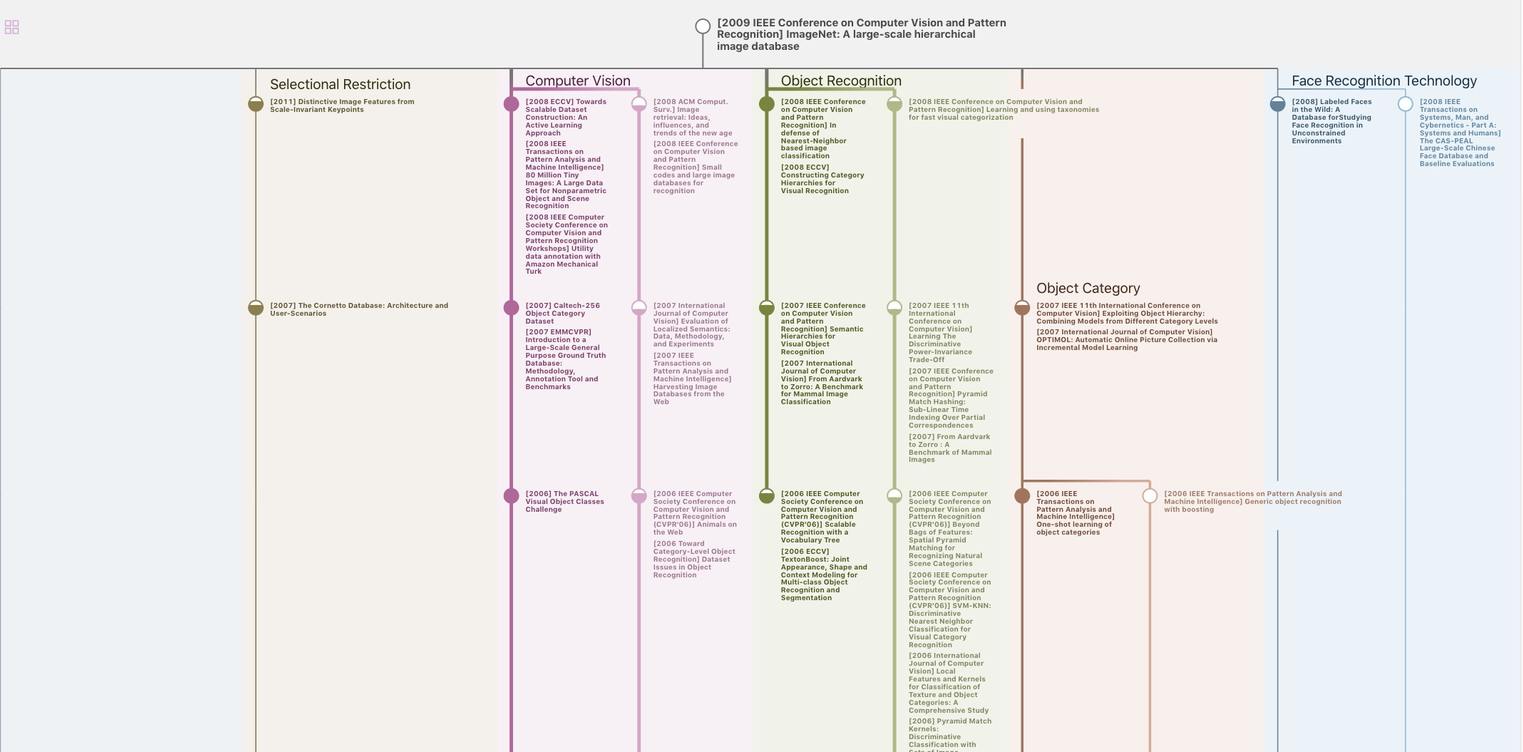
生成溯源树,研究论文发展脉络
Chat Paper
正在生成论文摘要