Identifying Chinese Opinion Expressions with Extremely-Noisy Crowdsourcing Annotations
PROCEEDINGS OF THE 60TH ANNUAL MEETING OF THE ASSOCIATION FOR COMPUTATIONAL LINGUISTICS (ACL 2022), VOL 1: (LONG PAPERS)(2022)
摘要
Recent works of opinion expression identification (OEI) rely heavily on the quality and scale of the manually-constructed training corpus, which could be extremely difficult to satisfy. Crowdsourcing is one practical solution for this problem, aiming to create a large-scale but quality-unguaranteed corpus. In this work, we investigate Chinese OEI with extremely-noisy crowdsourcing annotations, constructing a dataset at a very low cost. Following Zhang et al. (2021), we train the annotator-adapter model by regarding all annotations as gold-standard in terms of crowd annotators, and test the model by using a synthetic expert, which is a mixture of all annotators. As this annotator-mixture for testing is never modeled explicitly in the training phase, we propose to generate synthetic training samples by a pertinent mixup strategy to make the training and testing highly consistent. The simulation experiments on our constructed dataset show that crowdsourcing is highly promising for OEI, and our proposed annotator-mixup can further enhance the crowd-sourcing modeling.
更多查看译文
关键词
chinese opinion expressions,extremely-noisy
AI 理解论文
溯源树
样例
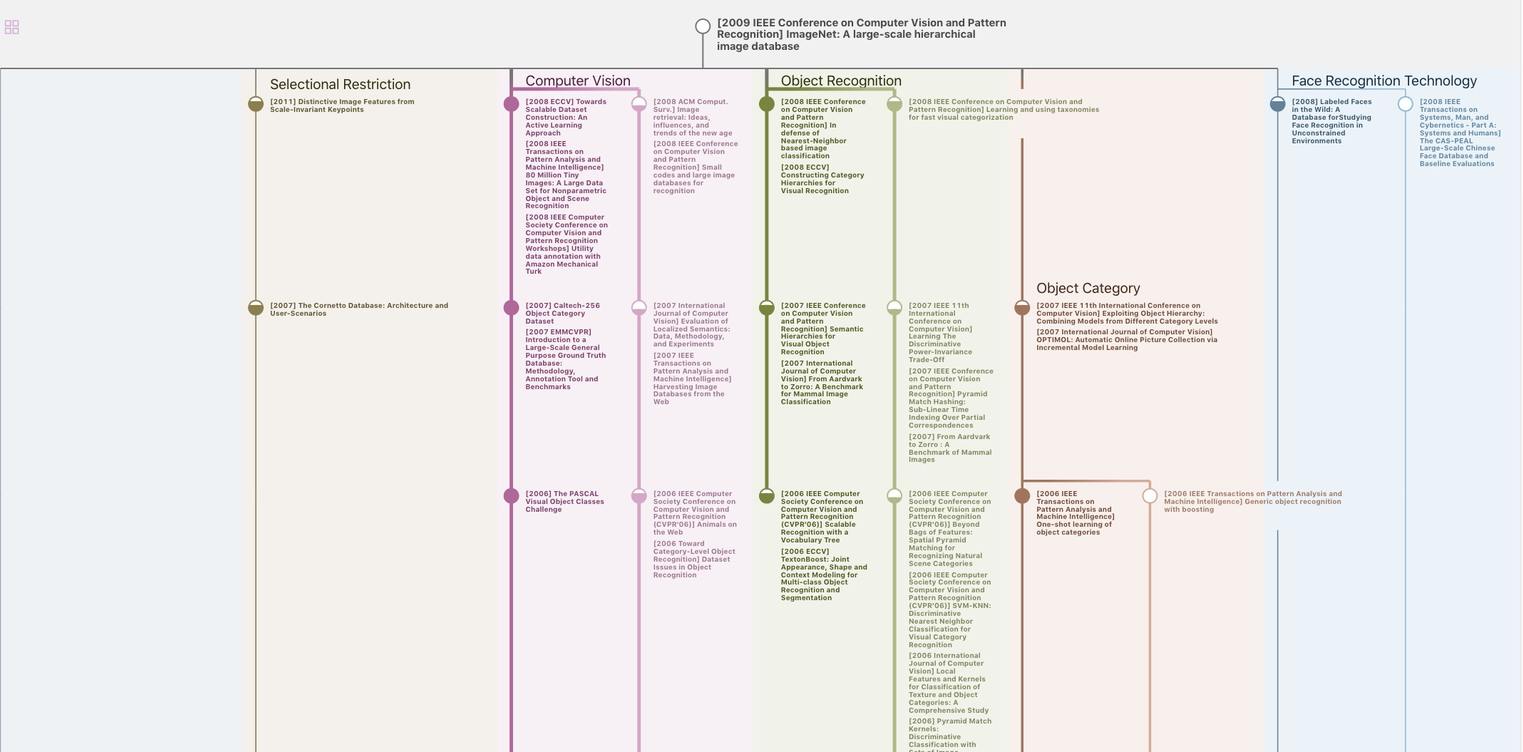
生成溯源树,研究论文发展脉络
Chat Paper
正在生成论文摘要