EVOTER: Evolution of Transparent Explainable Rule-sets
CoRR(2022)
摘要
Most AI systems are black boxes generating reasonable outputs for given inputs. Some domains, however, have explainability and trustworthiness requirements that cannot be directly met by these approaches. Various methods have therefore been developed to interpret black-box models after training. This paper advocates an alternative approach where the models are transparent and explainable to begin with. This approach, EVOTER, evolves rule-sets based on simple logical expressions. The approach is evaluated in several prediction/classification and prescription/policy search domains with and without a surrogate. It is shown to discover meaningful rule sets that perform similarly to black-box models. The rules can provide insight into the domain, and make biases hidden in the data explicit. It may also be possible to edit them directly to remove biases and add constraints. EVOTER thus forms a promising foundation for building trustworthy AI systems for real-world applications in the future.
更多查看译文
关键词
evolution,transparent,evoter,rule-sets
AI 理解论文
溯源树
样例
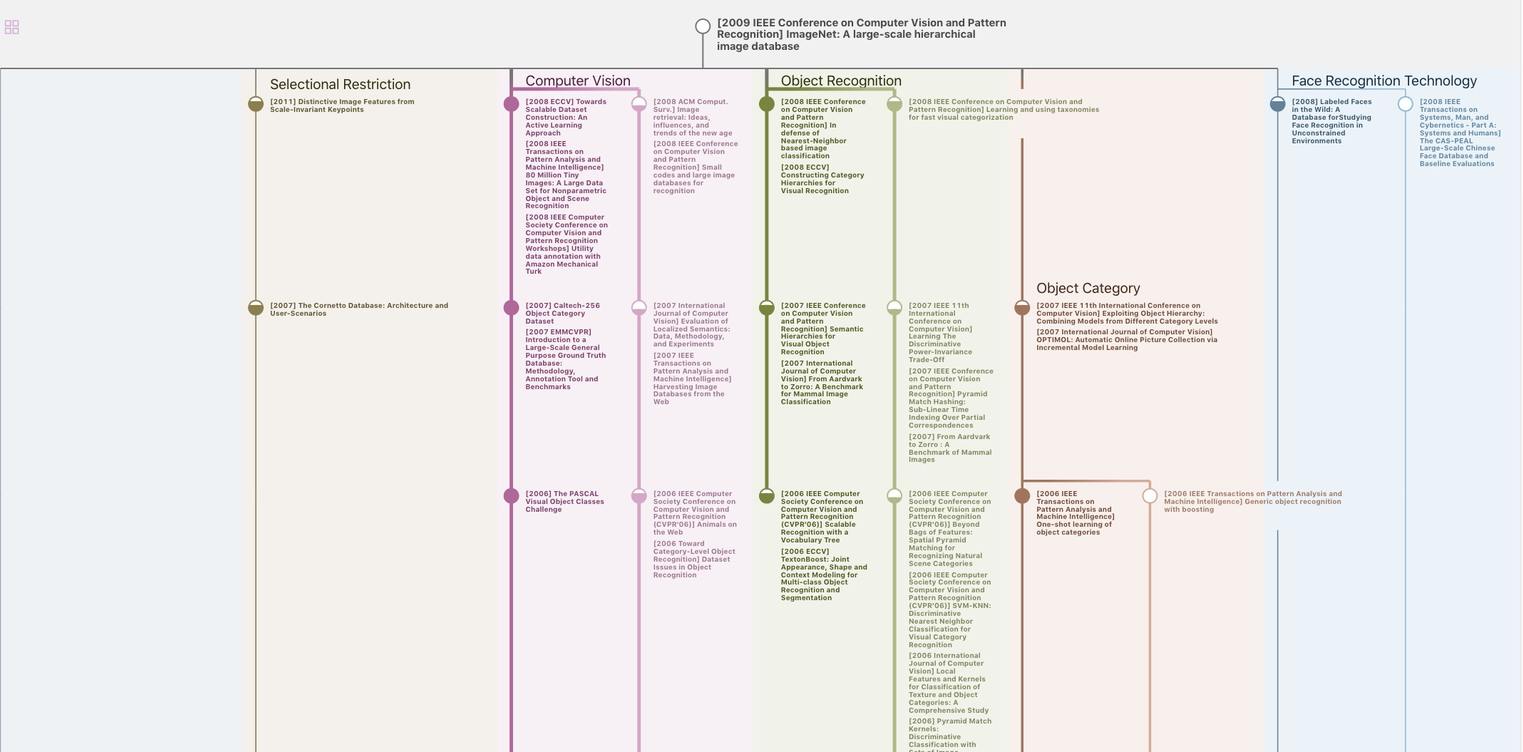
生成溯源树,研究论文发展脉络
Chat Paper
正在生成论文摘要