Fast environmental sound classification based on resource adaptive convolutional neural network
SCIENTIFIC REPORTS(2022)
Abstract
Recently, with the construction of smart city, the research on environmental sound classification (ESC) has attracted the attention of academia and industry. The development of convolutional neural network (CNN) makes the accuracy of ESC reach a higher level, but the accuracy improvement brought by CNN is often accompanied by the deepening of network layers, which leads to the rapid growth of parameters and floating-point operations (FLOPs). Therefore, it is difficult to transplant CNN model to embedded devices, and the classification speed is also difficult to accept. In order to reduce the hardware requirements of running CNN and improve the speed of ESC, this paper proposes a resource adaptive convolutional neural network (RACNN). RACNN uses a novel resource adaptive convolutional (RAC) module, which can generate the same number of feature maps as conventional convolution operations more cheaply, and extract the time and frequency features of audio efficiently. The RAC block based on the RAC module is designed to build the lightweight RACNN model, and the RAC module can also be used to upgrade the existing CNN model. Experiments based on public datasets show that RACNN achieves higher performance than the state-of-the-art methods with lower computational complexity.
MoreTranslated text
Key words
Acoustics,Computer science,Science,Humanities and Social Sciences,multidisciplinary
AI Read Science
Must-Reading Tree
Example
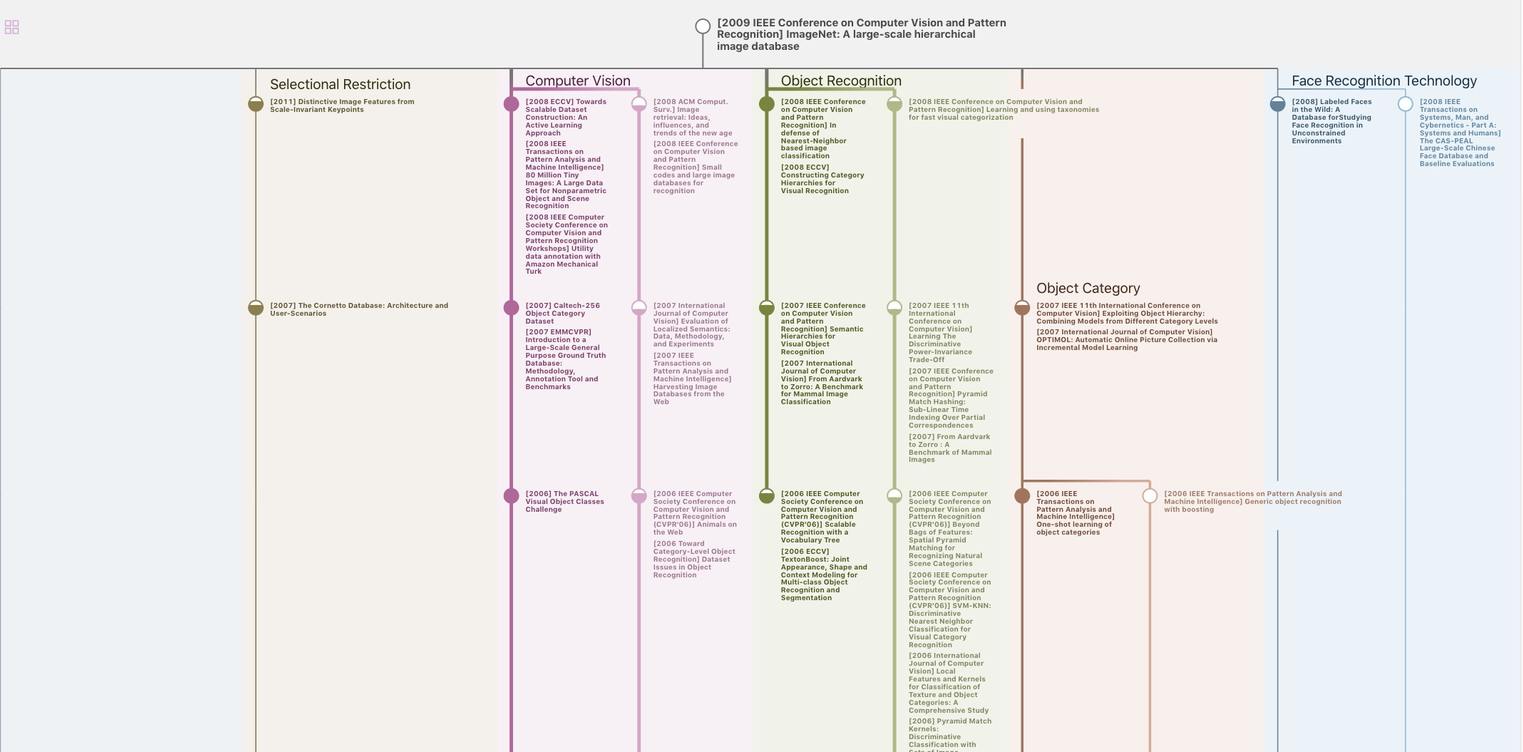
Generate MRT to find the research sequence of this paper
Chat Paper
Summary is being generated by the instructions you defined