Adversarial Multiscale Feature Learning Framework for Overlapping Chromosome Segmentation
Entropy (Basel, Switzerland)(2022)
摘要
Chromosome karyotype analysis is of great clinical importance in the diagnosis and treatment of diseases. Since manual analysis is highly time and effort consuming, computer-assisted automatic chromosome karyotype analysis based on images is routinely used to improve the efficiency and accuracy of the analysis. However, the strip-shaped chromosomes easily overlap each other when imaged, significantly affecting the accuracy of the subsequent analysis and hindering the development of chromosome analysis instruments. In this paper, we present an adversarial, multiscale feature learning framework to improve the accuracy and adaptability of overlapping chromosome segmentation. We first adopt the nested U-shaped network with dense skip connections as the generator to explore the optimal representation of the chromosome images by exploiting multiscale features. Then we use the conditional generative adversarial network (cGAN) to generate images similar to the original ones; the training stability of the network is enhanced by applying the least-square GAN objective. Finally, we replace the common cross-entropy loss with the advanced Lovász-Softmax loss to improve the model’s optimization and accelerate the model’s convergence. Comparing with the established algorithms, the performance of our framework is proven superior by using public datasets in eight evaluation criteria, showing its great potential in overlapping chromosome segmentation.
更多查看译文
关键词
overlapping chromosome segmentation,conditional generative adversarial network,nested U-shaped network,multiscale feature learning,Lovász-Softmax
AI 理解论文
溯源树
样例
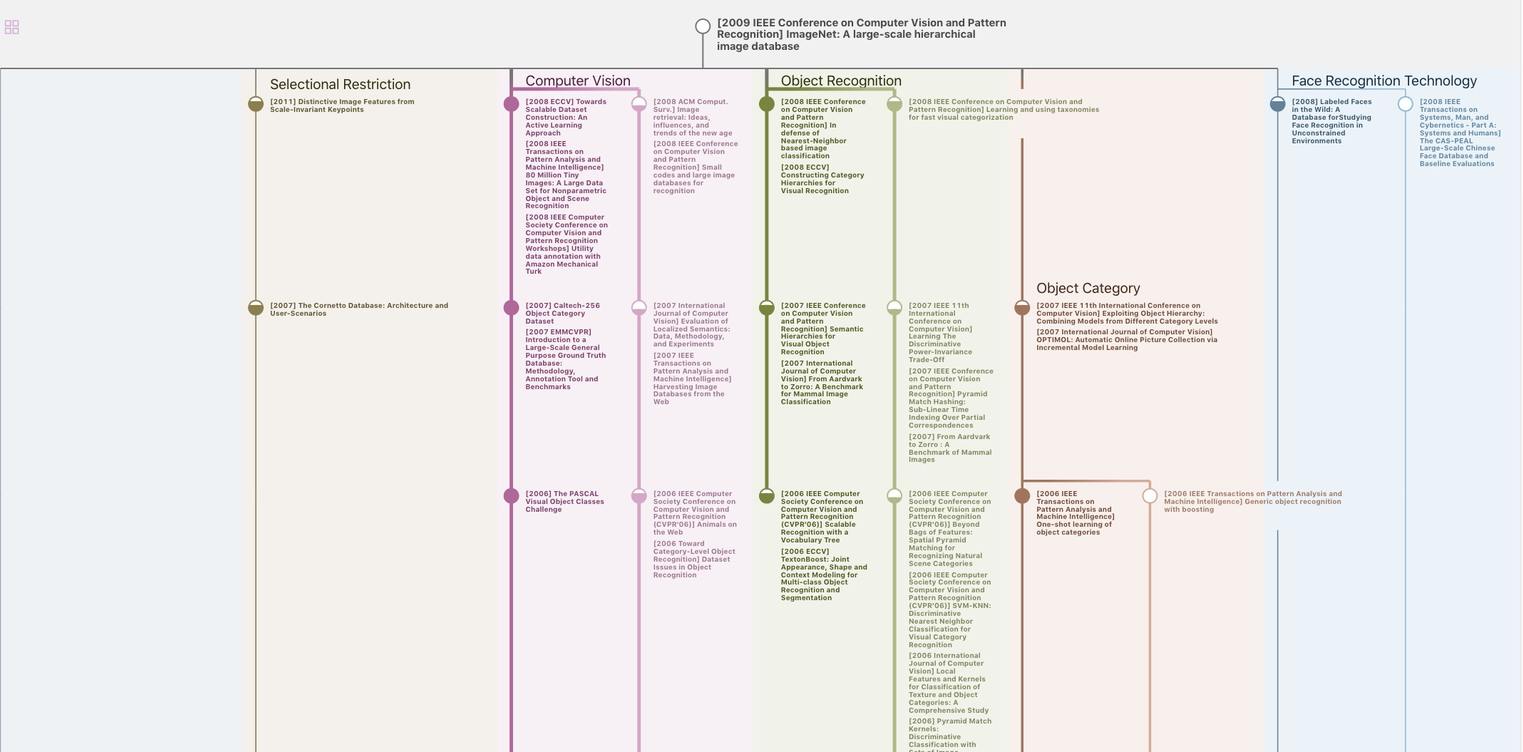
生成溯源树,研究论文发展脉络
Chat Paper
正在生成论文摘要