Transfer learning of clinical outcomes from preclinical molecular data, principles and perspectives
BRIEFINGS IN BIOINFORMATICS(2022)
摘要
Accurate transfer learning of clinical outcomes from one cellular context to another, between cell types, developmental stages, omics modalities or species, is considered tremendously useful. When transferring a prediction task from a source domain to a target domain, what counts is the high quality of the predictions in the target domain, requiring states or processes common to both the source and the target that can be learned by the predictor reflected by shared denominators. These may form a compendium of knowledge that is learned in the source to enable predictions in the target, usually with few, if any, labeled target training samples to learn from. Transductive transfer learning refers to the learning of the predictor in the source domain, transferring its outcome label calculations to the target domain, considering the same task. Inductive transfer learning considers cases where the target predictor is performing a different yet related task as compared with the source predictor. Often, there is also a need to first map the variables in the input/feature spaces and/or the variables in the output/outcome spaces. We here discuss and juxtapose various recently published transfer learning approaches, specifically designed (or at least adaptable) to predict clinical (human in vivo) outcomes based on preclinical (mostly animal-based) molecular data, towards finding the right tool for a given task, and paving the way for a comprehensive and systematic comparison of the suitability and accuracy of transfer learning of clinical outcomes.
更多查看译文
关键词
transfer learning, shared denominators, biomarkers, transductive transfer learning, inductive transfer learning, unsupervised transfer learning
AI 理解论文
溯源树
样例
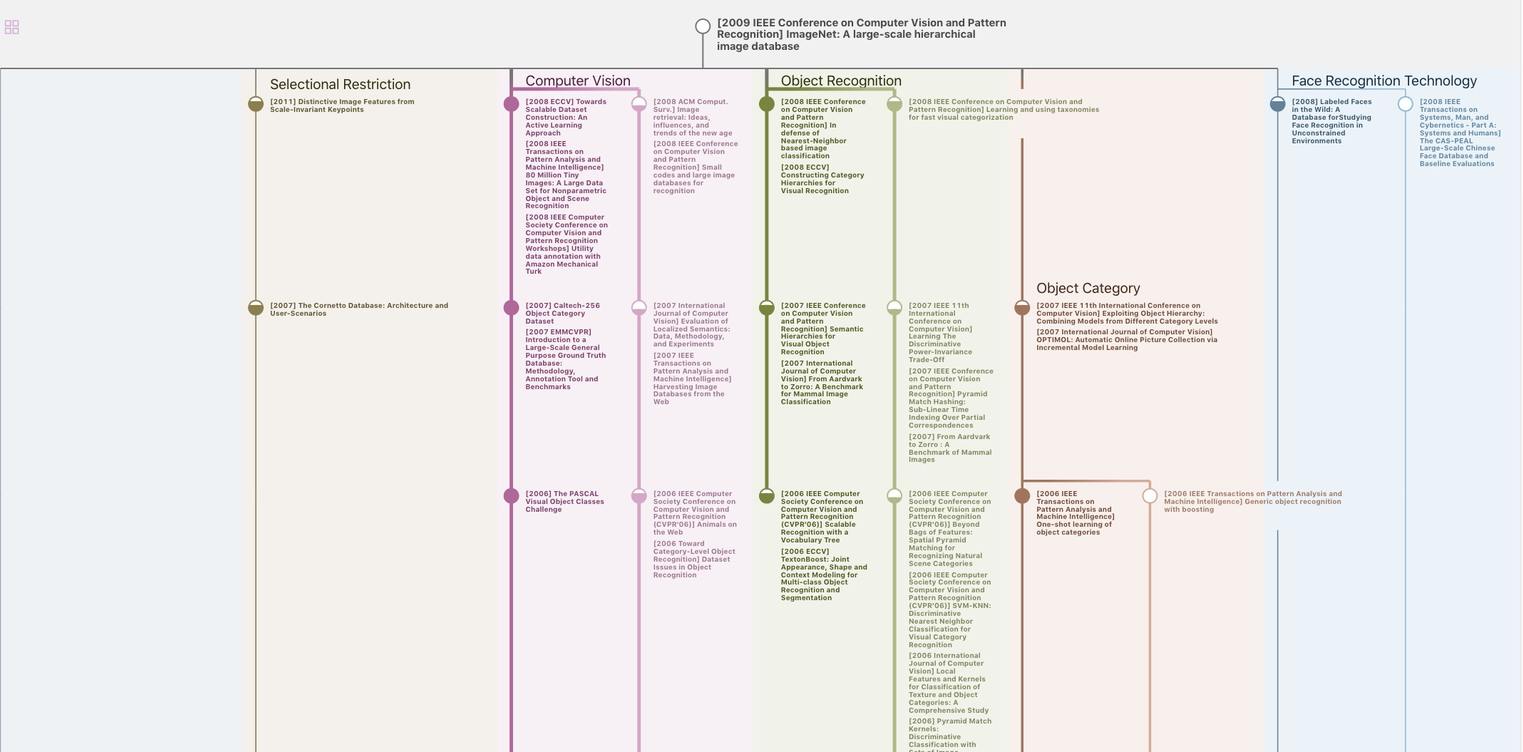
生成溯源树,研究论文发展脉络
Chat Paper
正在生成论文摘要