Discrete-Time Fractional-Order Dynamical Networks Minimum-Energy State Estimation
user-62626390733dcd7b976fc023(2021)
摘要
Fractional-order dynamical networks are increasingly being used to model and describe processes demonstrating long-term memory or complex interlaced dependencies among the spatial and temporal components of a wide variety of dynamical networks. Notable examples include networked control systems or neurophysiological networks which are created using electroencephalographic (EEG) or blood-oxygen-level-dependent data. As a result, the estimation of the states of fractional-order dynamical networks poses an important problem. To this effect, this article addresses the problem of minimum-energy state estimation for discrete-time fractional-order dynamical networks, where the state and output equations are affected by an additive noise that is considered to be deterministic, bounded, and unknown. Specifically, we derive the corresponding estimator and show that the resulting estimation error is exponentially input-to-state stable with respect to the disturbances and to a signal that is decreasing with the increase of the accuracy of the adopted approximation model. An illustrative example shows the effectiveness of the proposed method on real-world neurophysiological networks. Our results may significantly contribute to the development of novel neurotechnologies, particularly in the development of state estimation paradigms for neural signals such as EEG, which are often noisy signals known to be affected by artifacts not having any particular stochastic characterization.
更多查看译文
关键词
Biological networks,cyberphysical systems,decision or estimation theory,other applications
AI 理解论文
溯源树
样例
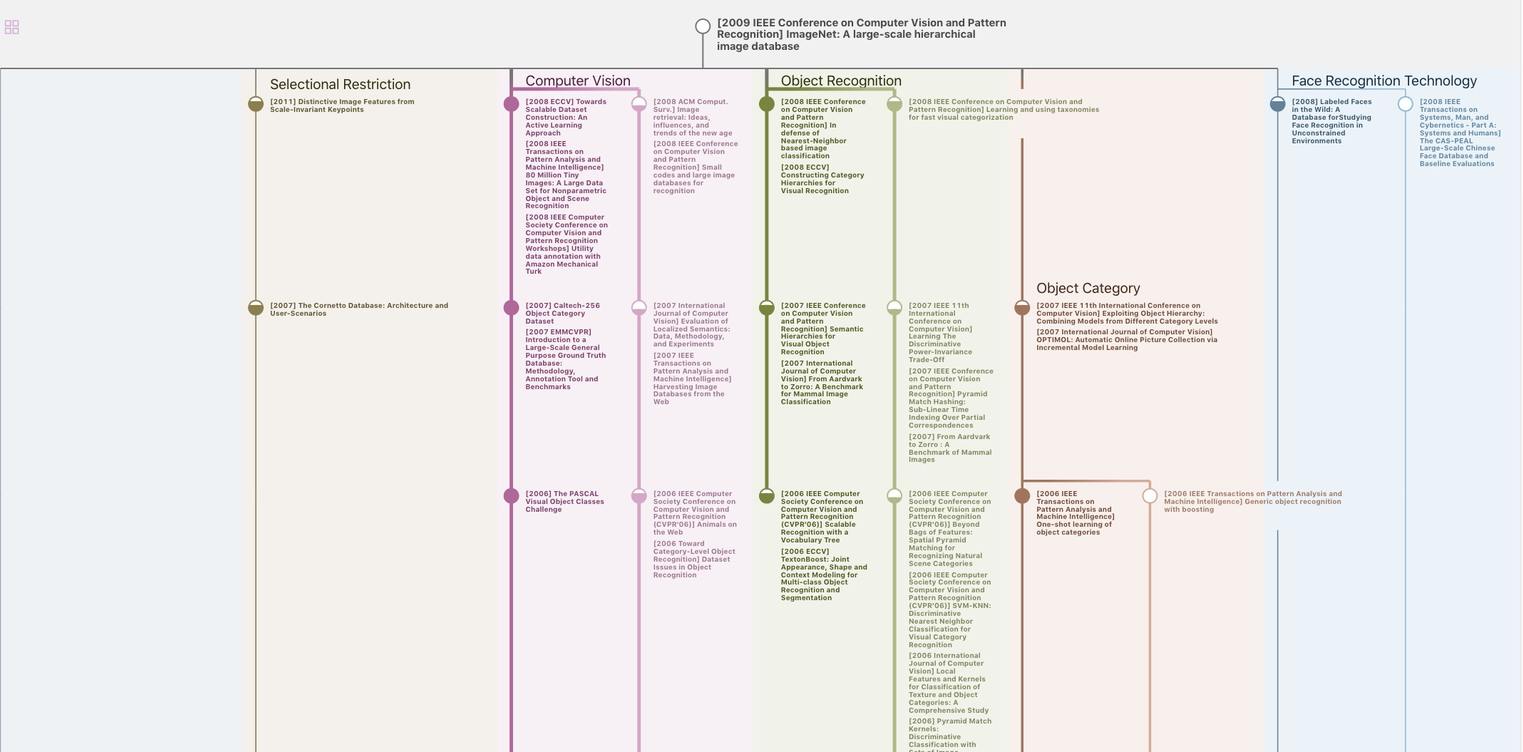
生成溯源树,研究论文发展脉络
Chat Paper
正在生成论文摘要