Deep transfer operator learning for partial differential equations under conditional shift
arxiv(2022)
摘要
Transfer learning enables the transfer of knowledge gained while learning to perform one task (source) to a related but different task (target), hence addressing the expense of data acquisition and labelling, potential computational power limitations and dataset distribution mismatches. We propose a new transfer learning framework for task-specific learning (functional regression in partial differential equations) under conditional shift based on the deep operator network (DeepONet). Task-specific operator learning is accomplished by fine-tuning task-specific layers of the target DeepONet using a hybrid loss function that allows for the matching of individual target samples while also preserving the global properties of the conditional distribution of the target data. Inspired by conditional embedding operator theory, we minimize the statistical distance between labelled target data and the surrogate prediction on unlabelled target data by embedding conditional distributions onto a reproducing kernel Hilbert space. We demonstrate the advantages of our approach for various transfer learning scenarios involving nonlinear partial differential equations under diverse conditions due to shifts in the geometric domain and model dynamics. Our transfer learning framework enables fast and efficient learning of heterogeneous tasks despite considerable differences between the source and target domains.
更多查看译文
关键词
Applied mathematics,Computational science,Engineering,general
AI 理解论文
溯源树
样例
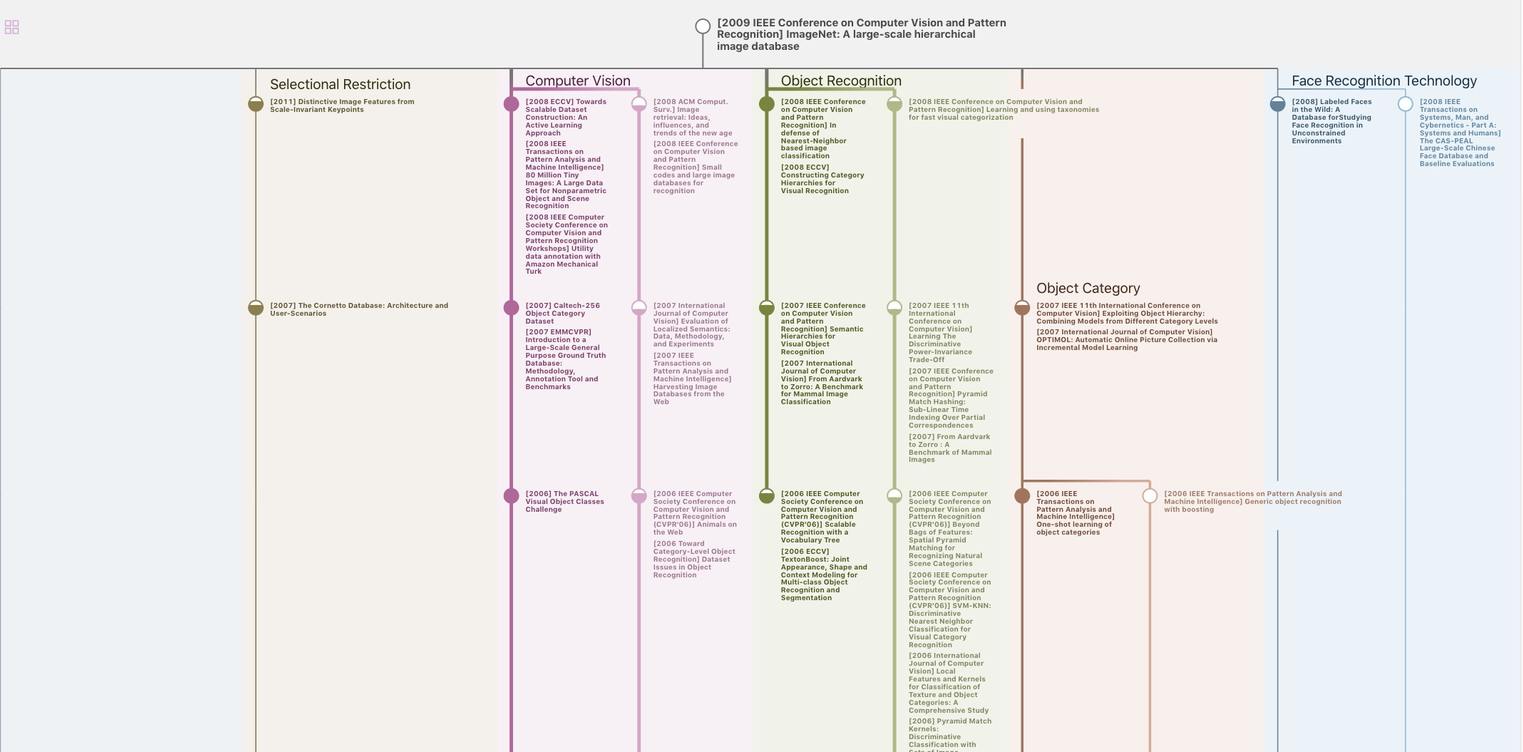
生成溯源树,研究论文发展脉络
Chat Paper
正在生成论文摘要