A Category-aware Multi-interest Model for Personalized Product Search
International World Wide Web Conference(2022)
Abstract
Product search has been an important way for people to find products on online shopping platforms. Existing approaches in personalized product search mainly embed user preferences into one single vector. However, this simple strategy easily results in sub-optimal representations, failing to model and disentangle user's multiple preferences. To overcome this problem, we proposed a category-aware multi-interest model to encode users as multiple preference embeddings to represent user-specific interests. Specifically, we also capture the category indications for each preference to indicate the distribution of categories it focuses on, which is derived from rich relations between users, products, and attributes. Based on these category indications, we develop a category attention mechanism to aggregate these various preference embeddings considering current queries and items as the user's comprehensive representation. By this means, we can use this representation to calculate matching scores of retrieved items to determine whether they meet the user's search intent. Besides, we introduce a homogenization regularization term to avoid the redundancy between user interests. Experimental results show that the proposed method significantly outperforms existing approaches.
MoreTranslated text
Key words
personalized product search,multi-interest,knowledge graph
AI Read Science
Must-Reading Tree
Example
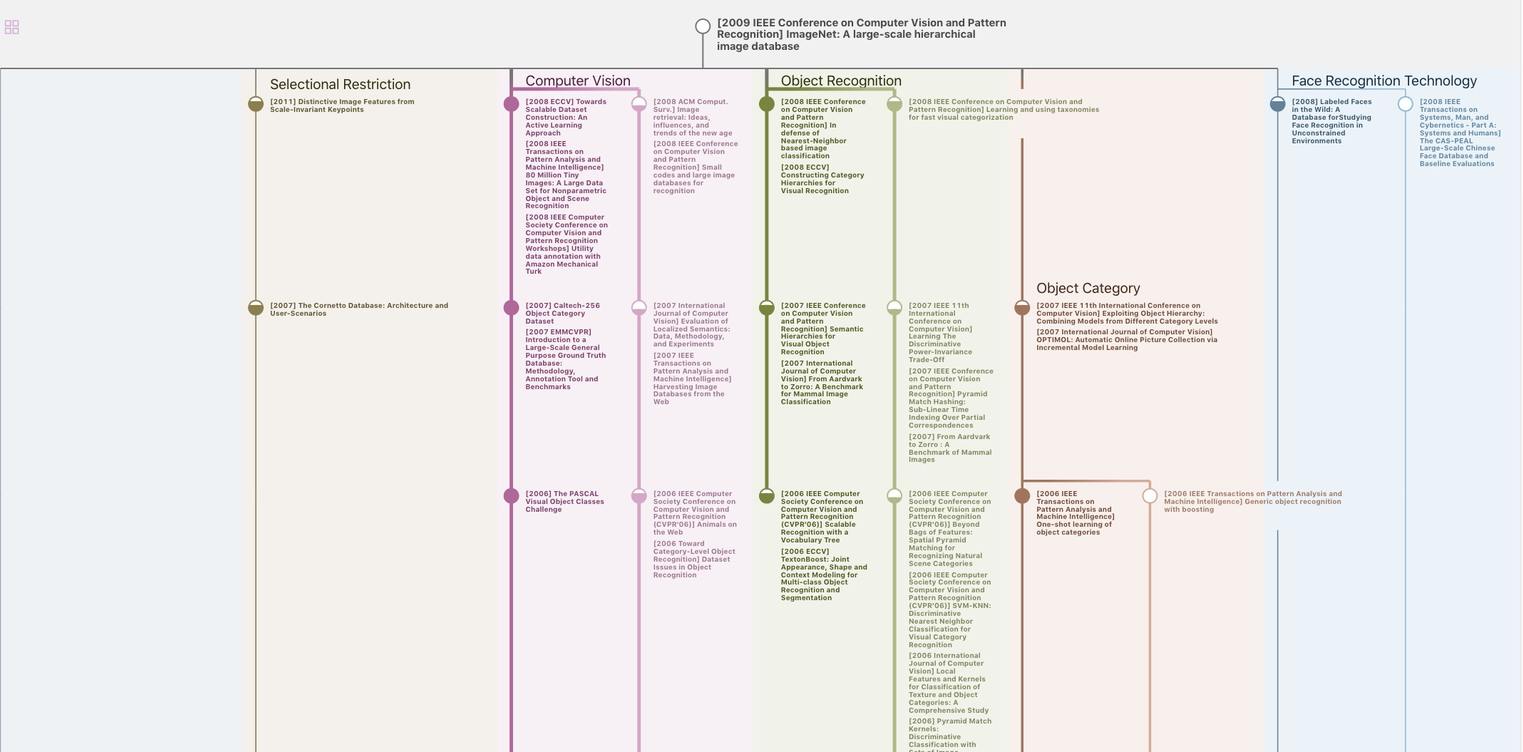
Generate MRT to find the research sequence of this paper
Chat Paper
Summary is being generated by the instructions you defined