Improving generalization of machine learning-identified biomarkers with causal modeling: an investigation into immune receptor diagnostics
arxiv(2023)
摘要
Machine learning is increasingly used to discover diagnostic and prognostic biomarkers from high-dimensional molecular data. However, a variety of factors related to experimental design may affect the ability to learn generalizable and clinically applicable diagnostics. Here, we argue that a causal perspective improves the identification of these challenges and formalizes their relation to the robustness and generalization of machine learning-based diagnostics. To make for a concrete discussion, we focus on a specific, recently established high-dimensional biomarker - adaptive immune receptor repertoires (AIRRs). Through simulations, we illustrate how major biological and experimental factors of the AIRR domain may influence the learned biomarkers. In conclusion, we argue that causal modeling improves machine learning-based biomarker robustness by identifying stable relations between variables and by guiding the adjustment of the relations and variables that vary between populations.
更多查看译文
关键词
biomarkers,causal modeling,immune,generalization,learning-identified
AI 理解论文
溯源树
样例
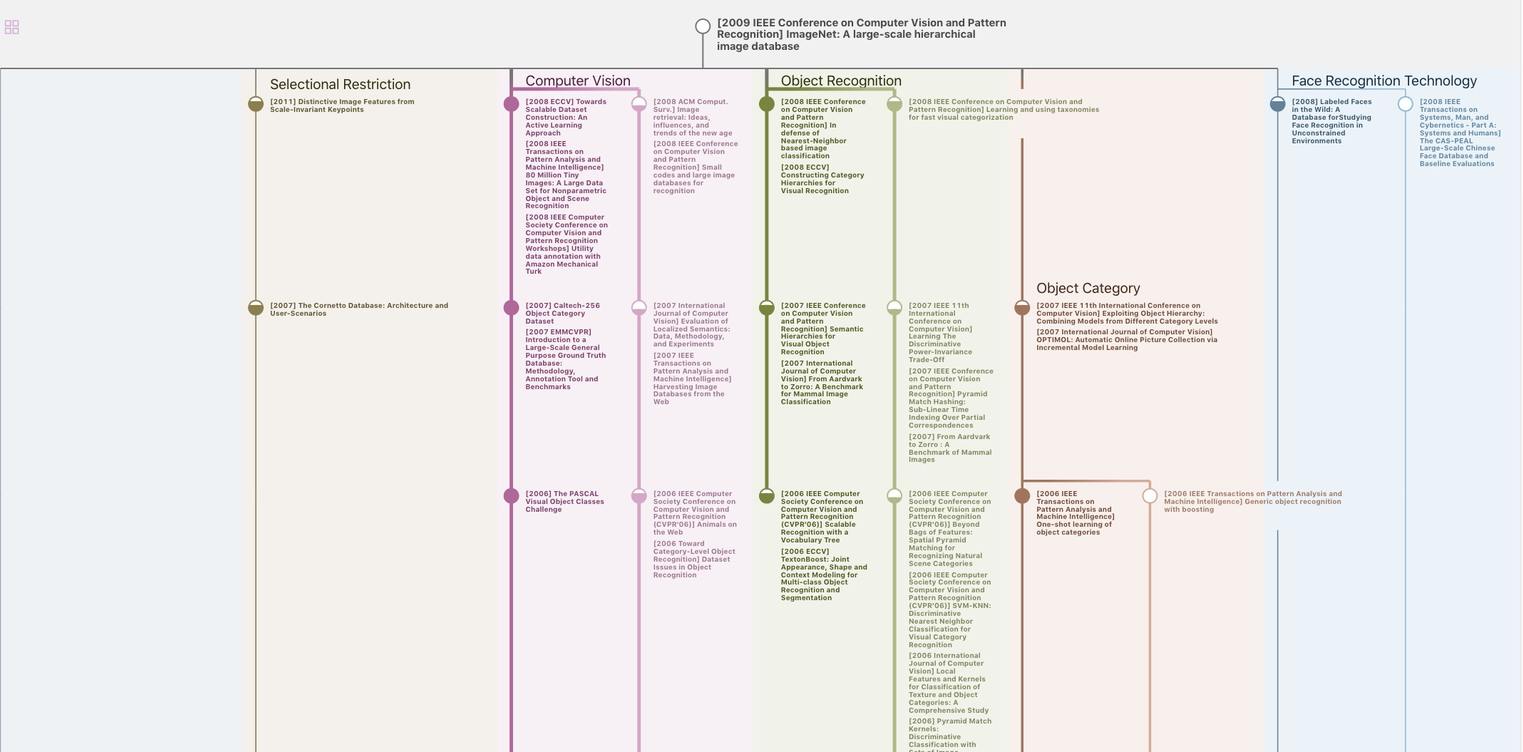
生成溯源树,研究论文发展脉络
Chat Paper
正在生成论文摘要