A predictive controller for real-time energy management of plug-in hybrid electric vehicles
Energy(2022)
摘要
Battery aging can degrade the energy efficiency of plug-in hybrid electric vehicles (PHEVs) significantly. This paper presents a novel intelligent real-time energy management strategy (EMS) for PHEVs, integrating battery life and fuel consumption optimization. The two-objective offline optimization problem is solved by the dynamic programming (DP) approach to obtain the globally optimal solutions. For the real-time implementation, a model predictive control (MPC) scheme is combined with an adaptive neuro-fuzzy inference system (ANFIS) model. DP carries out a receding-horizon optimization using future traffic information. Level-set functions are exploited within the DP algorithm to reduce numerical errors and decrease the computational effort of the baseline DP approach. Contrary to the real-time EMSs with a pre-determined state-of-charge (SOC) reference, an ANFIS model provides the SOC reference online. The proposed method is evaluated in simulation over multiple real-time driving cycles and compared with the DP results and two other real-time approaches. The effect of prediction horizon length is also studied. The simulation results demonstrate that the developed method can optimize battery life and fuel consumption. The results indicate 93%–97% matching to those of optimal controller, that is much better compared to the two other tested approaches.
更多查看译文
关键词
Battery life,Dynamic programming,Energy management,Level-set functions,Plug-in HEVs,Predictive control,Receding-horizon optimization
AI 理解论文
溯源树
样例
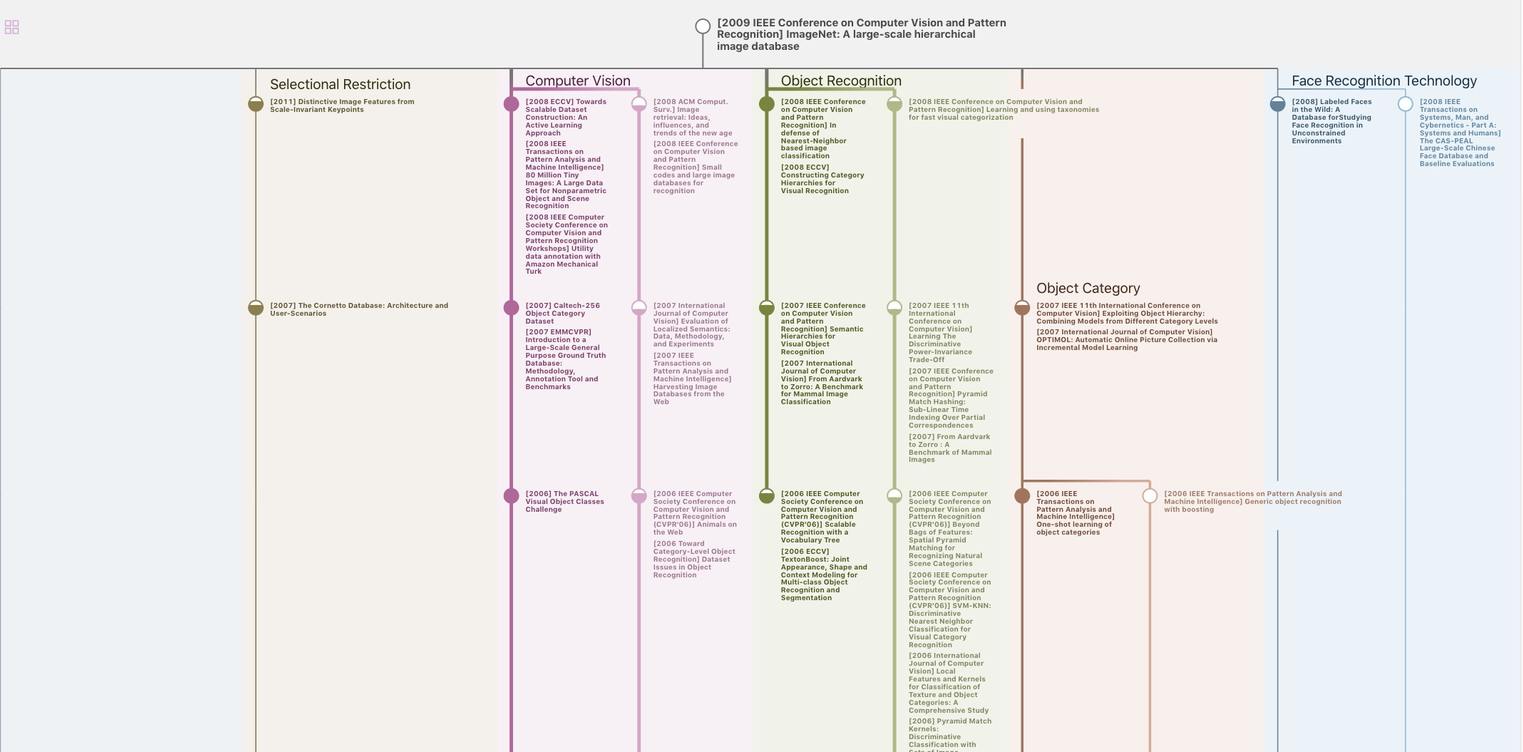
生成溯源树,研究论文发展脉络
Chat Paper
正在生成论文摘要