AutoMLBench: A comprehensive experimental evaluation of automated machine learning frameworks
EXPERT SYSTEMS WITH APPLICATIONS(2024)
摘要
With the booming demand for machine learning applications, it has been recognized that the number of knowledgeable data scientists cannot scale with the growing data volumes and application needs in our digital world. In response to this demand, several automated machine learning (AutoML) frameworks have been developed to fill the gap of human expertise by automating the process of building machine learning pipelines. Each framework comes with different heuristics-based design decisions. In this study, we present a comprehensive evaluation and comparison of the performance characteristics of six popular AutoML frameworks, namely, AutoWeka, AutoSKlearn, TPOT, Recipe, ATM and SmartML across 100 data sets from established AutoML benchmark suites. Our experimental evaluation considers different aspects for its comparison, including the performance impact of several design decisions, including time budget, size of search space, meta-learning, and ensemble construction. The results of our study reveal various interesting insights that can significantly guide and impact the design of AutoML frameworks.
更多查看译文
关键词
Automated machine learning,Meta-learning,Search space,Ensemble,Time budget
AI 理解论文
溯源树
样例
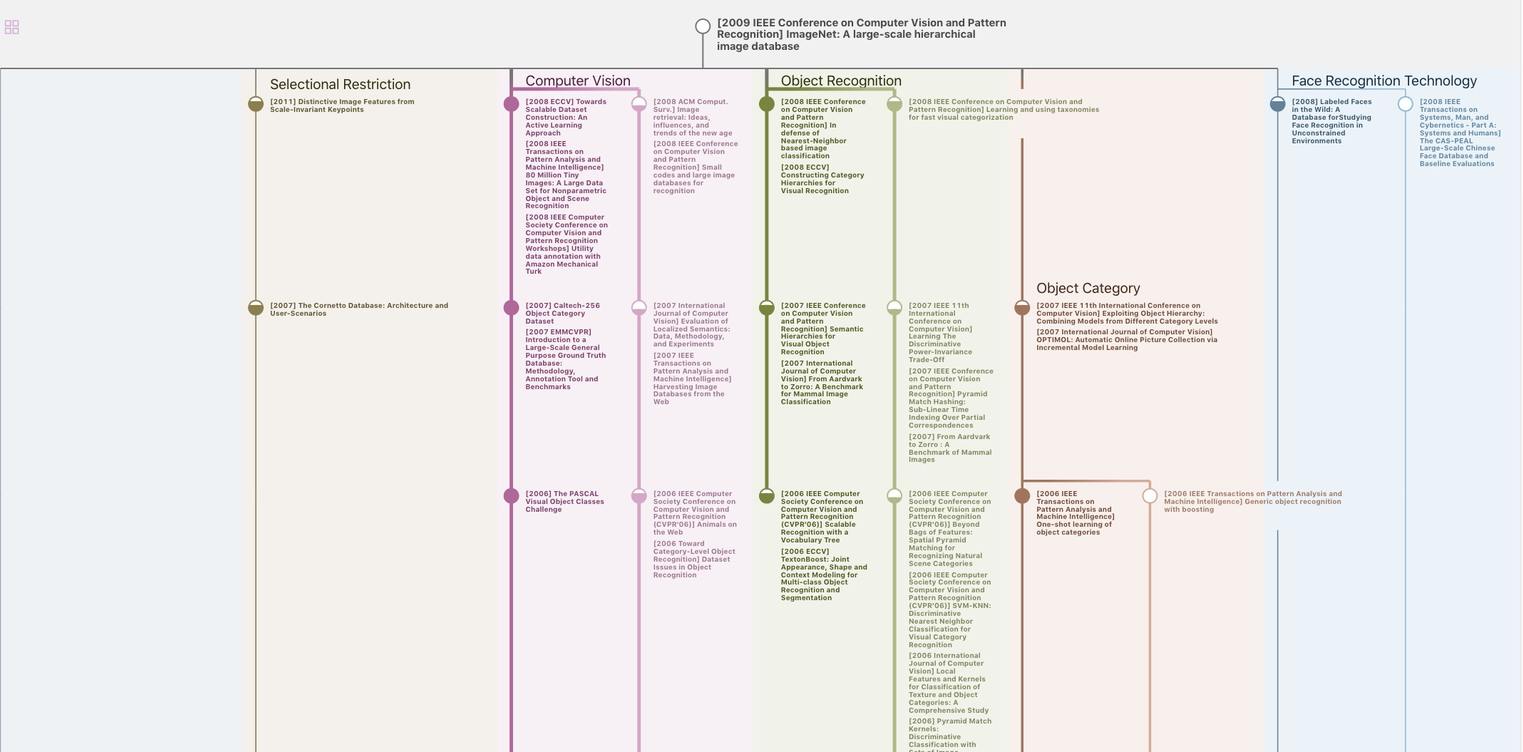
生成溯源树,研究论文发展脉络
Chat Paper
正在生成论文摘要