Exploiting Multiple EEG Data Domains with Adversarial Learning.
Annual International Conference of the IEEE Engineering in Medicine and Biology Society (EMBC)(2022)
摘要
Electroencephalography (EEG) is shown to be a valuable data source for evaluating subjects' mental states. However, the interpretation of multi-modal EEG signals is challenging, as they suffer from poor signal-to-noise-ratio, are highly subject-dependent, and are bound to the equipment and experimental setup used, (i.e. domain). This leads to machine learning models often suffer from poor generalization ability, where they perform significantly worse on real-world data than on the exploited training data. Recent research heavily focuses on cross-subject and cross-session transfer learning frameworks to reduce domain calibration efforts for EEG signals. We argue that multi-source learning via learning domain-invariant representations from multiple data-sources is a viable alternative, as the available data from different EEG data-source domains (e.g., subjects, sessions, experimental setups) grow massively. We propose an adversarial inference approach to learn data-source invariant representations in this context, enabling multi-source learning for EEG-based brain- computer interfaces. We unify EEG recordings from different source domains (i.e., emotion recognition datasets SEED, SEED-IV, DEAP, DREAMER), and demonstrate the feasibility of our invariant representation learning approach in suppressing data- source-relevant information leakage by 35% while still achieving stable EEG-based emotion classification performance.
更多查看译文
关键词
multiple eeg data domains,learning
AI 理解论文
溯源树
样例
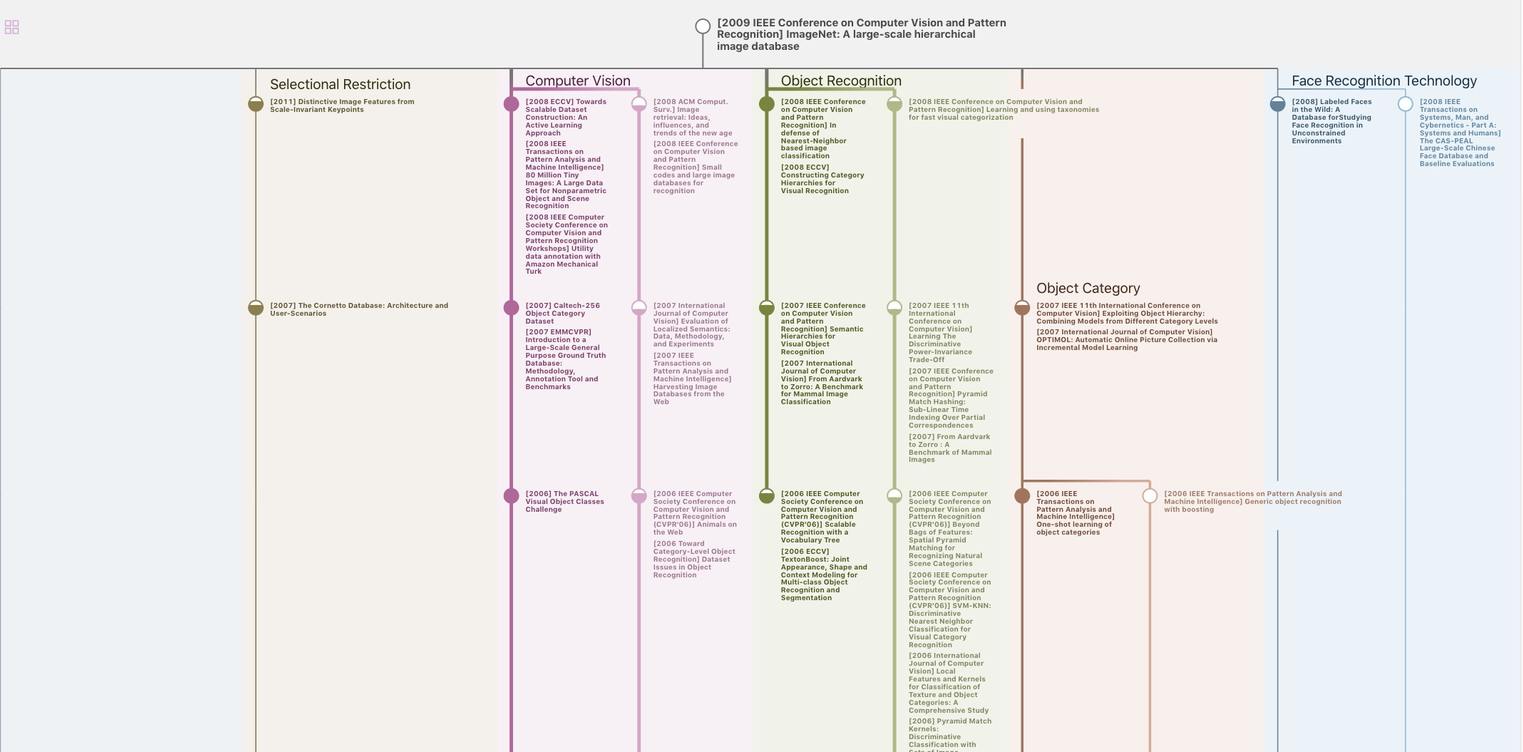
生成溯源树,研究论文发展脉络
Chat Paper
正在生成论文摘要