UFRC: A Unified Framework for Reliable COVID-19 Detection on Crowdsourced Cough Audio
2022 44th Annual International Conference of the IEEE Engineering in Medicine & Biology Society (EMBC)(2022)
关键词
COVID-19,Cough,Crowdsourcing,Humans,Reproducibility of Results,Uncertainty
AI 理解论文
溯源树
样例
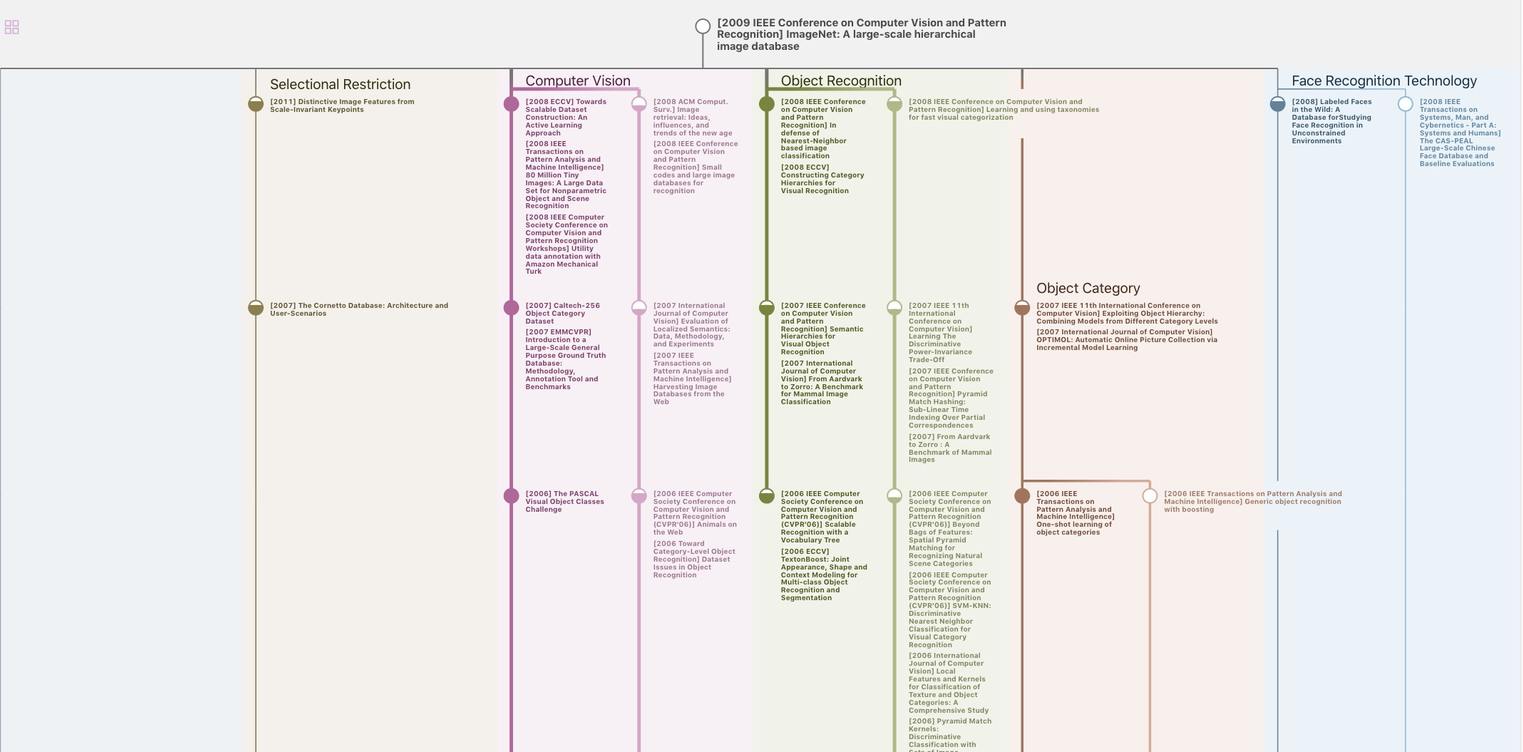
生成溯源树,研究论文发展脉络
Chat Paper
正在生成论文摘要