Learning Phone Recognition From Unpaired Audio and Phone Sequences Based on Generative Adversarial Network
IEEE/ACM Transactions on Audio, Speech and Language Processing(2022)
摘要
AbstractASRhas been shown to achieve great performance recently. However, most of them rely on massive paired data, which is not feasible for low-resource languages worldwide. This paper investigates how to learn directly from unpaired phone sequences and speech utterances. We design a two-stage iterative framework. GAN training is adopted in the first stage to find the mapping relationship between unpaired speech and phone sequence. In the second stage, another HMM model is introduced to train from the generator’s output, which boosts the performance and provides a better segmentation for the next iteration. In the experiment, we first investigate different choices of model designs. Thenwe compare the framework to different types of baselines: (i) supervised methods (ii) acoustic unit discovery based methods (iii) methods learning from unpaired data. Our framework performs consistently better than all acoustic unit discovery methods and previous methods learning from unpaired data based on the TIMIT dataset.
更多查看译文
关键词
Hidden Markov models, Training, Generators, Speech recognition, Acoustics, Generative adversarial networks, Data models, Generative adversarial network, phone recognition, unsupervised learning
AI 理解论文
溯源树
样例
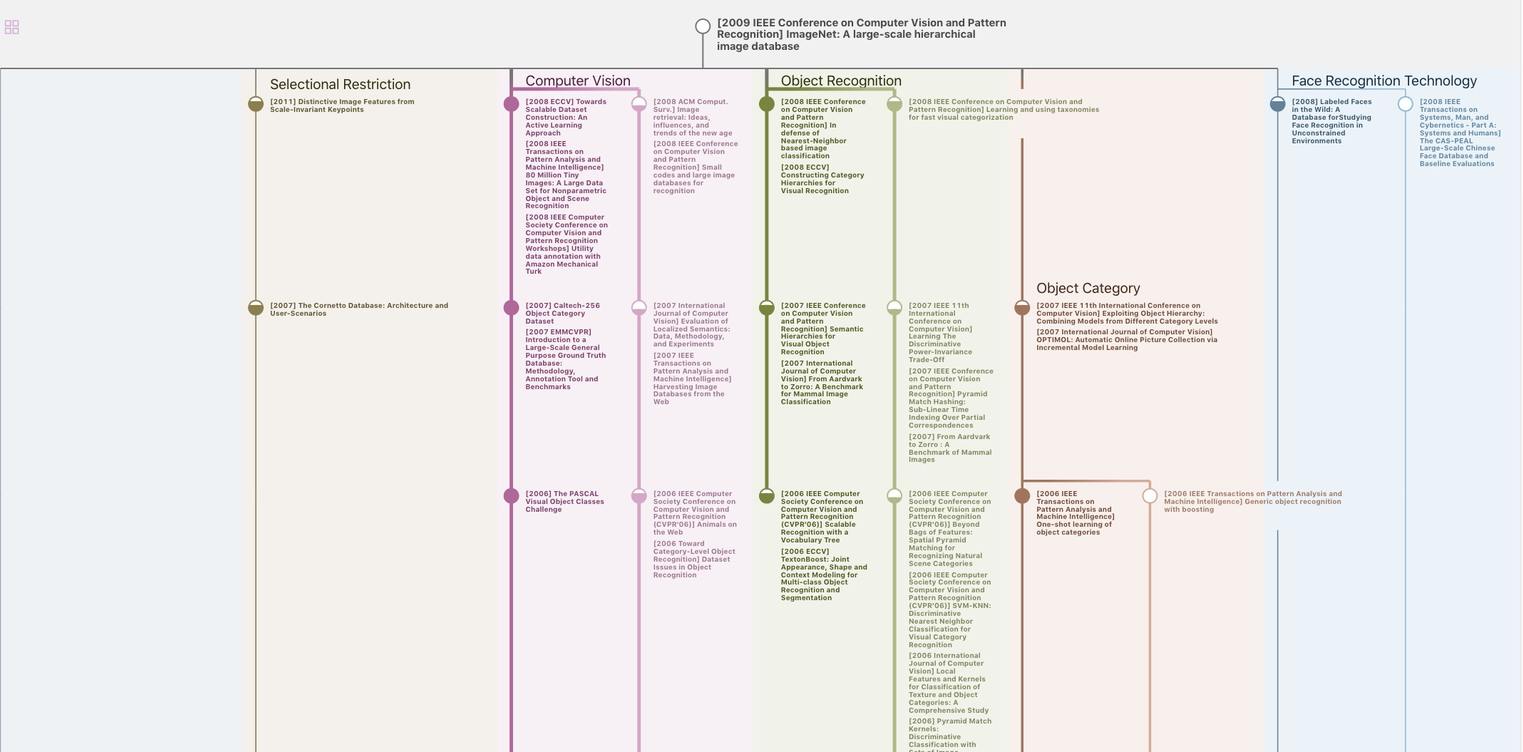
生成溯源树,研究论文发展脉络
Chat Paper
正在生成论文摘要