EXPERT: Public Benchmarks for Dynamic Heterogeneous Academic Graphs
CoRR(2022)
摘要
Machine learning models that learn from dynamic graphs face nontrivial challenges in learning and inference as both nodes and edges change over time. The existing large-scale graph benchmark datasets that are widely used by the community primarily focus on homogeneous node and edge attributes and are static. In this work, we present a variety of large scale, dynamic heterogeneous academic graphs to test the effectiveness of models developed for multi-step graph forecasting tasks. Our novel datasets cover both context and content information extracted from scientific publications across two communities: Artificial Intelligence (AI) and Nuclear Nonproliferation (NN). In addition, we propose a systematic approach to improve the existing evaluation procedures used in the graph forecasting models.
更多查看译文
关键词
public benchmarks,graphs
AI 理解论文
溯源树
样例
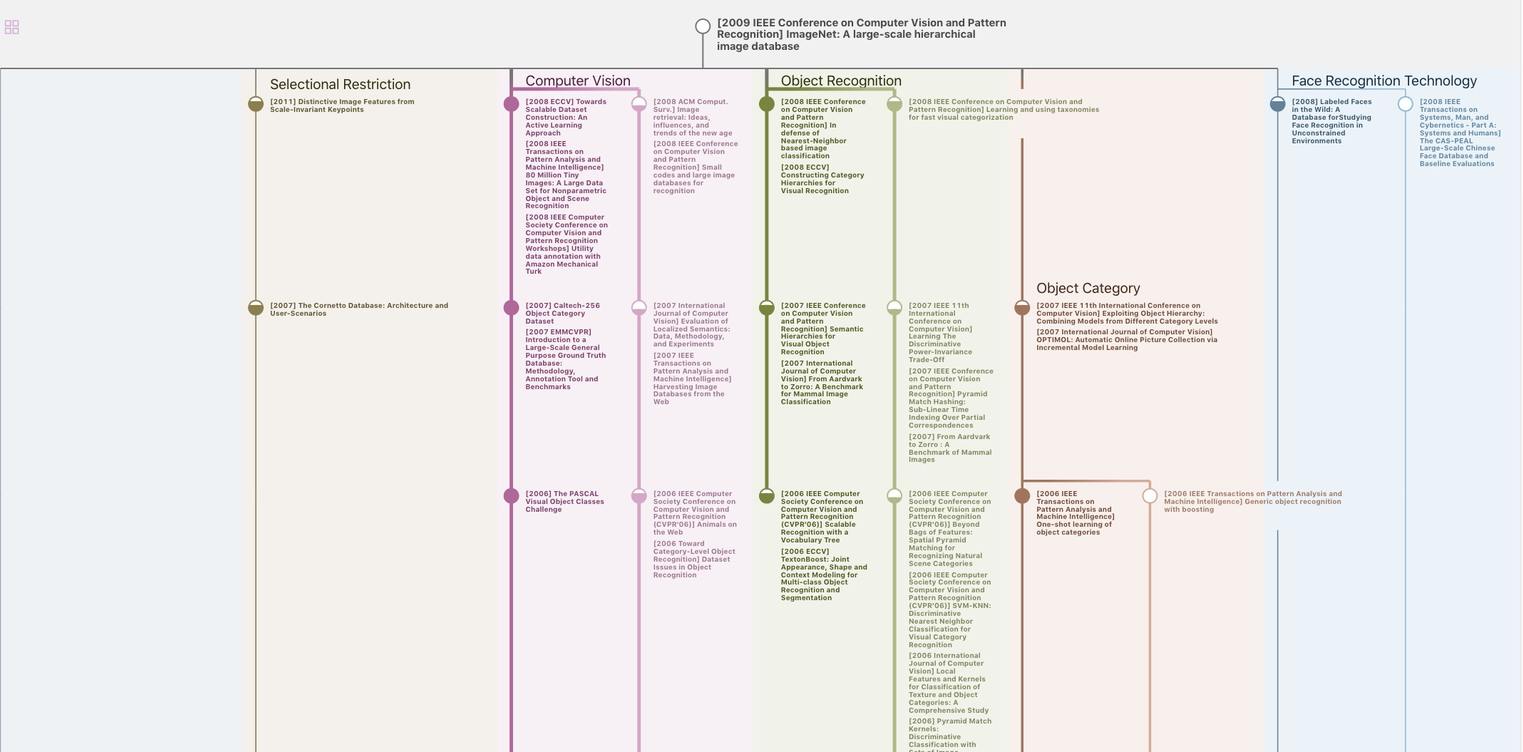
生成溯源树,研究论文发展脉络
Chat Paper
正在生成论文摘要