Machine learning to predict vasopressin responsiveness in patients with septic shock
PHARMACOTHERAPY(2022)
摘要
Study Objectives The objective of this study was to develop and externally validate a model to predict adjunctive vasopressin response in patients with septic shock being treated with norepinephrine for bedside use in the intensive care unit. Design This was a retrospective analysis of two adult tertiary intensive care unit septic shock populations. Setting Barnes-Jewish Hospital (BJH) from 2010 to 2017 and Beth Israel Deaconess Medical Center (BIDMC) from 2001 to 2012. Patients Two septic shock populations (548 BJH patients and 464 BIDMC patients) that received vasopressin as second-line vasopressor. Intervention Patients who were vasopressin responsive were compared with those who were nonresponsive. Vasopressin response was defined as survival with at least a 20% decrease in maximum daily norepinephrine requirements by one calendar day after vasopressin initiation, without a third-line vasopressor. Measurements Two supervised machine learning models (gradient-boosting machine [XGBoost] and elastic net penalized logistic regression [EN]) were trained in 1000 bootstrap replications of the BJH data and externally validated in the BIDMC data to predict vasopressin responsiveness. Main Results Vasopressin responsiveness was similar among each cohort (BJH 45% and BIDMC 39%). Mortality was lower for vasopressin responders compared with nonresponders in the BJH (51% vs. 73%) and BIDMC (45% vs. 83%) cohorts, respectively. Both models demonstrated modest discrimination in the training (XGBoost area under receiver operator curve [AUROC] 0.61 [95% confidence interval (CI) 0.61-0.61], EN 0.59 [95% CI 0.58-0.59]) and external validation (XGBoost 0.68 [95% CI 0.63-0.73], EN 0.64 [95% CI 0.59-0.69]) datasets. Conclusion Vasopressin nonresponsiveness is common and associated with increased mortality. The models' modest performances highlight the complexity of septic shock and indicate that more research will be required before clinical decision support tools can aid in anticipating patient-specific responsiveness to vasopressin.
更多查看译文
关键词
artificial intelligence, pharmacology, predictive modeling, septic shock, vasopressors
AI 理解论文
溯源树
样例
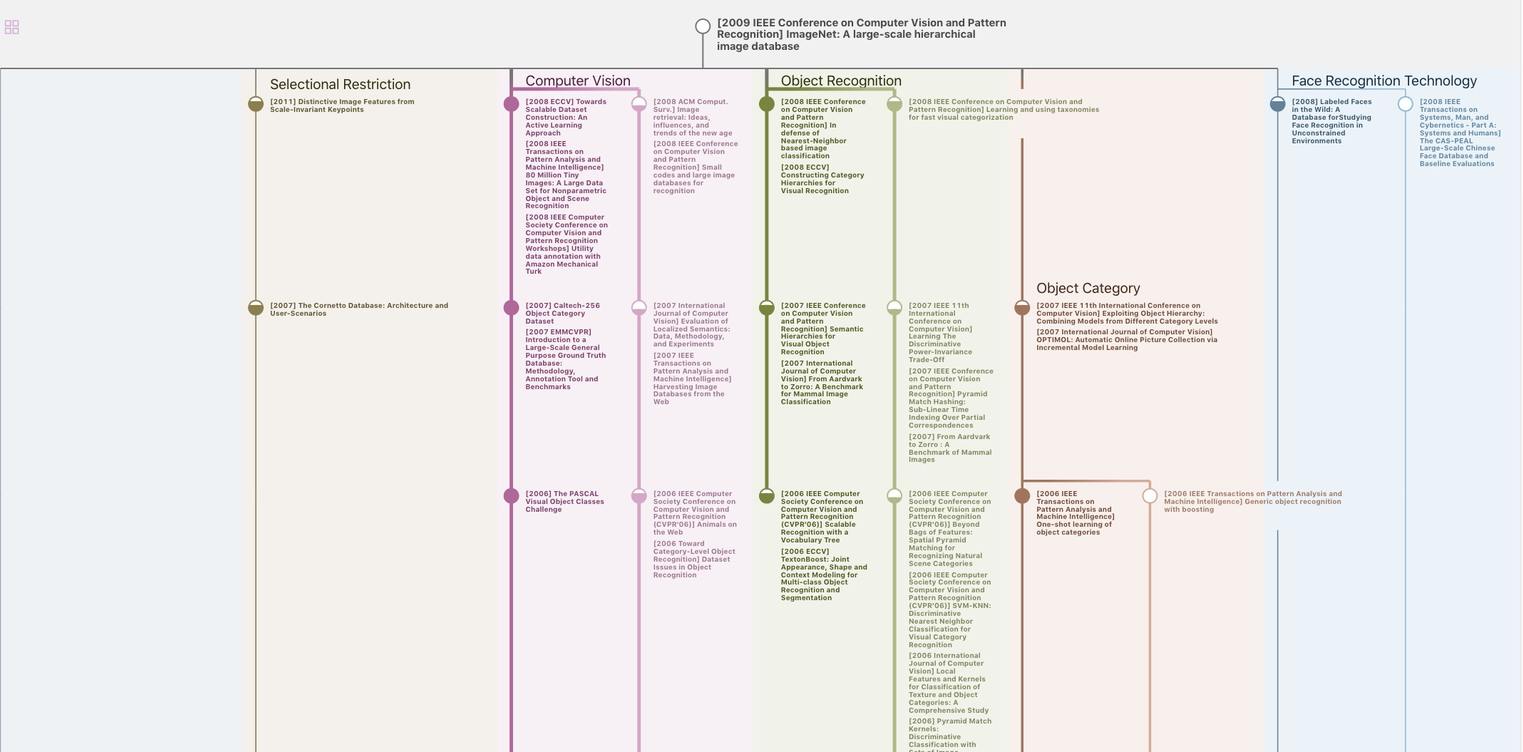
生成溯源树,研究论文发展脉络
Chat Paper
正在生成论文摘要