Supervised dimension reduction for optical vapor sensing
RSC ADVANCES(2022)
摘要
Detecting and identifying vapors at low concentrations is important for air quality assessment, food quality assurance, and homeland security. Optical vapor sensing using photonic crystals has shown promise for rapid vapor detection and identification. Despite the recent advances of optical sensing using photonic crystals, the data analysis method commonly used in this field has been limited to an unsupervised method called principal component analysis (PCA). In this study, we applied four different supervised dimension reduction methods on differential reflectance spectra data from optical vapor sensing experiments. We found that two of the supervised methods, linear discriminant analysis and least-squares regression PCA, yielded better interclass separation, vapor identification and improved classification accuracy compared to PCA.
更多查看译文
关键词
pH Sensing,Optical Sensors
AI 理解论文
溯源树
样例
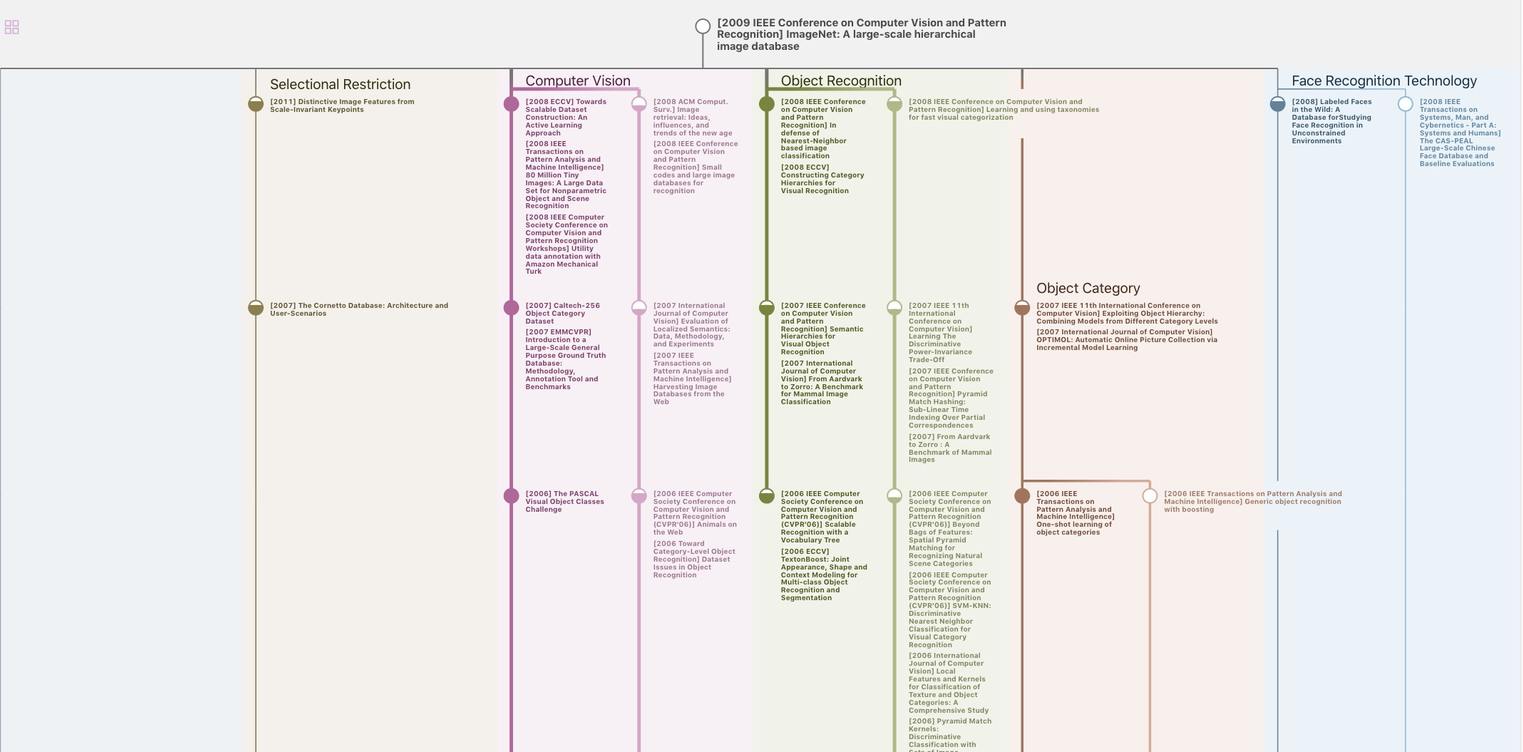
生成溯源树,研究论文发展脉络
Chat Paper
正在生成论文摘要