High-resolution mapping of wildfire drivers in California based on machine learning.
The Science of the total environment(2022)
Abstract
Wildfires are important natural disturbances of ecosystems; however, they threaten the sustainability of ecosystems, climate and humans worldwide. It is vital to quantify and map the controlling drivers of wildfires for effective wildfire prediction and risk management. However, high-resolution mapping of wildfire drivers remains challenging. Here we established machine-learning (Random Forests) models using 23 climate and land surface variables as model inputs to reconstruct the spatial variability and seasonality of wildfire occurrence and extent in California. The importance of individual drivers was then quantified based on the Shapley value method. Thus, we provided spatially resolved maps of wildfire drivers at high resolutions up to 0.004° × 0.004°. The results indicated that precipitation and soil moisture are the major drivers dominating 37% of the total burnt area for large and extreme wildfires in summer and 63% in autumn, while elevation plays a major role for 15-58% of burnt areas in small wildfires in all seasons. Winds are also an important contributor to summer wildfires, accounting for 41% of large and extreme burnt areas. This study enhanced our knowledge of spatial variability of wildfire drivers across diverse landscapes in a fine-scale mapping, providing valuable perspectives and case studies for other regions of the world with frequently occurred wildfire.
MoreTranslated text
AI Read Science
Must-Reading Tree
Example
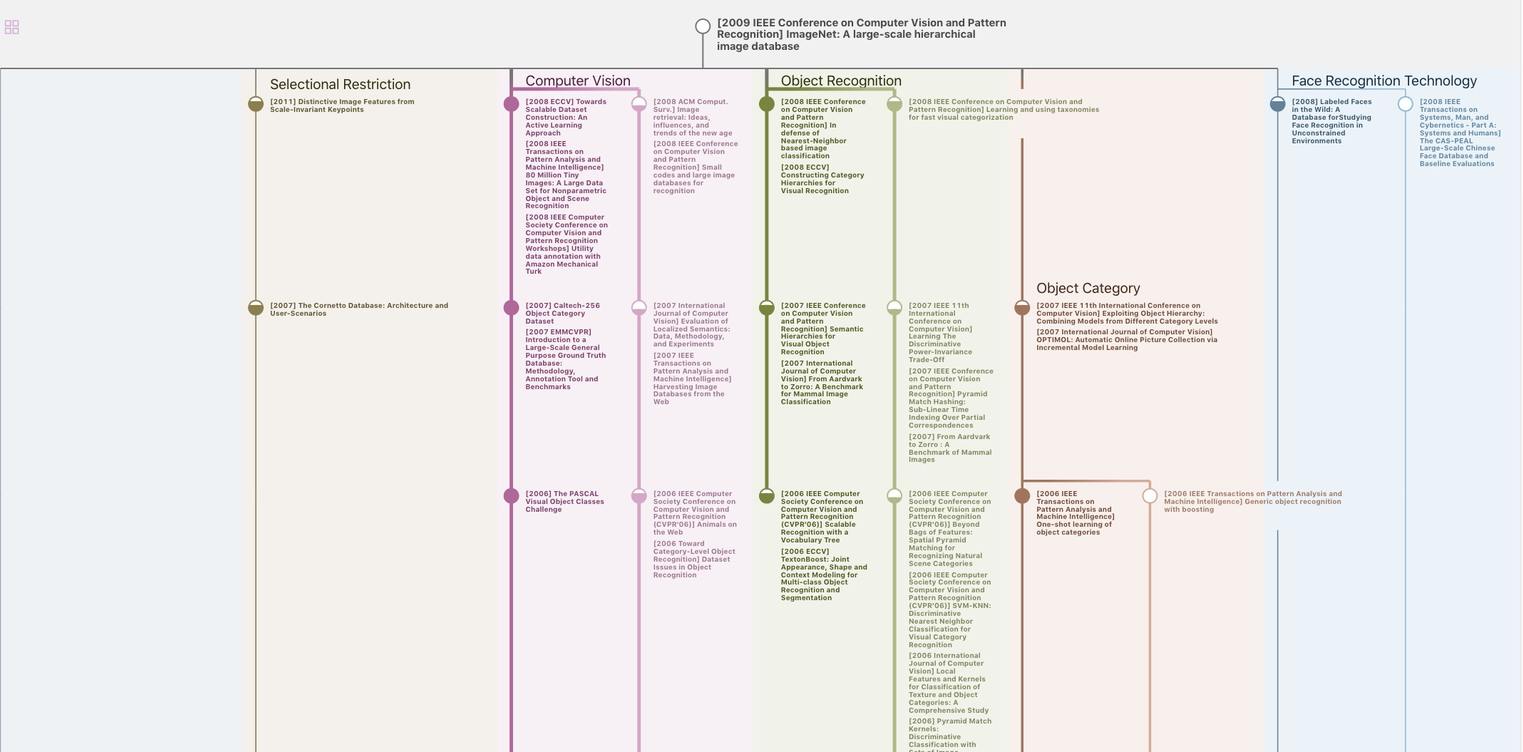
Generate MRT to find the research sequence of this paper
Chat Paper
Summary is being generated by the instructions you defined