GRAND++: Graph Neural Diffusion with A Source Term
International Conference on Learning Representations (ICLR)(2022)
摘要
We propose GRAph Neural Diffusion with a source term (GRAND++) for graph deep learning with a limited number of labeled nodes, i.e., low-labeling rate. GRAND++ is a class of continuous-depth graph deep learning architectures whose theoretical underpinning is the diffusion process on graphs with a source term. The source term guarantees two interesting theoretical properties of GRAND++: (i) the representation of graph nodes, under the dynamics of GRAND++, will not converge to a constant vector over all nodes even as the time goes to infinity, which mitigates the over-smoothing issue of graph neural networks and enables graph learning in very deep architectures. (ii) GRAND++ can provide accurate classification even when the model is trained with a very limited number of labeled training data. We experimentally verify the above two advantages on various graph deep learning benchmark tasks, showing a significant improvement over many existing graph neural networks.
更多查看译文
关键词
graph deep learning,low-labeling rates,diffusion on graphs,random walk
AI 理解论文
溯源树
样例
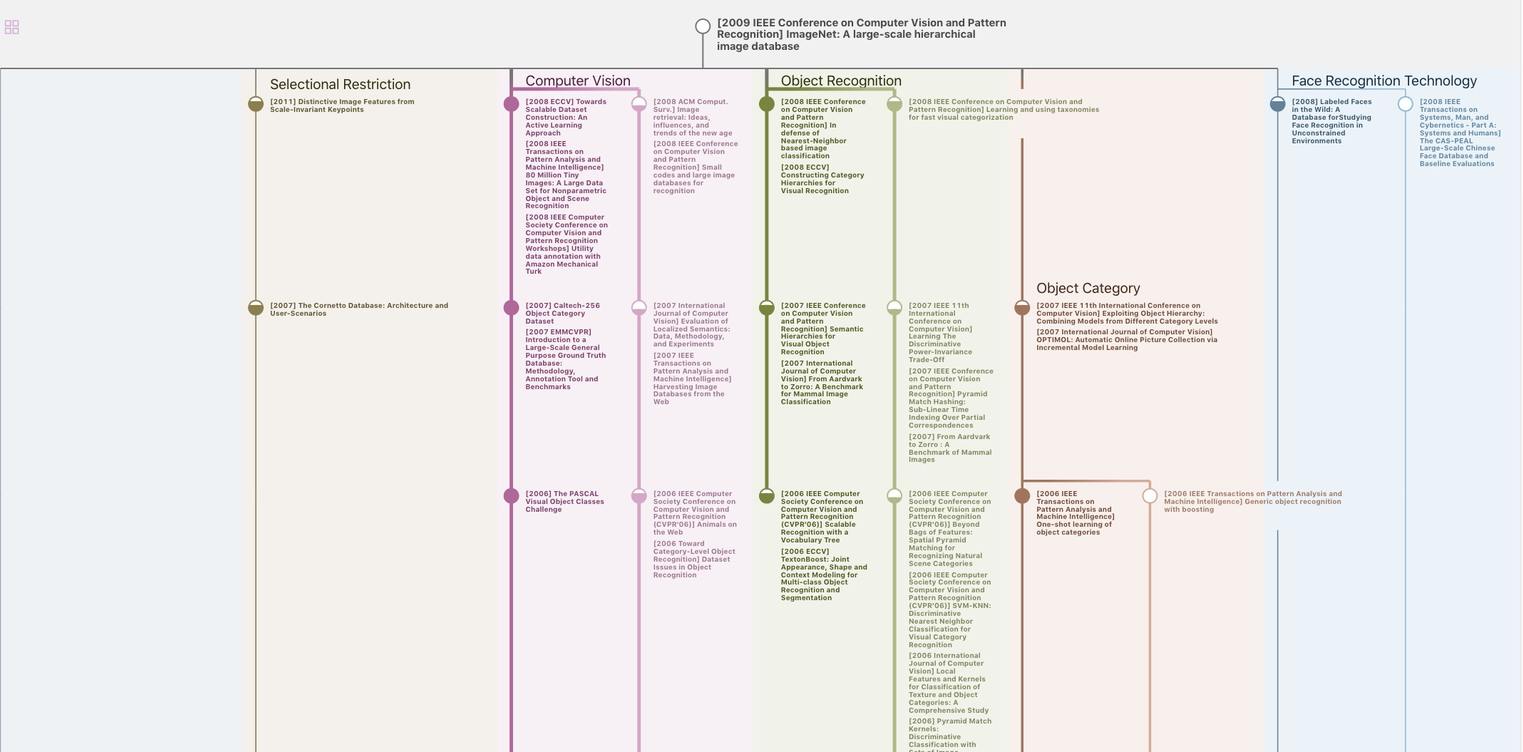
生成溯源树,研究论文发展脉络
Chat Paper
正在生成论文摘要