Generative Pseudo-Inverse Memory
International Conference on Learning Representations (ICLR)(2022)
摘要
We propose Generative Pseudo-Inverse Memory (GPM), a class of deep generative memory models that are fast to write in and read out. Memory operations are recast as seeking robust solutions of linear systems, which naturally lead to the use of matrix pseudo-inverses. The pseudo-inverses are iteratively approximated, with practical computation complexity of almost $O(1)$. We prove theoretically and verify empirically that our model can retrieve exactly what have been written to the memory under mild conditions. A key capability of GPM is iterative reading, during which the attractor dynamics towards fixed points are enabled, allowing the model to iteratively improve sample quality in denoising and generating. More impressively, GPM can store a large amount of data while maintaining key abilities of accurate retrieving of stored patterns, denoising of corrupted data and generating novel samples. Empirically we demonstrate the efficiency and versatility of GPM on a comprehensive suite of experiments involving binarized MNIST, binarized Omniglot, FashionMNIST, CIFAR10 & CIFAR100 and CelebA.
更多查看译文
AI 理解论文
溯源树
样例
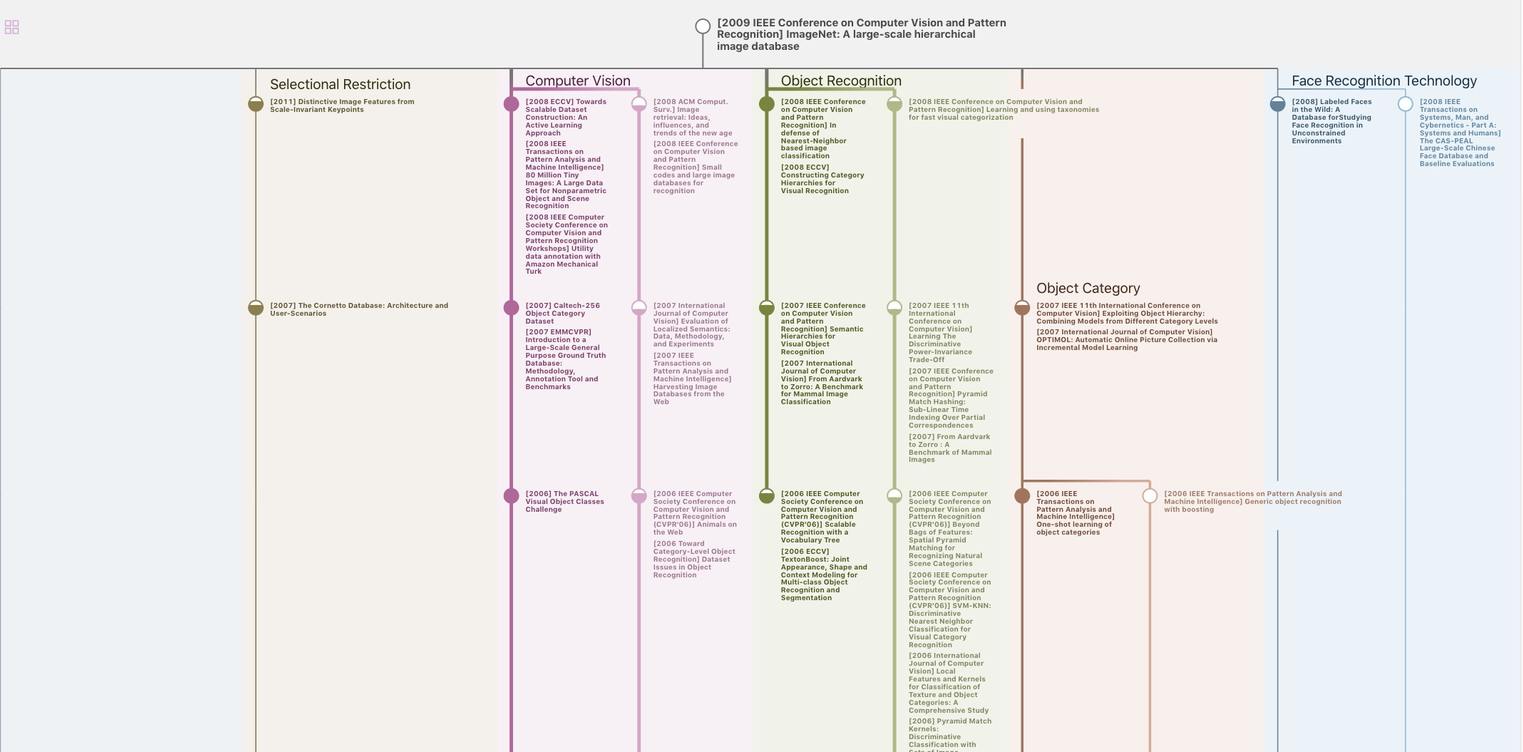
生成溯源树,研究论文发展脉络
Chat Paper
正在生成论文摘要