Practical Integration via Separable Bijective Networks
International Conference on Learning Representations (ICLR)(2022)
摘要
Neural networks have enabled learning over examples that contain thousands of dimensions.
However, most of these models are limited to training and evaluating on a finite collection of \textit{points} and do not consider the hypervolume in which the data resides.
Any analysis of the model's local or global behavior is therefore limited to very expensive or imprecise estimators.
We propose to formulate neural networks as a composition of a bijective (flow) network followed by a learnable, separable network.
This construction allows for learning (or assessing) over full hypervolumes with precise estimators at tractable computational cost via integration over the \textit{input space}.
We develop the necessary machinery, propose several practical integrals to use during training, and demonstrate their utility.
更多查看译文
关键词
integration,flow,likelihood,classification,regression,out of distribution,regularization
AI 理解论文
溯源树
样例
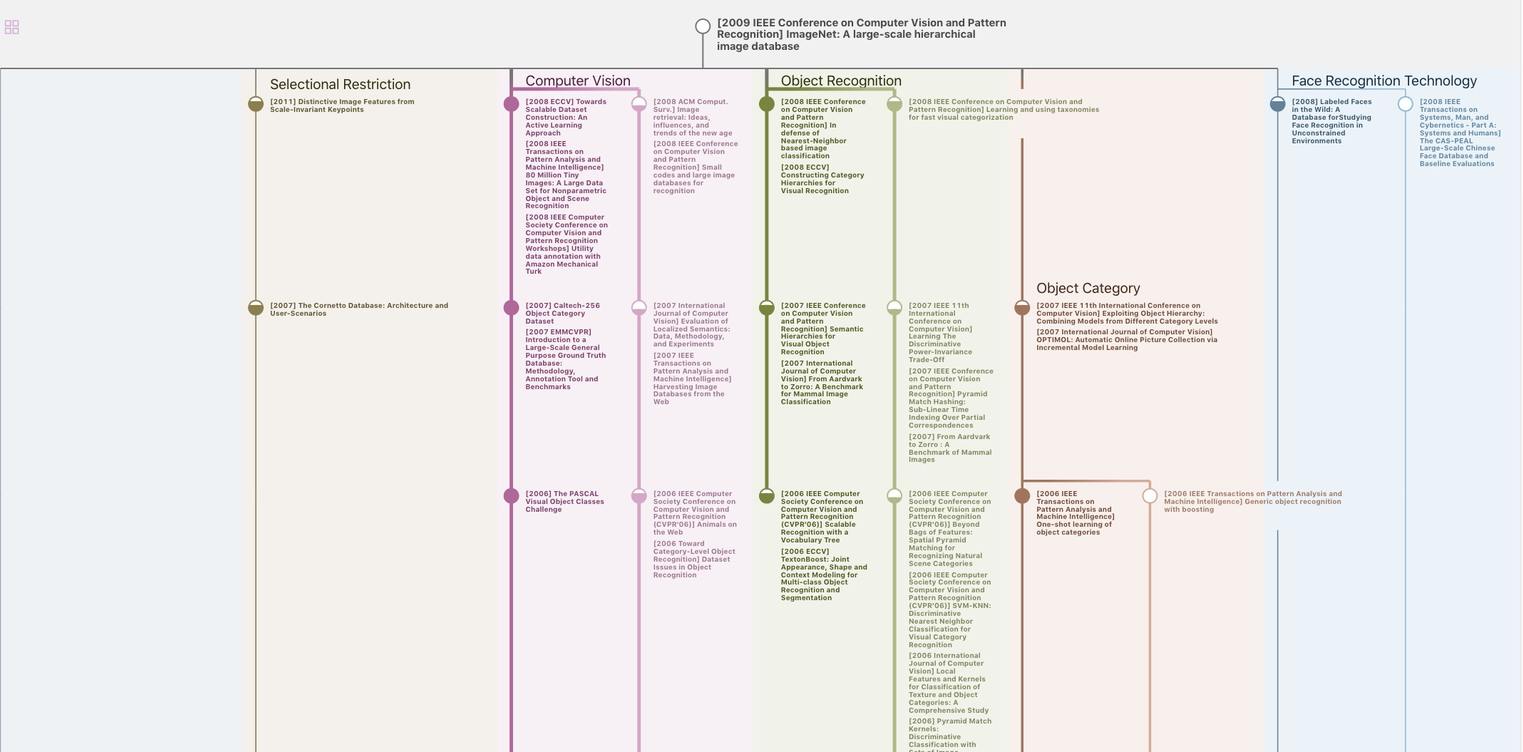
生成溯源树,研究论文发展脉络
Chat Paper
正在生成论文摘要