Online Ad Hoc Teamwork under Partial Observability
International Conference on Learning Representations (ICLR)(2022)
摘要
Autonomous agents often need to work together as a team to accomplish complex cooperative tasks. Due to privacy and other realistic constraints, agents might need to collaborate with previously unknown teammates on the fly. This problem is known as ad hoc teamwork, which remains a core research challenge. Prior works usually rely heavily on strong assumptions like full observability, fixed and predefined teammates' types. This paper relaxes these assumptions with a novel reinforcement learning framework called ODITS, which allows the autonomous agent to adapt to arbitrary teammates in an online fashion. Instead of limiting teammates into a finite set of predefined types, ODITS automatically learns latent variables of teammates' behaviors to infer how to cooperate with new teammates effectively. To overcome partial observability, we introduce an information-based regularizer to derive proxy representations of the learned variables from local observations. Extensive experimental results show that ODITS significantly outperforms various baselines in widely used ad hoc teamwork tasks.
更多查看译文
关键词
coordination,reinforcement learning
AI 理解论文
溯源树
样例
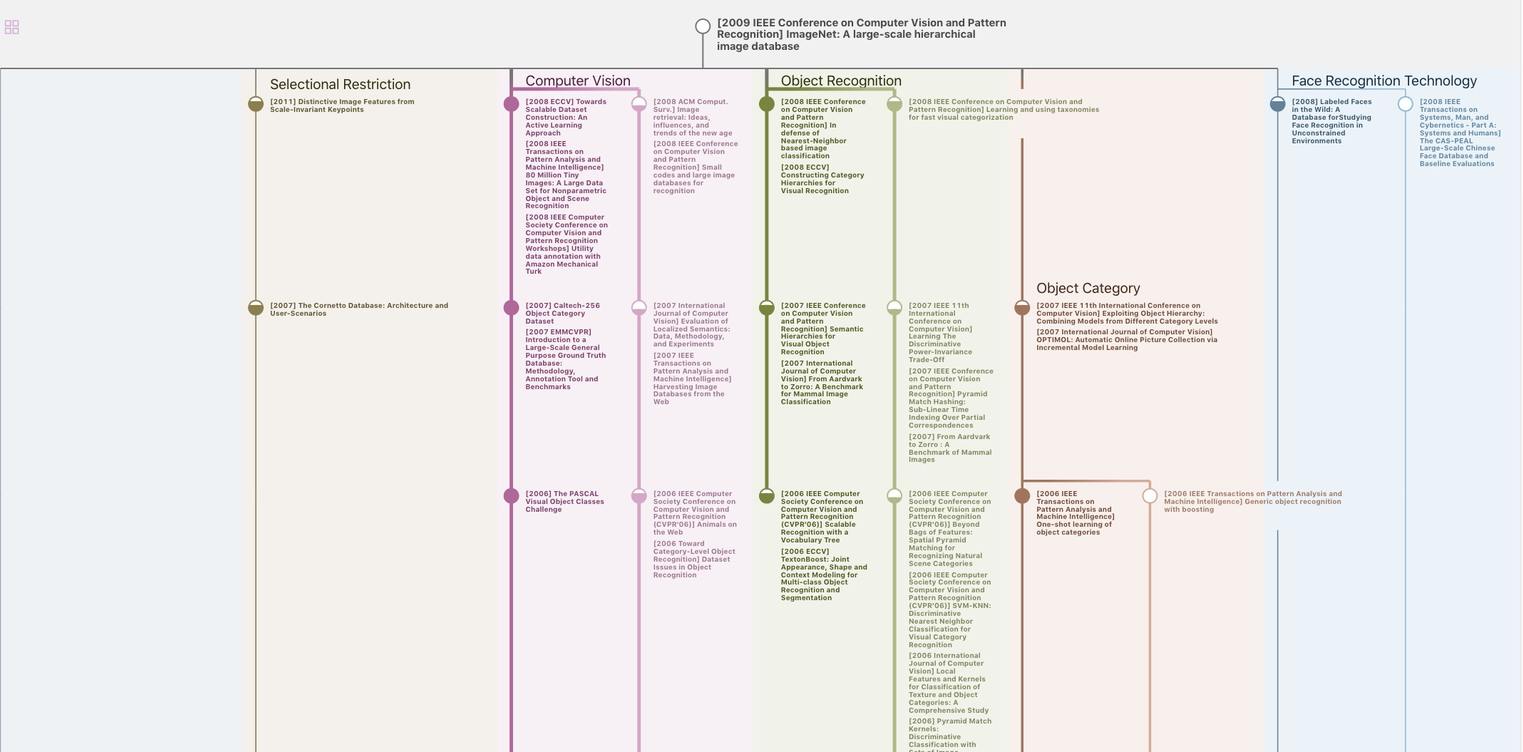
生成溯源树,研究论文发展脉络
Chat Paper
正在生成论文摘要