Specifying prior distributions in reliability applications
APPLIED STOCHASTIC MODELS IN BUSINESS AND INDUSTRY(2024)
Abstract
Especially when facing reliability data with limited information (e.g., a small number of failures), there are strong motivations for using Bayesian inference methods. These include the option to use information from physics-of-failure or previous experience with a failure mode in a particular material to specify an informative prior distribution. Another advantage is the ability to make statistical inferences without having to rely on specious (when the number of failures is small) asymptotic theory needed to justify non-Bayesian methods. Users of non-Bayesian methods are faced with multiple methods of constructing uncertainty intervals (Wald, likelihood, and various bootstrap methods) that can give substantially different answers when there is little information in the data. For Bayesian inference, there is only one method of constructing equal-tail credible intervals-but it is necessary to provide a prior distribution to fully specify the model. Much work has been done to find default prior distributions that will provide inference methods with good (and in some cases exact) frequentist coverage properties. This paper reviews some of this work and provides, evaluates, and illustrates principled extensions and adaptations of these methods to the practical realities of reliability data (e.g., non-trivial censoring).
MoreTranslated text
Key words
Bayesian inference,default prior,few failures,fisher information matrix,Jeffreys prior,noninformative prior,reference prior
AI Read Science
Must-Reading Tree
Example
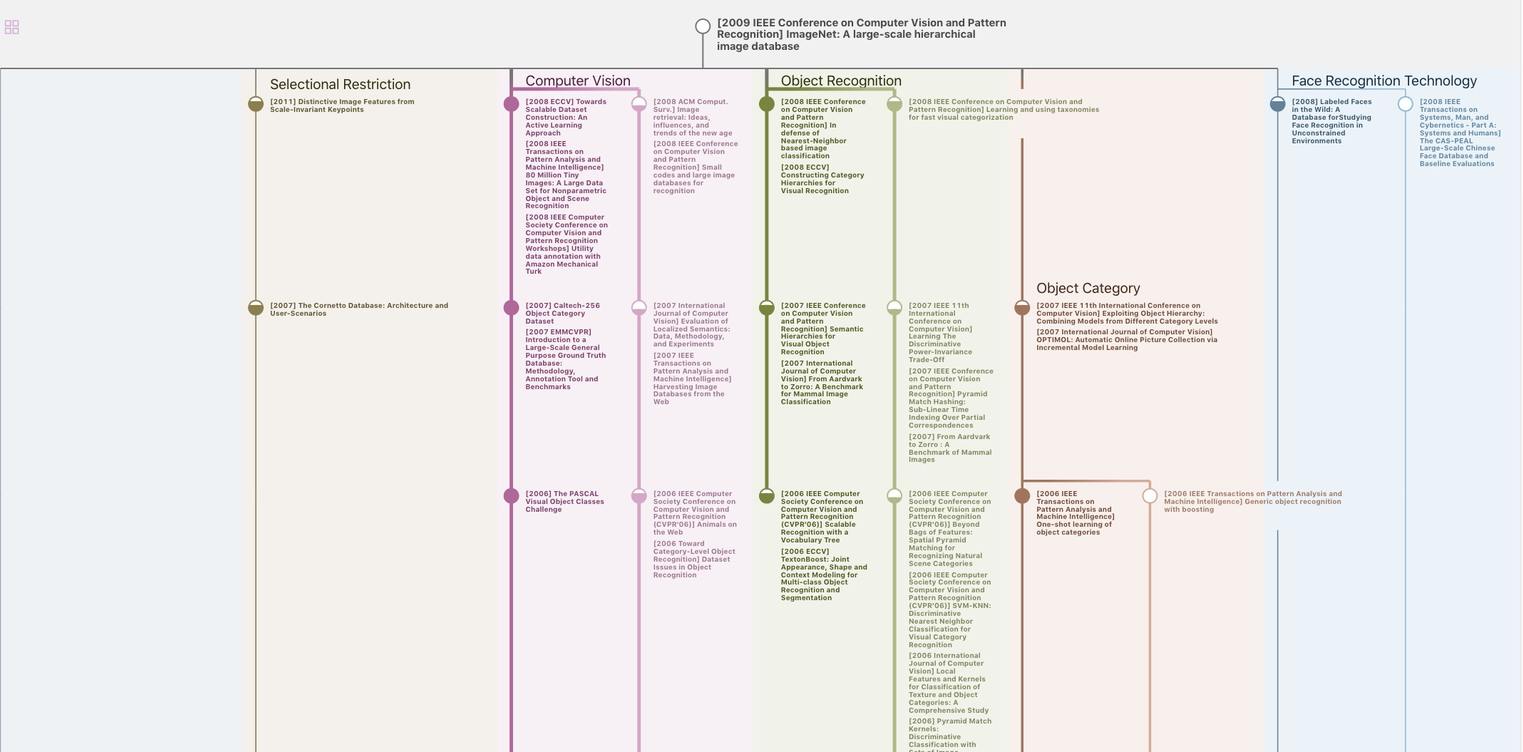
Generate MRT to find the research sequence of this paper
Chat Paper
Summary is being generated by the instructions you defined