Stability Analysis of Substituted Cobaltocenium[Bis(cyclopentadienyl)cobalt(III)] Employing Chemistry-Informed Neural Networks
JOURNAL OF CHEMICAL THEORY AND COMPUTATION(2022)
摘要
Cationic cobaltocenium derivatives are promisingcomponents of the anion exchange membranes because of theirexcellent thermal and alkaline stability under the operatingconditions of a fuel cell. Here, we present an efficient modelingapproach to assessing the chemical stability of substitutedcobaltocenium CoCp2+,basedonthecomputedelectronicstructure enhanced by machine learning techniques. Within theaqueous environment, the positive charge of the metal cation isbalanced by the hydroxide anion through formation of theCoCp2+OH-complexes, whose dissociation is studied within the implicit solvent employing the density functional theory. Thedata set of about 118 the CoCp2+OH-complexes based on 42 substituent groups characterized by a range of electron-donating(ED) and electron-withdrawing (EW) properties is constructed and analyzed. Given 12 carefully chosen chemistry-informeddescriptors of the complexes and relevant fragments, the stability of the complexes is found to strongly correlate with the energies ofthe highest occupied and lowest unoccupied molecular orbitals, modulated by a switching function of the Hirshfeld charge. Thelatter is used as a measure of the electron-withdrawing-donating character of the substituents. On the basis of this observation fromthe conventional regression analysis, two fully connected, feed-forward neural network (FNN) models with different unit structures,called the chemistry-informed (CINN) and the quadratic (QNN) neural networks, together with a support vector regression (SVR)model are developed. Both deep neural network models predict the bond dissociation energies of the cobaltocenium complexes withmean relative errors less than 10.56% and average absolute errors less than 1.63 kcal/mol, superior to the conventional regressionand the SVR model prediction. The results show the potential of QNN to efficiently capture more complex relationships. Theconcept of incorporating the domain (chemical) knowledge/insight into the neural network structure paves the way to applicationsof machine learning techniques with small data sets, ultimately leading to better predictive models compared to the classical machinelearning method SVR and conventional regression analysis.
更多查看译文
AI 理解论文
溯源树
样例
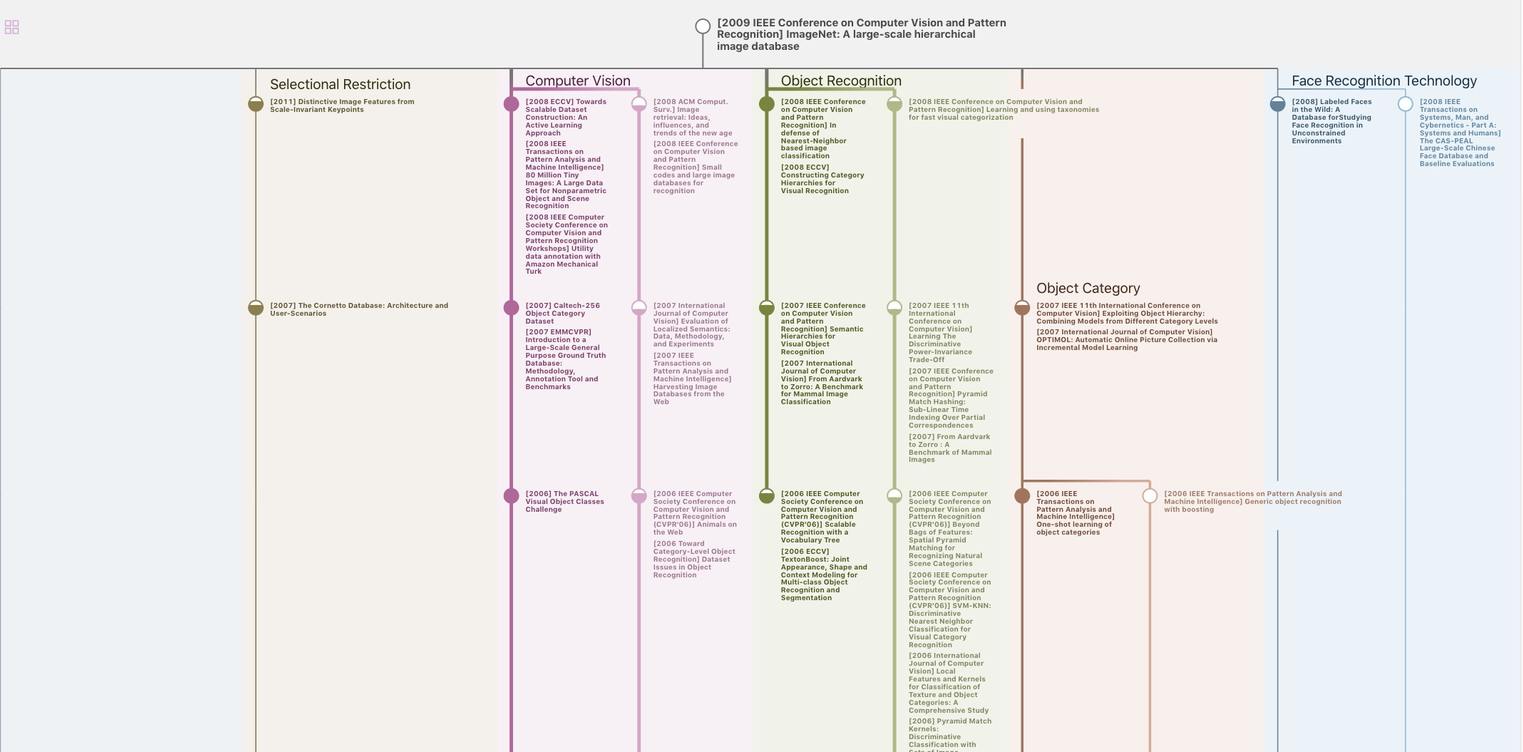
生成溯源树,研究论文发展脉络
Chat Paper
正在生成论文摘要