A zeroing neural dynamics based acceleration optimization approach for optimizers in deep neural networks
Neural Networks(2022)
摘要
The first-order optimizers in deep neural networks (DNN) are of pivotal essence for a concrete loss function to reach the local minimum or global one on the loss surface within convergence time. However, each optimizer possesses its own superiority and virtue when encountering a specific application scene and environment. In addition, the existing modified optimizers mostly emphasize a given optimizer without any transfer property. In this paper, a zeroing neural dynamics (ZND) based optimization approach for the first-order optimizers is proposed, which can assist ZND via the activation function to expedite the process of solving gradient information, with lower loss and higher accuracy. To the best of our knowledge, it is the first work to integrate the ZND in control domain with the first-order optimizers in DNN. This generic work is an optimization method for the most commonly-used first-order optimizers to handle different application scenes, rather than developing a brand-new algorithm besides the existing optimizers or their modifications. Furthermore, mathematic derivations concerning the gradient information transformation of the ZND are systematically provided. Finally, comparison experiments are implemented, which demonstrates the effectiveness of the proposed approach with different loss functions and network frameworks on the Reuters, CIFAR, and MNIST data sets.
更多查看译文
关键词
Zeroing neural dynamics (ZND),Optimizer,Deep neural networks (DNN),Optimization
AI 理解论文
溯源树
样例
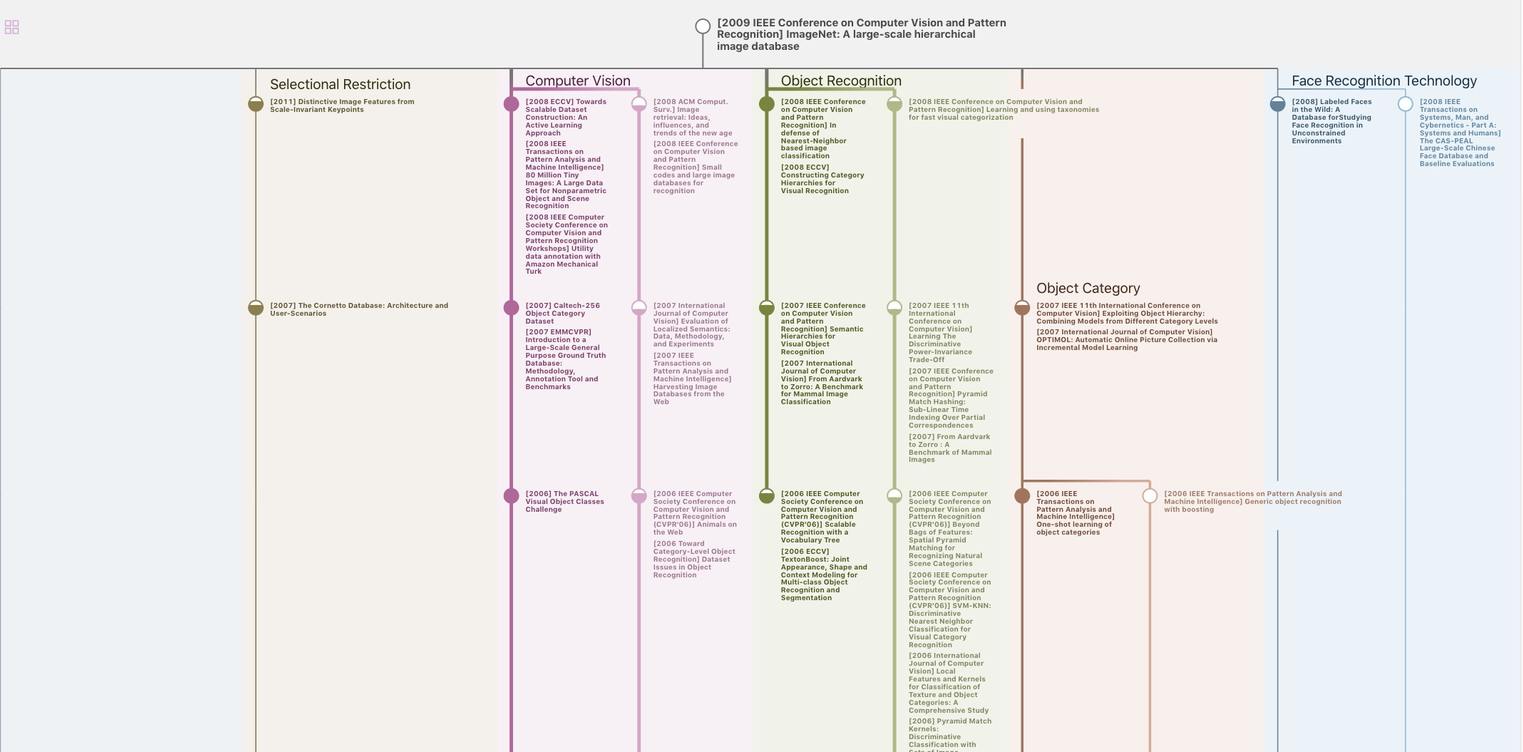
生成溯源树,研究论文发展脉络
Chat Paper
正在生成论文摘要