Using an object-based machine learning ensemble approach to upscale evapotranspiration measured from eddy covariance towers in a subtropical wetland
Science of The Total Environment(2022)
摘要
Accurate prediction of evapotranspiration (ET) in wetlands is critical for understanding the coupling effects of water, carbon, and energy cycles in terrestrial ecosystems. Multiple years of eddy covariance (EC) tower ET measurements at five representative wetland ecosystems in the subtropical Big Cypress National Preserve (BCNP), Florida (USA) provide a unique opportunity to assess the performance of the Moderate Resolution Imaging Spectroradiometer (MODIS) ET operational product MOD16A2 and upscale tower measured ET to generate local/regional wetland ET maps. We developed an object-based machine learning ensemble approach to evaluate and map wetland ET by linking tower measured ET with key predictors from MODIS products and meteorological variables. The results showed MOD16A2 had poor performance in characterizing ET patterns and was unsatisfactory for estimating ET over four wetland communities where Nash-Sutcliffe model Efficiency (NSE) was less than 0.5. In contrast, the site-specific machine learning ensemble model had a high predictive power with a NSE larger than 0.75 across all EC sites. We mapped the ET rate for two distinctive seasons and quantified the prediction diversity to identify regions easier or more challenging to estimate from model-based analyses. An integration of MODIS products and other datasets through the machine learning upscaling paradigm is a promising tool for local wetland ET mapping to guide regional water resource management.
更多查看译文
关键词
Wetland evapotranspiration estimation,Machine learning ensemble,Wetland ET upscaling,Everglades
AI 理解论文
溯源树
样例
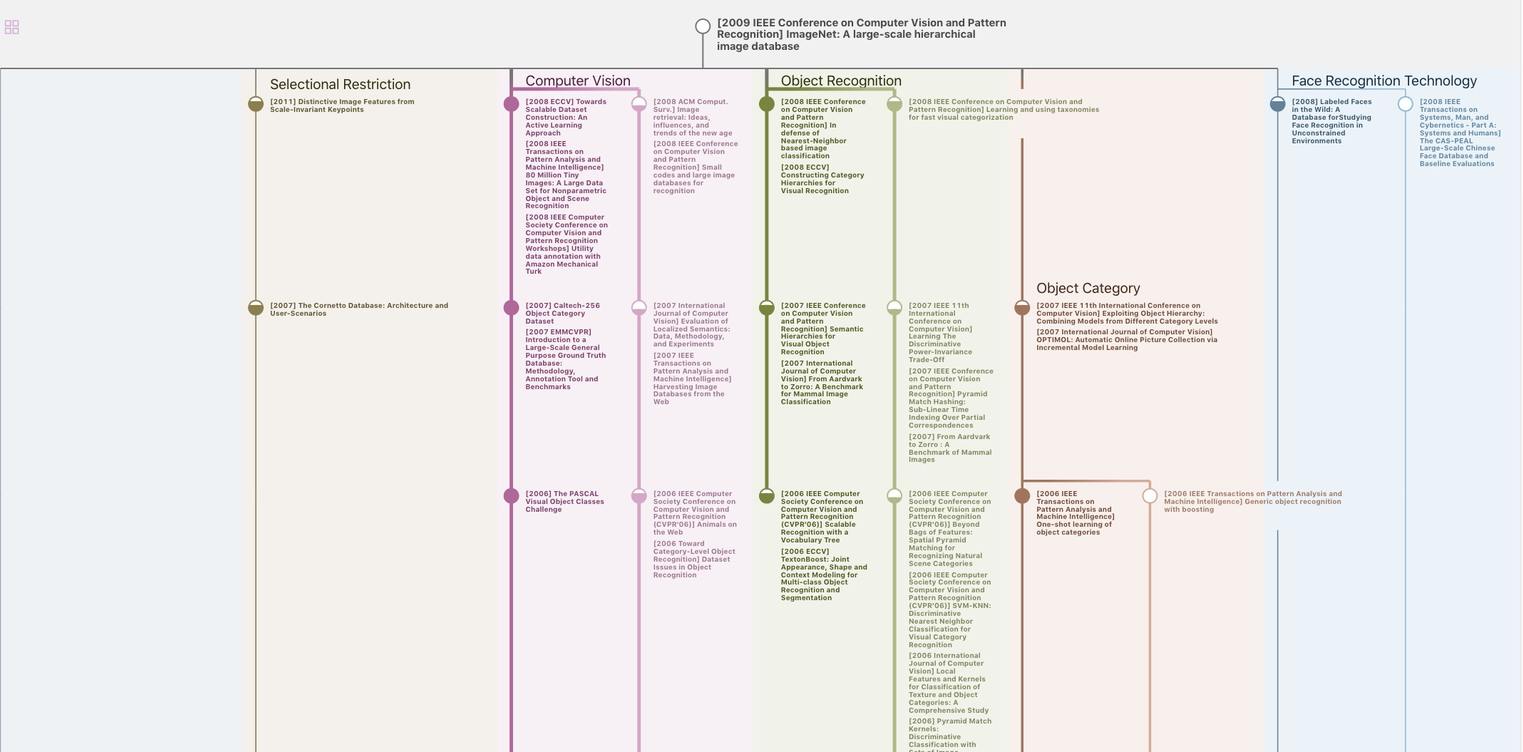
生成溯源树,研究论文发展脉络
Chat Paper
正在生成论文摘要