Multi-trait genomic prediction improves selection accuracy for enhancing seed mineral concentrations in pea (Pisum sativum L.)
biorxiv(2022)
摘要
The superiority of multi-trait genomic selection (MT-GS) over univariate genomic selection (UNI-GS) can be improved by redesigning the phenotyping strategy. In this study, we used about 300 advanced breeding lines from North Dakota State University (NDSU) pulse breeding program and about 200 USDA accessions evaluated for ten nutritional traits to assess the efficiency of sparse testing in MT-GS. Our results showed that sparse phenotyping using MT-GS consistently outperformed UNI-GS when compared to partially balanced phenotyping using MT-GS. This strategy can be further extended to multi-environment multi-trait GS to improve prediction performance and reduce the cost of phenotyping and time-consuming data collection process. Given that MT-GS relies on borrowing information from genetically correlated traits and relatives, consideration should be given to trait combinations in the training and prediction sets to improve model parameters estimate and ultimately prediction performance. Our results point to heritability and genetic correlation between traits as possible parameters to achieve this objective.
### Competing Interest Statement
The authors have declared no competing interest.
更多查看译文
关键词
seed mineral concentrations,pea,selection accuracy,multi-trait
AI 理解论文
溯源树
样例
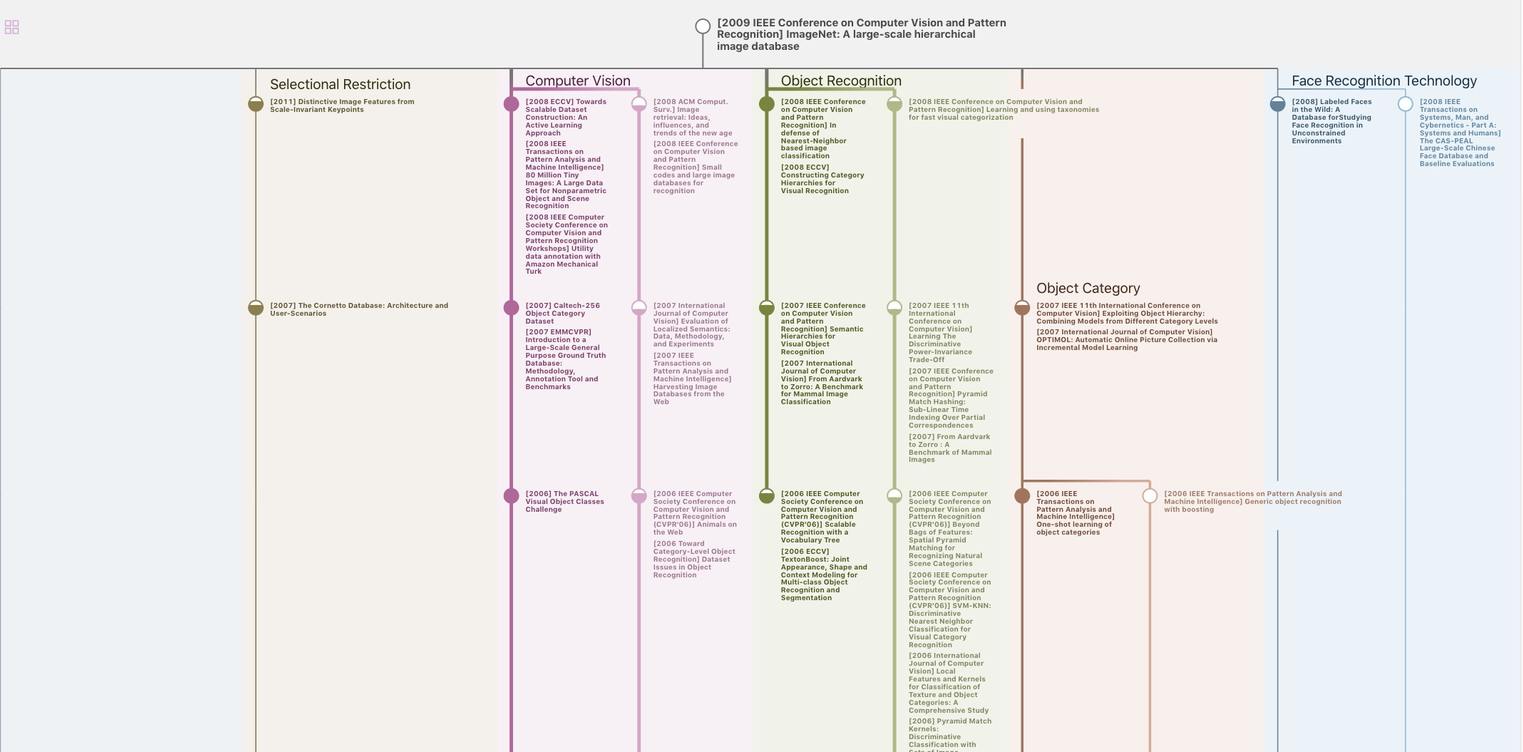
生成溯源树,研究论文发展脉络
Chat Paper
正在生成论文摘要