A Collection of Deep Learning-based Feature-Free Approaches for Characterizing Single-Objective Continuous Fitness Landscapes
PROCEEDINGS OF THE 2022 GENETIC AND EVOLUTIONARY COMPUTATION CONFERENCE (GECCO'22)(2022)
摘要
Exploratory Landscape Analysis is a powerful technique for numerically characterizing landscapes of single-objective continuous optimization problems. Landscape insights are crucial both for problem understanding as well as for assessing benchmark set diversity and composition. Despite the irrefutable usefulness of these features, they suffer from their own ailments and downsides. Hence, in this work we provide a collection of different approaches to characterize optimization landscapes. Similar to conventional landscape features, we require a small initial sample. However, instead of computing features based on that sample, we develop alternative representations of the original sample. These range from point clouds to 2D images and, therefore, are entirely feature-free. We demonstrate and validate our devised methods on the BBOB testbed and predict, with the help of Deep Learning, the high-level, expertbased landscape properties such as the degree of multimodality and the existence of funnel structures. The quality of our approaches is on par with methods relying on the traditional landscape features. Thereby, we provide an exciting new perspective on every research area which utilizes problem information such as problem understanding and algorithm design as well as automated algorithm configuration and selection.
更多查看译文
关键词
Deep Learning,Fitness Landscape,Exploratory Landscape Analysis,Continuous Black-Box Optimization
AI 理解论文
溯源树
样例
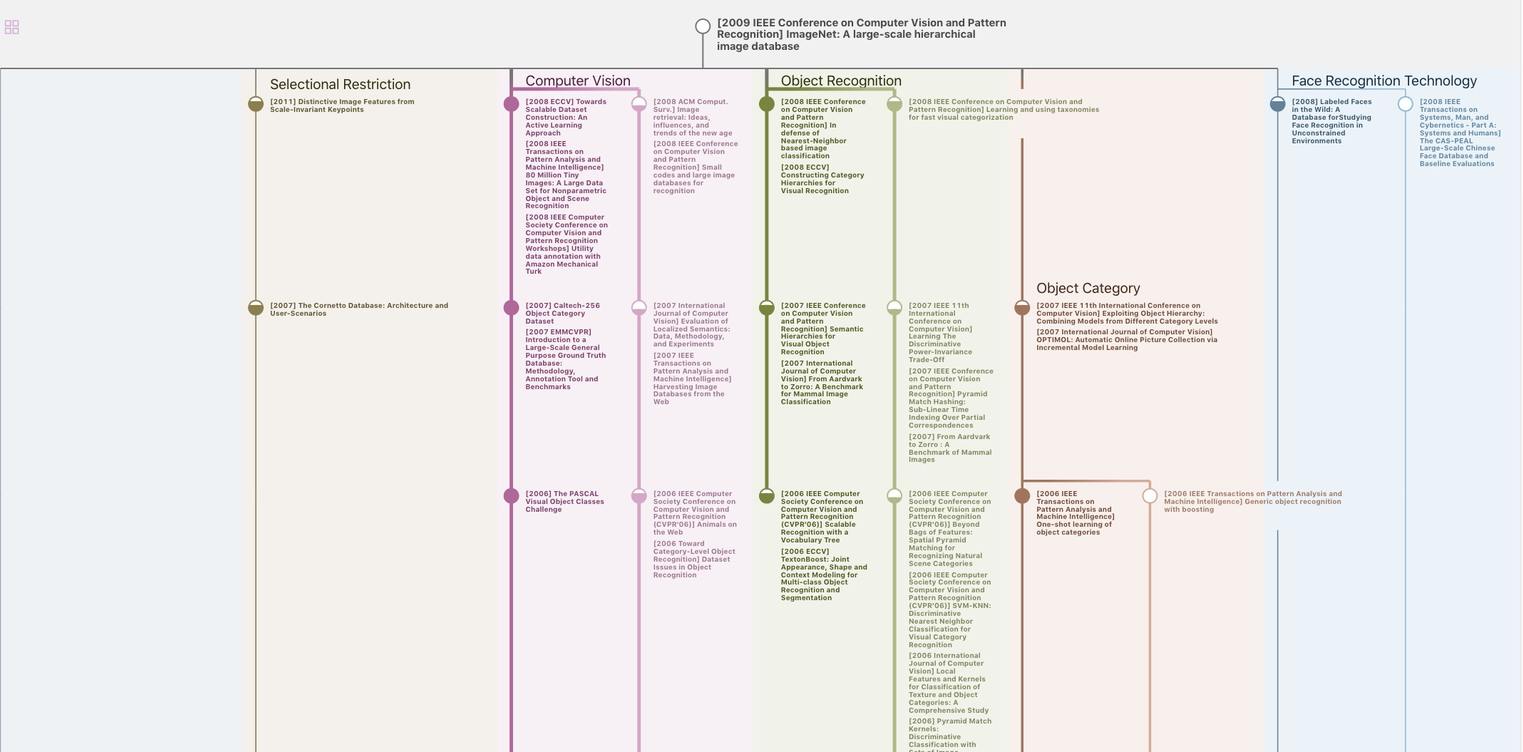
生成溯源树,研究论文发展脉络
Chat Paper
正在生成论文摘要