Text-Driven Separation of Arbitrary Sounds
Conference of the International Speech Communication Association (INTERSPEECH)(2022)
摘要
We propose a method of separating a desired sound source from a single-channel mixture, based on either a textual description or a short audio sample of the target source. This is achieved by combining two distinct models. The first model, SoundWords, is trained to jointly embed both an audio clip and its textual description to the same embedding in a shared representation. The second model, SoundFilter, takes a mixed source audio clip as an input and separates it based on a conditioning vector from the shared text-audio representation defined by SoundWords, making the model agnostic to the conditioning modality. Evaluating on multiple datasets, we show that our approach can achieve an SI-SDR of 9.1 dB for mixtures of two arbitrary sounds when conditioned on text and 10.1 dB when conditioned on audio. We also show that SoundWords is effective at learning co-embeddings and that our multi-modal training approach improves the performance of SoundFilter.
更多查看译文
关键词
separation,text-driven
AI 理解论文
溯源树
样例
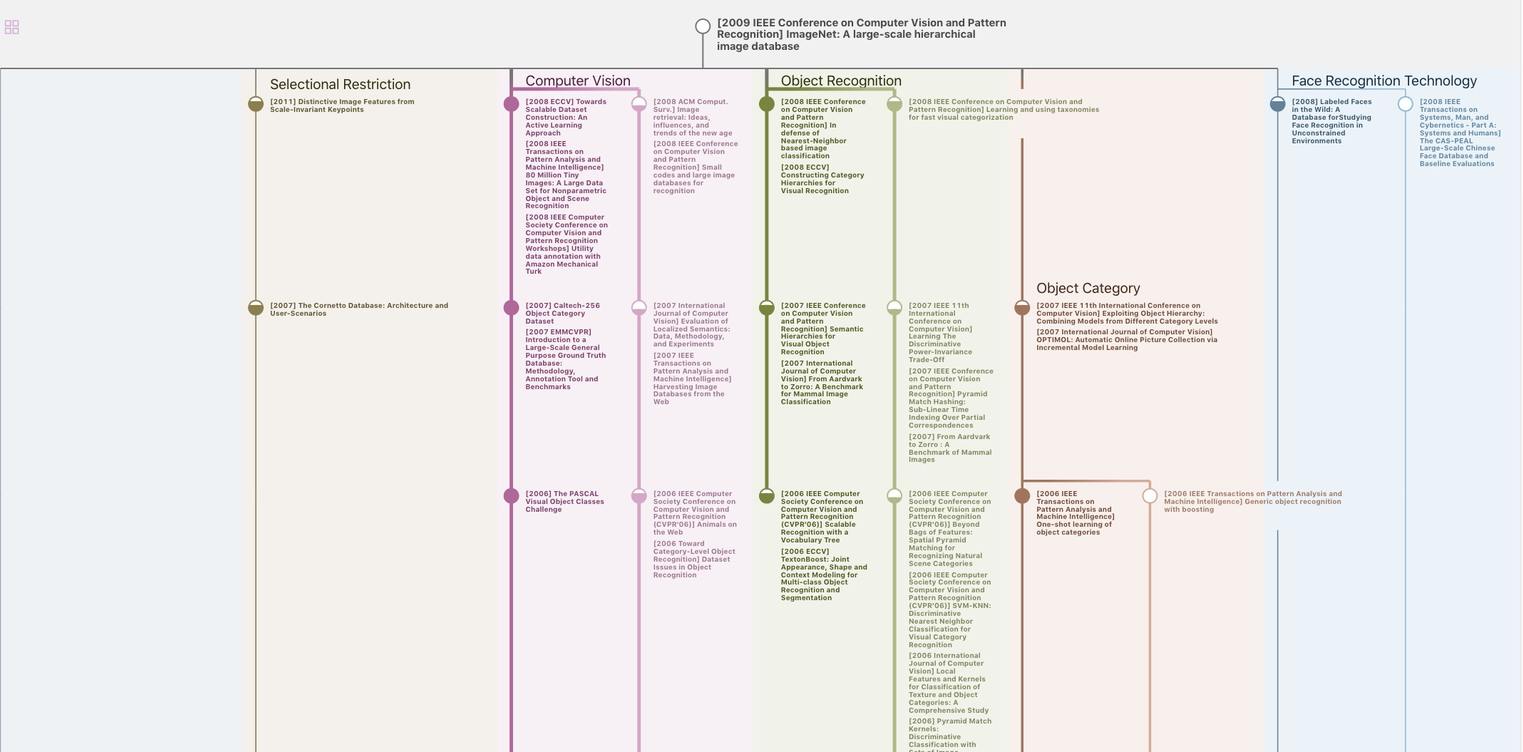
生成溯源树,研究论文发展脉络
Chat Paper
正在生成论文摘要