A General Compressive Sensing Construct Using Density Evolution
IEEE TRANSACTIONS ON SIGNAL PROCESSING(2024)
摘要
This paper proposes a general framework to design a sparse sensing matrix A is an element of R-mxn, for a linear measurement system y = Ax(#) + w, where y is an element of R-m, x(#) is an element of R-n, and w is an element of R-m denote the measurements, the signal with certain structures, and the measurement noise, respectively. By viewing the signal reconstruction from the measurements as a message passing algorithm over a graphical model, we leverage tools from coding theory, namely the density evolution technique, and provide a framework for the design of matrix A. Two design schemes for the sensing matrix, namely, (i) a regular sensing and (ii) a preferential sensing, are proposed and are incorporated into a single framework. As illustration, we consider the Lasso regression, ridge regression, and elastic net regression; and show that our framework can reproduce the classical results on the minimum sensor number, i.e., m. In the preferential sensing scenario, we consider the case in which the whole signal is divided into two disjoint parts, namely, high-priority part x(H)(#) and low-priority part x(L)(#). Then, by formulating the sensing system design as a bi-convex optimization problem, we obtain sensingmatrices which can provide a preferential treatment for x(H)(#). Numerical experiments with both synthetic data and real-world data are also provided to verify the effectiveness of our design scheme.
更多查看译文
关键词
Compressed sensing,graphical models
AI 理解论文
溯源树
样例
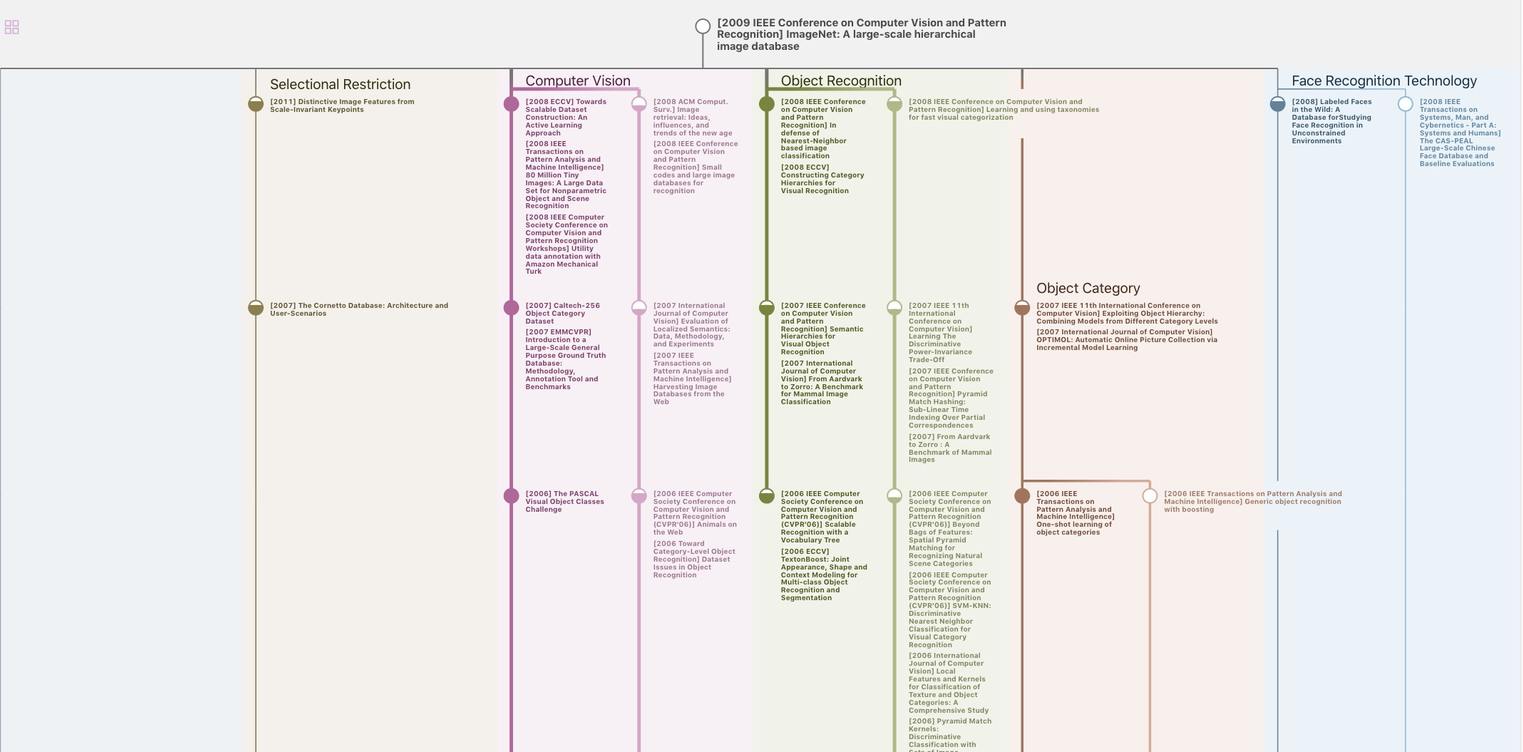
生成溯源树,研究论文发展脉络
Chat Paper
正在生成论文摘要