DualPrompt: Complementary Prompting for Rehearsal-Free Continual Learning.
European Conference on Computer Vision(2022)
摘要
Continual learning aims to enable a single model to learn a sequence of tasks without catastrophic forgetting. Top-performing methods usually require a rehearsal buffer to store past pristine examples for experience replay, which, however, limits their practical value due to privacy and memory constraints. In this work, we present a simple yet effective framework, DualPrompt, which learns a tiny set of parameters, called prompts, to properly instruct a pre-trained model to learn tasks arriving sequentially without buffering past examples. DualPrompt presents a novel approach to attach complementary prompts to the pre-trained backbone, and then formulates the objective as learning task-invariant and task-specific “instructions”. With extensive experimental validation, DualPrompt consistently sets state-of-the-art performance under the challenging class-incremental setting. In particular, DualPrompt outperforms recent advanced continual learning methods with relatively large buffer sizes. We also introduce a more challenging benchmark, Split ImageNet-R, to help generalize rehearsal-free continual learning research. Source code is available at https://github.com/google-research/l2p.
更多查看译文
关键词
Continual learning,Rehearsal-free,Prompt-based learning
AI 理解论文
溯源树
样例
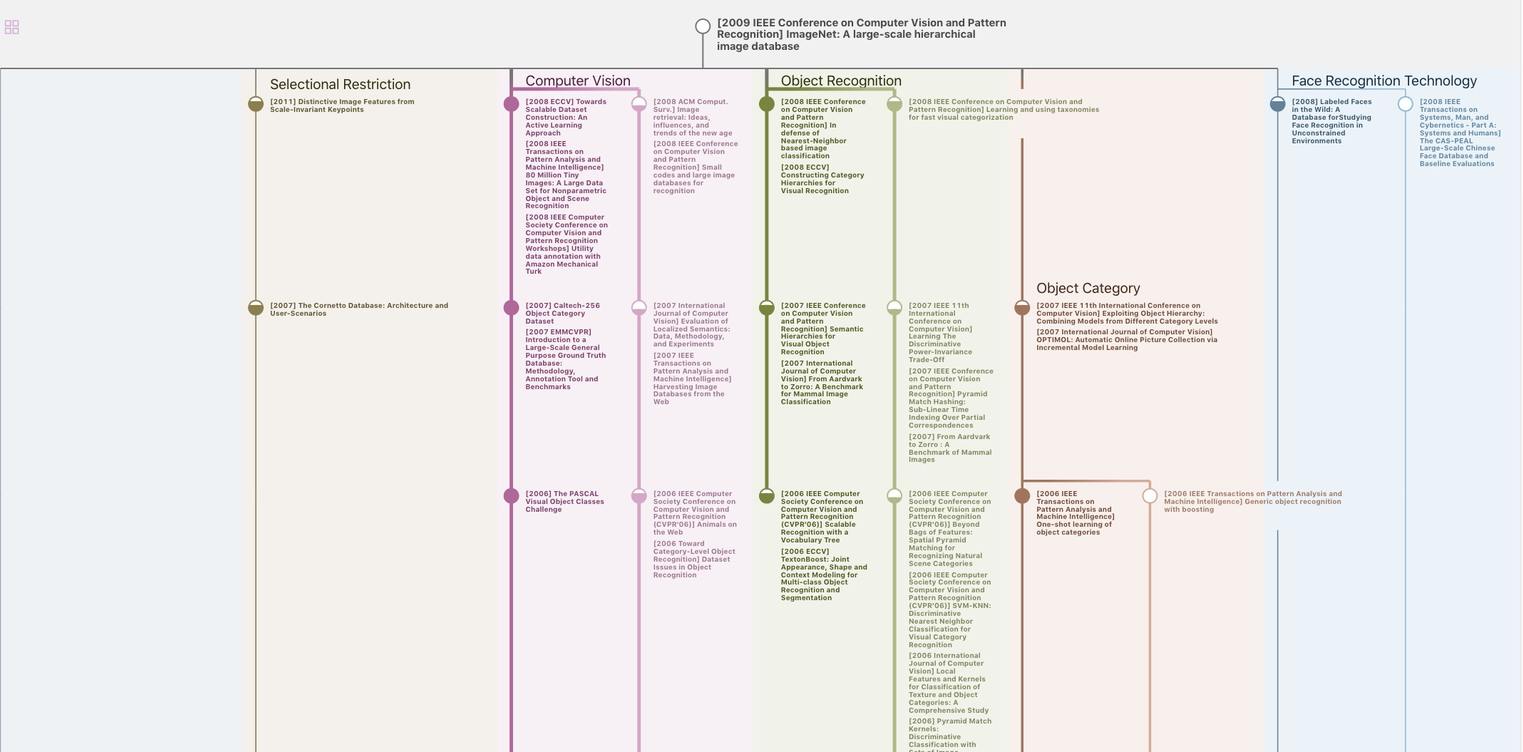
生成溯源树,研究论文发展脉络
Chat Paper
正在生成论文摘要