IDPG: An Instance-Dependent Prompt Generation Method
North American Chapter of the Association for Computational Linguistics (NAACL)(2022)
摘要
Prompt tuning is a new, efficient NLP transfer learning paradigm that adds a task-specific prompt in each input instance during the model training stage. It freezes the pre-trained language model and only optimizes a few task-specific prompts. In this paper, we propose a conditional prompt generation method to generate prompts for each input instance, referred to as the Instance-Dependent Prompt Generation (IDPG). Unlike traditional prompt tuning methods that use a fixed prompt, IDPG introduces a lightweight and trainable component to generate prompts based on each input sentence. Extensive experiments on ten natural language understanding (NLU) tasks show that the proposed strategy consistently outperforms various prompt tuning baselines and is on par with other efficient transfer learning methods such as Compacter while tuning far fewer model parameters.
更多查看译文
关键词
generation,instance-dependent
AI 理解论文
溯源树
样例
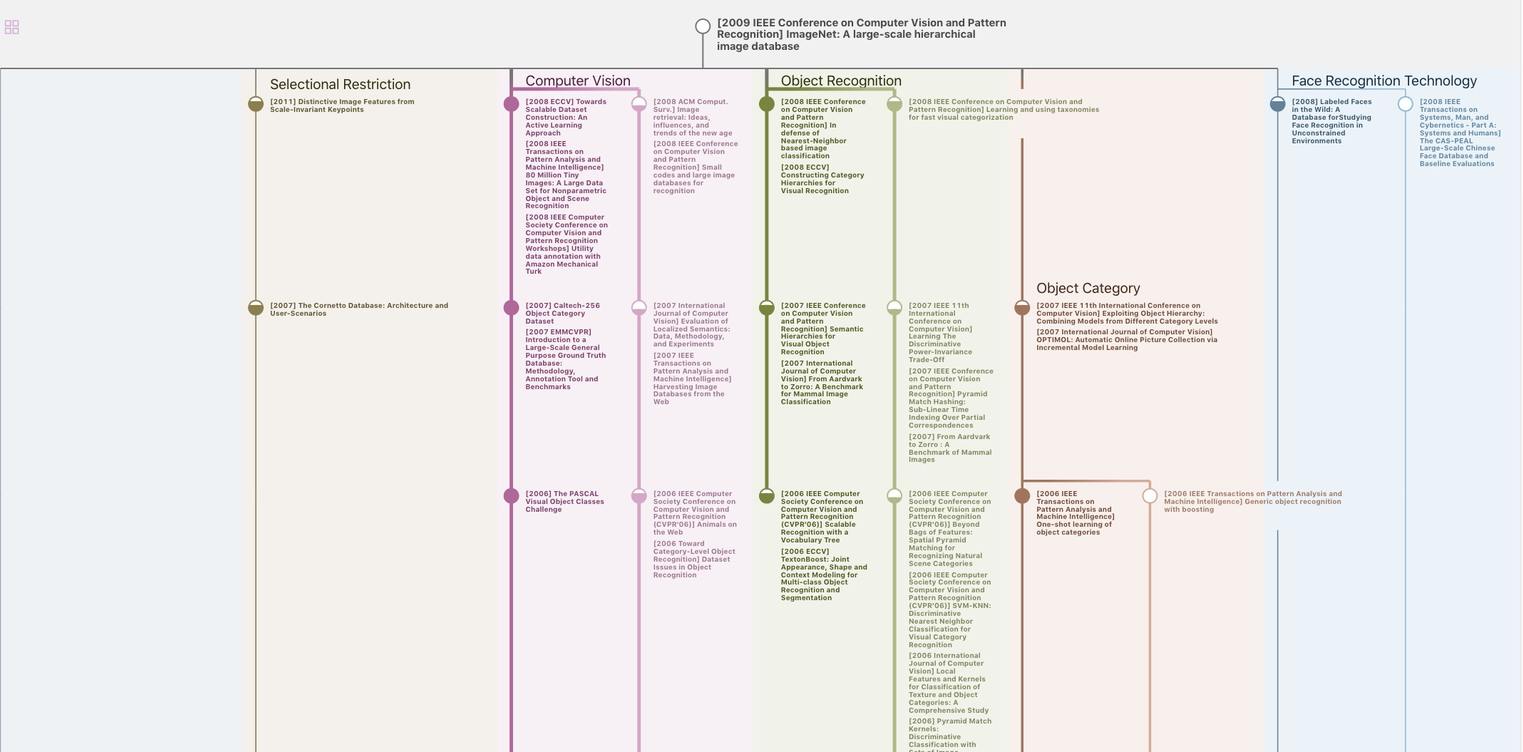
生成溯源树,研究论文发展脉络
Chat Paper
正在生成论文摘要