E^2TAD: An Energy-Efficient Tracking-based Action Detector
arXiv (Cornell University)(2022)
摘要
Video action detection (spatio-temporal action localization) is usually the starting point for human-centric intelligent analysis of videos nowadays. It has high practical impacts for many applications across robotics, security, healthcare, etc. The two-stage paradigm of Faster R-CNN inspires a standard paradigm of video action detection in object detection, i.e., firstly generating person proposals and then classifying their actions. However, none of the existing solutions could provide fine-grained action detection to the "who-when-where-what" level. This paper presents a tracking-based solution to accurately and efficiently localize predefined key actions spatially (by predicting the associated target IDs and locations) and temporally (by predicting the time in exact frame indices). This solution won first place in the UAV-Video Track of 2021 Low-Power Computer Vision Challenge (LPCVC).
更多查看译文
关键词
action,energy-efficient,tracking-based
AI 理解论文
溯源树
样例
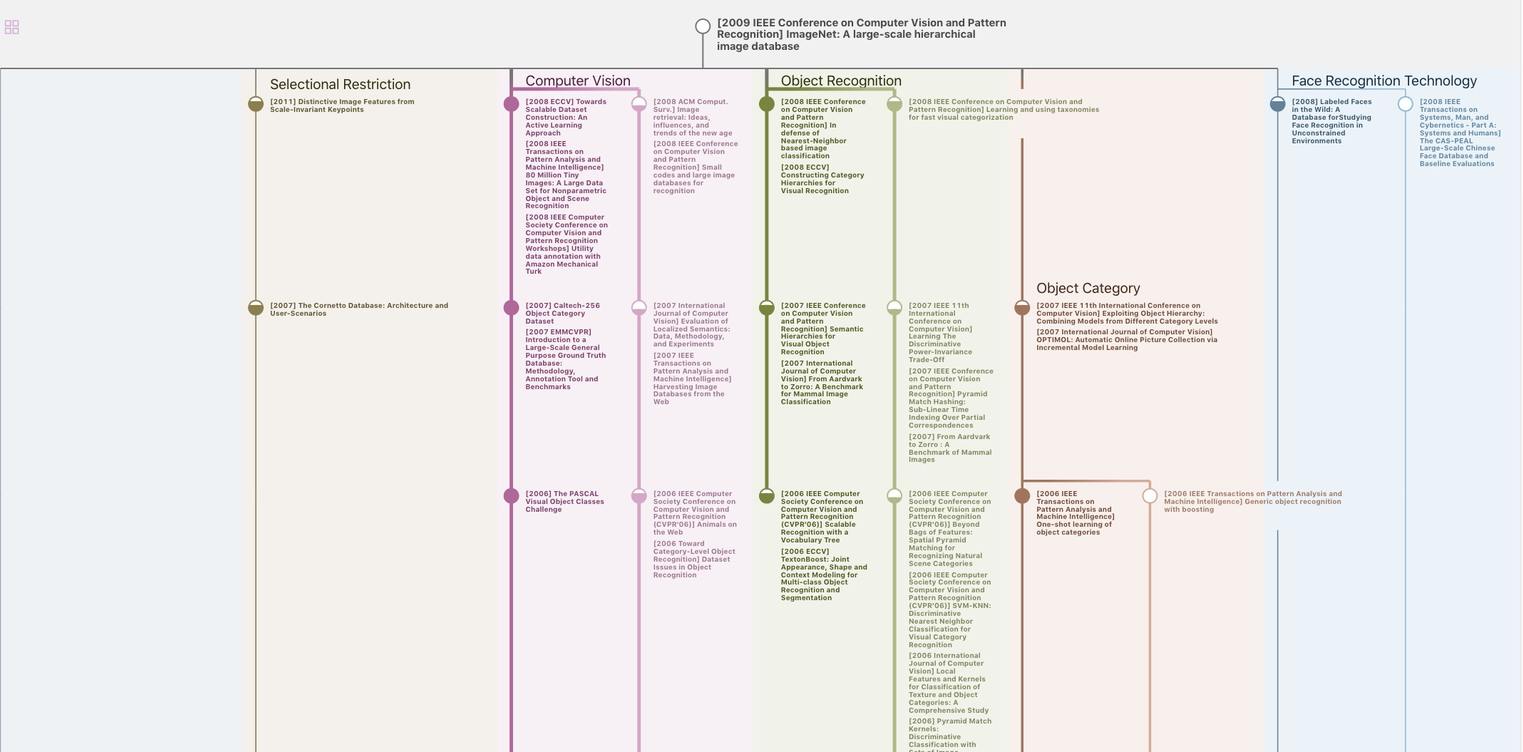
生成溯源树,研究论文发展脉络
Chat Paper
正在生成论文摘要