Data-driven prediction of forging outcome: Effect of preform shape on plastic strain in a magnesium alloy forging
Materials Today Communications(2022)
摘要
Closed-die cast-forging operations require a deliberate design of forging preforms (work-piece) to encourage process-related grain refinement and good metal flow during deformation. To this end, we propose a computational design framework and use artificial neural networks (ANNs) for generating and predicting the forging response of preform shapes, respectively. First, we develop a parametric CAD model to generate preforms algorithmically. The input space of this parametric CAD model is then uniformly sampled to generate a set of 3D models for offline finite element method (FEM) simulations. Finally, forging simulation data is processed to create a dataset for training feedforward ANNs to predict average effective plastic strain response in spatially varying regions of interest within the forging. In this study, we applied the computational design framework to generate preforms for a closed-die cast-forging operation of a Magnesium (Mg) alloy I-beam, and we predicted the average effective plastic strain response in spatially varying regions of the forging to within ±8% of the ground truth.
更多查看译文
关键词
Computational design,Neural network,Mg alloy,Closed-die forging,Preform shape,Finite element method
AI 理解论文
溯源树
样例
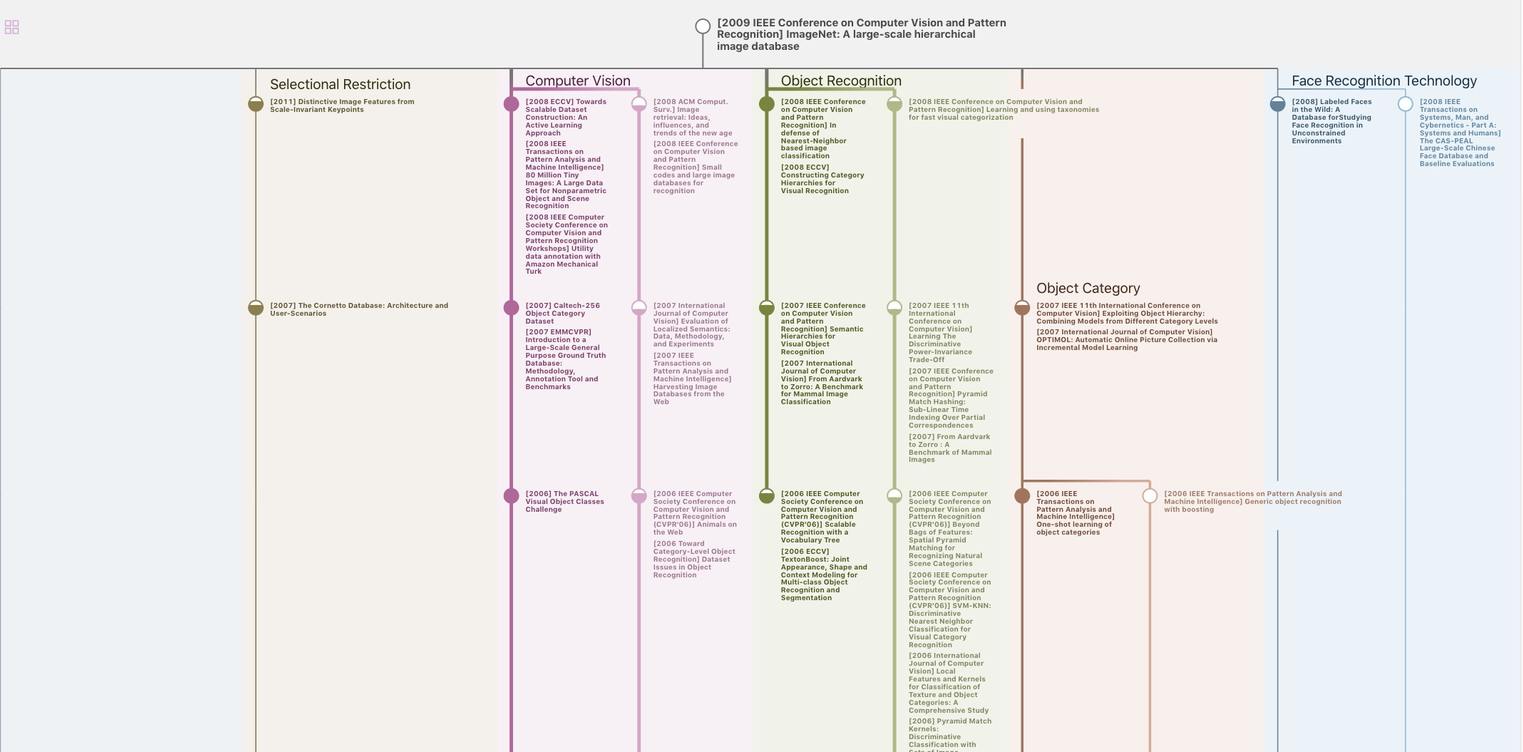
生成溯源树,研究论文发展脉络
Chat Paper
正在生成论文摘要