Spark plug defects detection based on improved Faster-RCNN algorithm.
JOURNAL OF X-RAY SCIENCE AND TECHNOLOGY(2022)
Abstract
The objective of this study is to apply an improved Faster-RCNN model in order to solve the problems of low detection accuracy and slow detection speed in spark plug defect detection. In detail, an attention module based symmetrical convolutional network (ASCN) is designed as the backbone to extract multi-scale features. Then, a multi-scale region generation network (MRPN), in which InceptionV2 is used to achieve sliding windows of different scales instead of a single sliding window, is proposed and tested. Additionally, a dataset of X-ray spark plug images is established, which contains 1,402 images. These images are divided into two subsets with a ratio of 4:1 for training and testing the improved Faster-RCNN model, respectively. The proposed model is transferred and learned on the pre-training model of MS COCO dataset. In the test experiments, the proposed method achieves an average accuracy of 89% and a recall of 97%. Compared with other Faster-RCNN models, YOLOv3, SSD and RetinaNet, our proposed new method improves the average accuracy by more than 6% and the recall by more than 2%. Furthermore, the new method can detect at 20fps when the input image size is 1024×1024×3 and can also be used for real-time automatic detection of spark plug defects.
MoreTranslated text
Key words
Defect detection, attention module, inceptionV2, faster-RCNN, spark plug
AI Read Science
Must-Reading Tree
Example
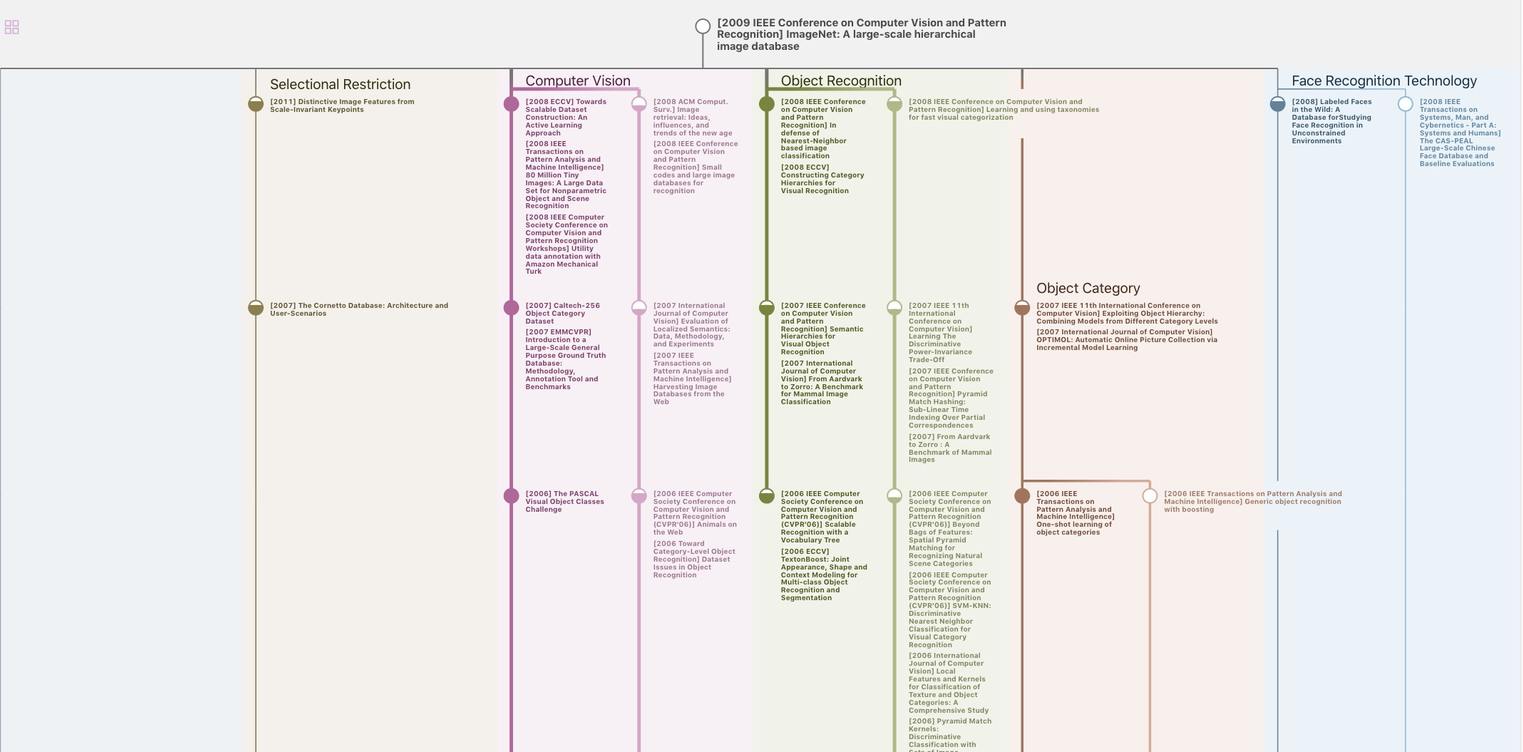
Generate MRT to find the research sequence of this paper
Chat Paper
Summary is being generated by the instructions you defined