Sharing personal ECG time-series data privately
JOURNAL OF THE AMERICAN MEDICAL INFORMATICS ASSOCIATION(2022)
摘要
Objective Emerging technologies (eg, wearable devices) have made it possible to collect data directly from individuals (eg, time-series), providing new insights on the health and well-being of individual patients. Broadening the access to these data would facilitate the integration with existing data sources (eg, clinical and genomic data) and advance medical research. Compared to traditional health data, these data are collected directly from individuals, are highly unique and provide fine-grained information, posing new privacy challenges. In this work, we study the applicability of a novel privacy model to enable individual-level time-series data sharing while maintaining the usability for data analytics. Methods and materials We propose a privacy-protecting method for sharing individual-level electrocardiography (ECG) time-series data, which leverages dimensional reduction technique and random sampling to achieve provable privacy protection. We show that our solution provides strong privacy protection against an informed adversarial model while enabling useful aggregate-level analysis. Results We conduct our evaluations on 2 real-world ECG datasets. Our empirical results show that the privacy risk is significantly reduced after sanitization while the data usability is retained for a variety of clinical tasks (eg, predictive modeling and clustering). Discussion Our study investigates the privacy risk in sharing individual-level ECG time-series data. We demonstrate that individual-level data can be highly unique, requiring new privacy solutions to protect data contributors. Conclusion The results suggest our proposed privacy-protection method provides strong privacy protections while preserving the usefulness of the data.
更多查看译文
关键词
data privacy, ECG data, time-series, data sharing, predictive analytics
AI 理解论文
溯源树
样例
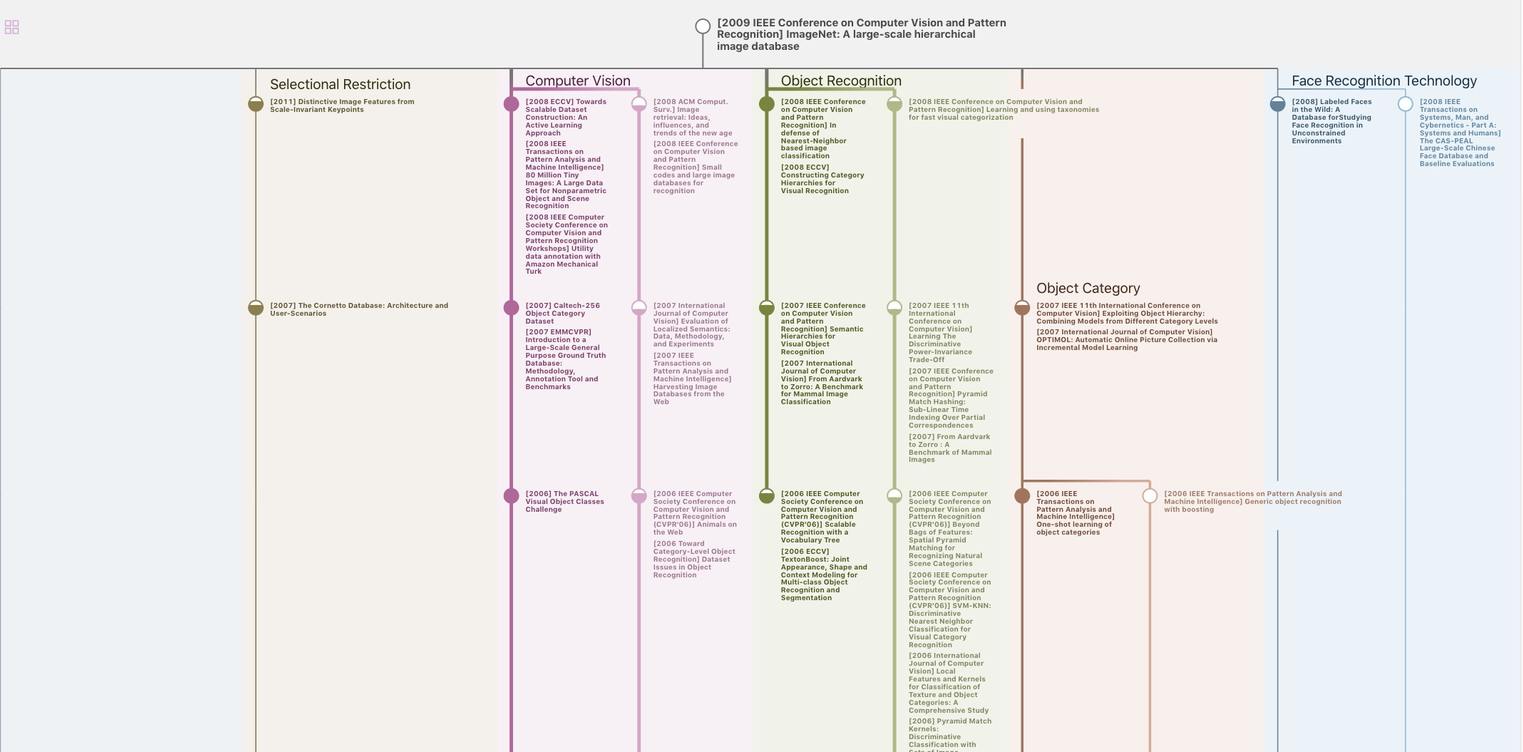
生成溯源树,研究论文发展脉络
Chat Paper
正在生成论文摘要