Global-prior-guided fusion network for salient object detection
Expert Systems with Applications(2022)
摘要
RGB image and depth map (RGB-D)-based salient object detection (SOD) has been well-studied in recent years, especially using deep neural networks. An RGB image provides rich local and semantic features, while the depth map provides global structural information. Many researchers have treated depth information as a supplement to RGB maps. However, depth maps in various datasets are not as precise as RGB information, as they are captured under various conditions. Therefore, thoroughly exploiting these features at different levels remains unresolved. Many cognitive theories, such as the topological perception theory, claim that global properties are prior to local ones and are important for human cognition. In this paper, we propose a novel global-prior-guided fusion network with global-prior extraction modules to fuse cross-modality features. Each module contains a cross attention guided by deeper global priors, and the global prior extracted by this module is used to guide the processing of local features in shallow layers. The global guided network first integrates the local and global cross features into the decoder of depth maps, and then the fused structural features of the decoder are finally fused into the saliency decoder. Experimental results show that our method outperformed other state-of-the-art methods in the RGB-D-based SOD task on seven datasets (i.e., DUT-RGBD, NJUD, LFSD, NLPR, RGBD135, SIP, and STERE) and in terms of most metrics. To thoroughly exploit the modules we designed, we extended our model to accomplish the tasks of RGB and video SOD with slight adaptions, and obtained results comparable to those of the state-of-the-art (SOTA) methods in both fields.
更多查看译文
关键词
Global prior,RGB-D salient object detection,Saliency detection,Global fusion strategy
AI 理解论文
溯源树
样例
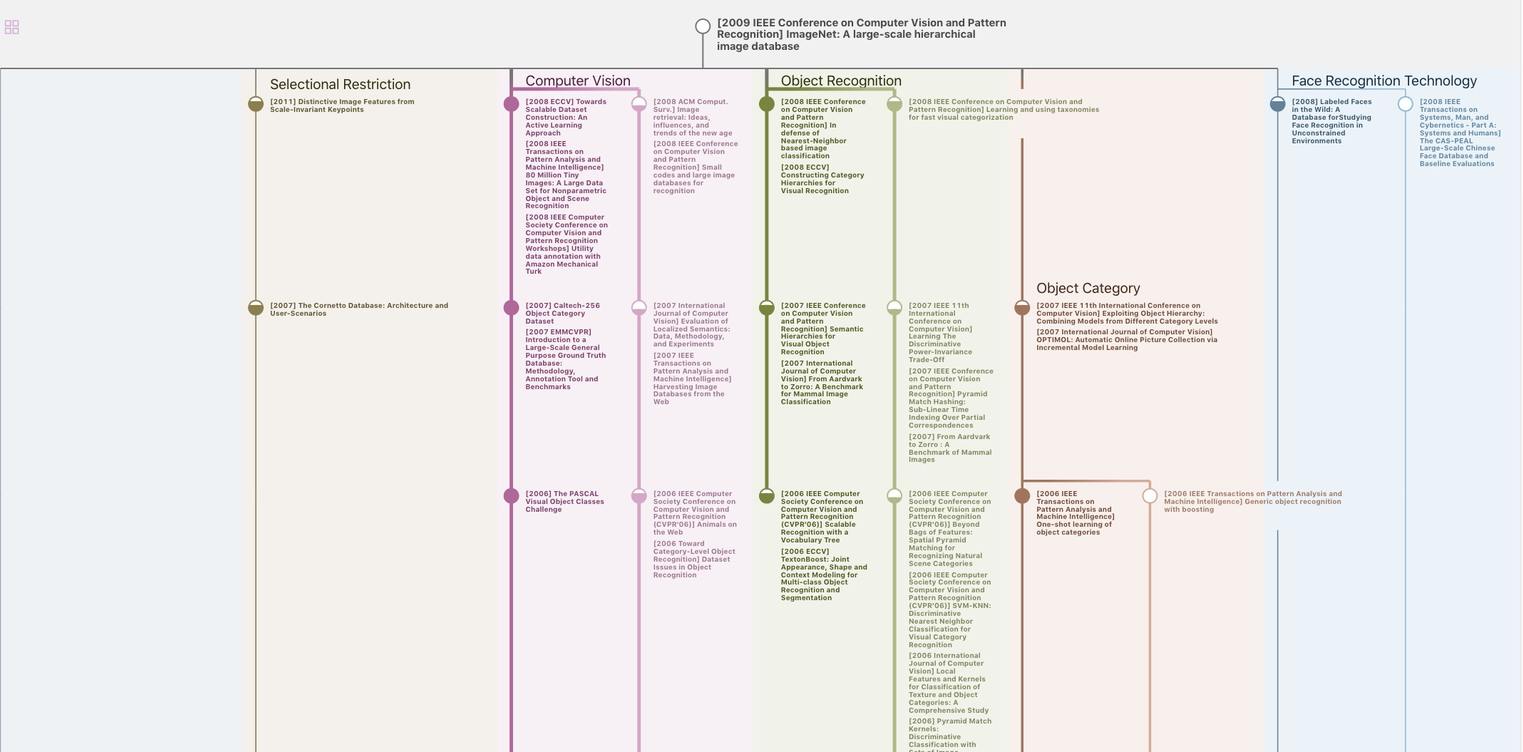
生成溯源树,研究论文发展脉络
Chat Paper
正在生成论文摘要