A spiking neural network model for the control of oscillatory phase differences between multiple brain areas
bioRxiv (Cold Spring Harbor Laboratory)(2022)
摘要
The causal mechanisms which underlie rapid, transient, and dynamic coordination of large-scale functional brain networks remain unknown. Neuronal oscillations have been hypothesized to play a critical role influencing long-range communication between brain areas, with oscillatory phase differences influencing the effective connectivity between areas ( [Fries, 2005][1] ). However, it remains unclear how networks of spiking neurons could causally and mechanistically control the relative phase difference between cortical regions. Here we describe a simple model of spiking neurons that can dynamically control the relative phase differences between multiple brain areas. The model applies to any inhibition-based rhythm, and has four essential elements: a) tonic excitation of local recurrent networks of excitatory and inhibitory cells produces oscillations ( [Börgers et al., 2012][2] ); b) winner-take-all (WTA) dynamics permit only a small fraction of cells to fire on any given cycle ( [de Almeida et al., 2009][3] ); c) di-synaptic feed-forward inhibition leads to oscillatory phase resetting in a target area ( [Traub et al., 1996][4] ); and d) the range of conduction delays for inter-regional axonal projections span the duration of one oscillatory cycle ( [Swadlow, 1998][5] ). The key assumption of our model is to link WTA dynamics to conduction delays, such that only axons with a narrow range of delays are active at one time and are selected via local activity. Phase resetting in the target area converts this conduction delay into a specific phase difference. Thus, the model converts local differences in cellular excitation into inter-areal phase differences via WTA dynamics and feed-forward inhibition, where excitatory cells act as phase difference effectors or detectors. Including several WTA modules within a given area allows it to serve as a coordinating hub, controlling the relative phase differences of multiple distributed brain regions. Finally, we show how this model can help explains recent empirical results showing that individual neurons are sensitive to the relative phase difference between areas ( [Canolty et al., 2010][6] ).
### Competing Interest Statement
The authors have declared no competing interest.
[1]: #ref-13
[2]: #ref-4
[3]: #ref-1
[4]: #ref-39
[5]: #ref-36
[6]: #ref-6
更多查看译文
关键词
spiking neural network model,oscillatory phase differences,brain,neural network
AI 理解论文
溯源树
样例
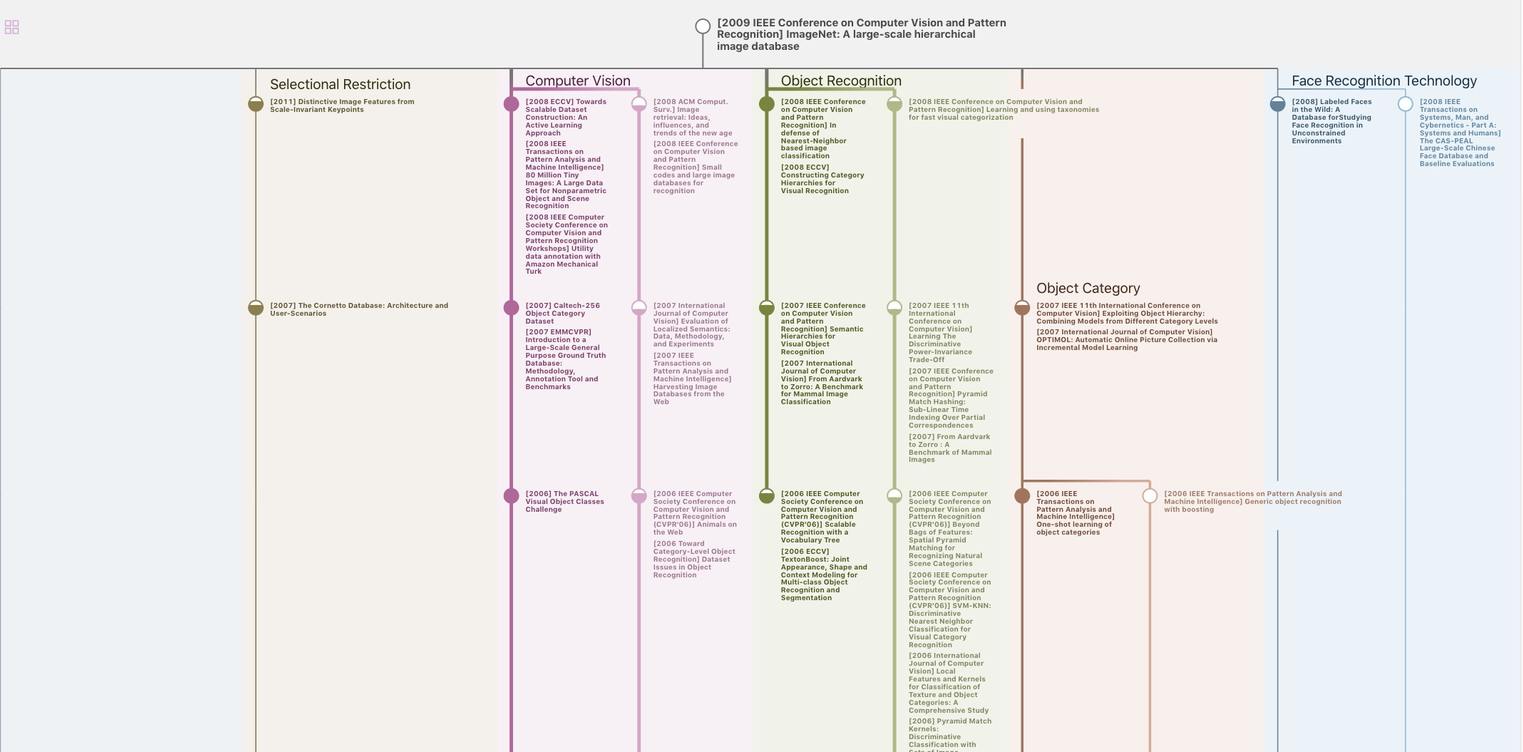
生成溯源树,研究论文发展脉络
Chat Paper
正在生成论文摘要