Quantum Machine Learning Framework for Virtual Screening in Drug Discovery: a Prospective Quantum Advantage
arxiv(2022)
摘要
Machine Learning (ML) for Ligand Based Virtual Screening (LB-VS) is an important in-silico tool for discovering new drugs in a faster and cost-effective manner, especially for emerging diseases such as COVID-19. In this paper, we propose a general-purpose framework combining a classical Support Vector Classifier (SVC) algorithm with quantum kernel estimation for LB-VS on real-world databases, and we argue in favor of its prospective quantum advantage. Indeed, we heuristically prove that our quantum integrated workflow can, at least in some relevant instances, provide a tangible advantage compared to state-of-art classical algorithms operating on the same datasets, showing strong dependence on target and features selection method. Finally, we test our algorithm on IBM Quantum processors using ADRB2 and COVID-19 datasets, showing that hardware simulations provide results in line with the predicted performances and can surpass classical equivalents.
更多查看译文
关键词
quantum machine learning,quantum kernel methods,machine learning,support vector classifier,drug discovery,ligand based
AI 理解论文
溯源树
样例
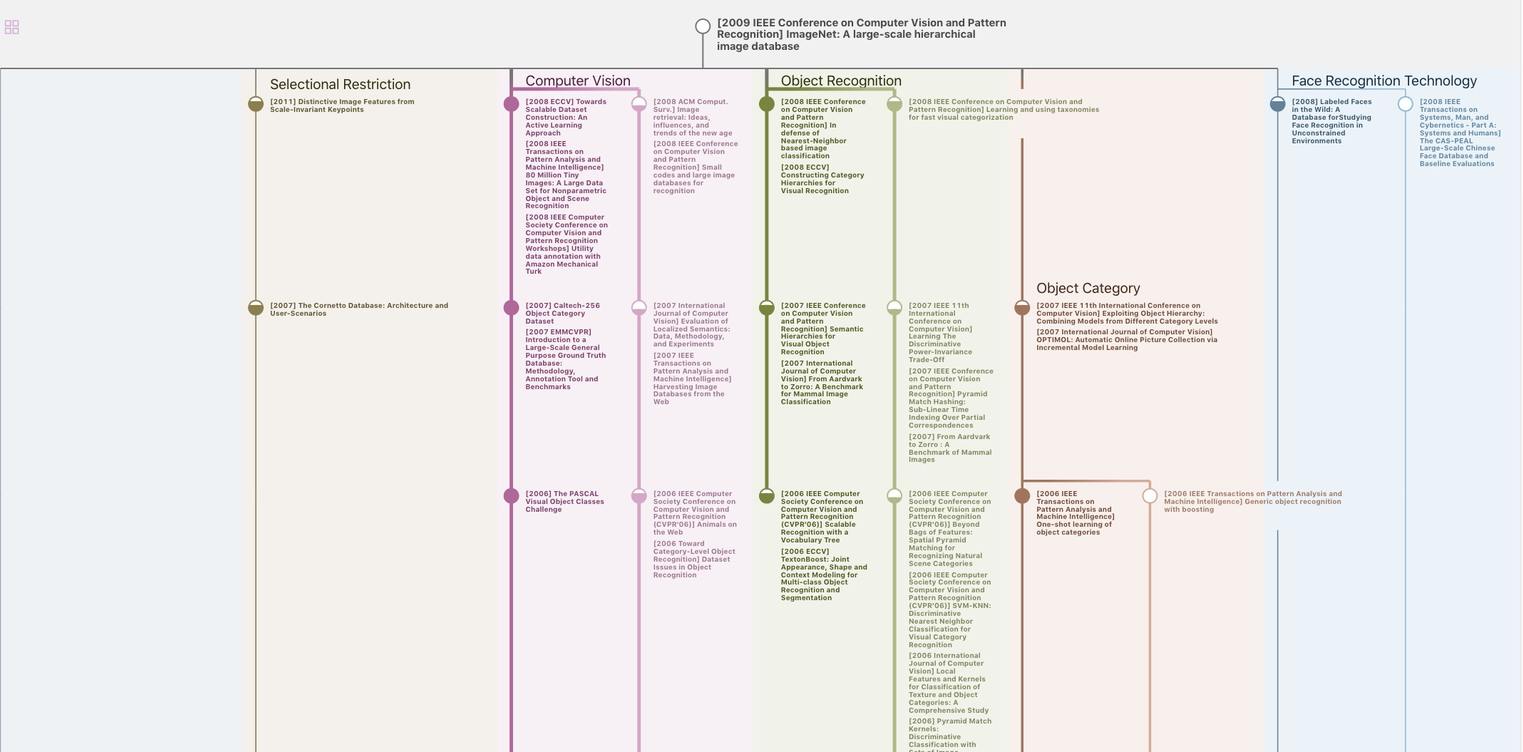
生成溯源树,研究论文发展脉络
Chat Paper
正在生成论文摘要