Thresholding Gini Variable Importance with a single trained Random Forest: An Empirical Bayes Approach
bioRxiv (Cold Spring Harbor Laboratory)(2023)
摘要
Background Random Forests (RF) are a widely used modelling tool, enabling feature-selection via a variable importance measure. For this, a threshold is required that separates label-associated features from false positives. In the absence of a good understanding of the characteristics of the variable importance measures, current approaches attempt to select features by training multiple RFs to generate statistical power via a permutation null, employ recursive feature elimination or a combination of both. However, for high-dimensional datasets, such as genome data with millions of variables, this is computationally infeasible.
Method We present RFlocalfdr, a statistical approach for thresholding that identifies which features are significantly associated with the prediction label and reduces false positives. It builds on the empirical Bayes argument of [Efron (2005)][1] and models the variable importance as mixture of two distributions – null and non-null “genes.”
Result We demonstrate on synthetic data that RFlocalfdr has an equivalent accuracy to computationally more intensive approaches, while being up to 100 times faster. RFlocalfdr is the only tested method able to successfully threshold a dataset with 6 Million features and 10,000 samples. RFlocalfdr performs analysis in real-time and is compatible with any RF implementation that returns variable importance and counts, such as ranger or VariantSpark.
Conclusion RFlocalfdr allows for robust feature selection by placing a confidence value on the predicted importance score. It does so without repeated fitting of the RF or the use of additional shadow variables and is thus usable for data sets with very large numbers of variables.
### Competing Interest Statement
The authors have declared no competing interest.
[1]: #ref-13
更多查看译文
关键词
random forest,importance
AI 理解论文
溯源树
样例
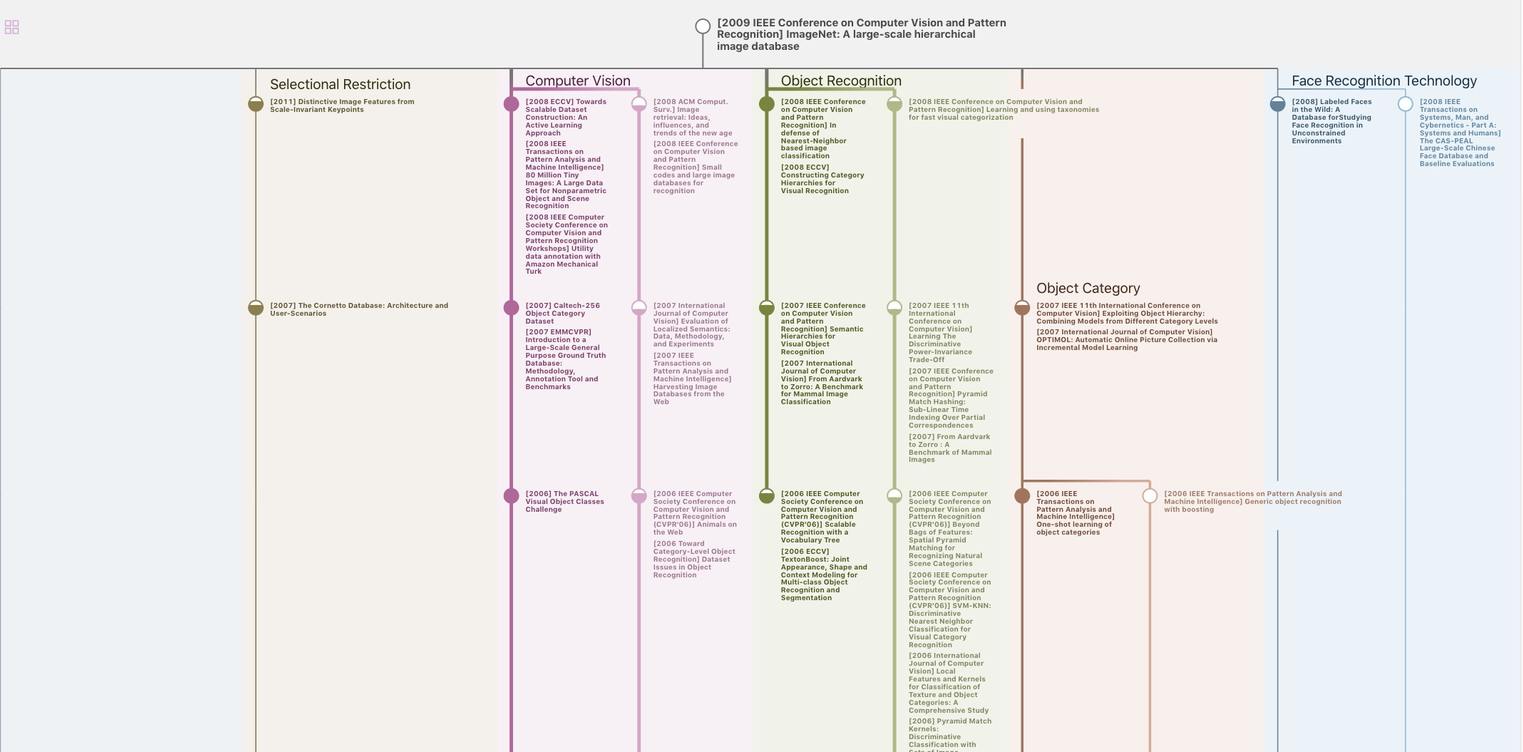
生成溯源树,研究论文发展脉络
Chat Paper
正在生成论文摘要