Evaluating Procedures for Establishing Generative Adversarial Network-based Stochastic Image Models in Medical Imaging
MEDICAL IMAGING 2022: IMAGE PERCEPTION, OBSERVER PERFORMANCE, AND TECHNOLOGY ASSESSMENT(2022)
Abstract
Modern generative models, such as generative adversarial networks (GANs), hold tremendous promise for several areas of medical imaging, such as unconditional medical image synthesis, image restoration, reconstruction and translation, and optimization of imaging systems. However, procedures for establishing stochastic image models (SIMs) using GANs remain generic and do not address specific issues relevant to medical imaging. In this work, canonical SIMs that simulate realistic vessels in angiography images are employed to evaluate procedures for establishing SIMs using GANs. The GAN-based SIM is compared to the canonical SIM based on its ability to reproduce those statistics that are meaningful to the particular medically realistic SIM considered. It is shown that evaluating GANs using classical metrics and medically relevant metrics may lead to different conclusions about the fidelity of the trained GANs. This work highlights the need for the development of objective metrics for evaluating GANs.
MoreTranslated text
Key words
Generative adversarial networks,objective image quality assessment,image perception,stochastic,image models
AI Read Science
Must-Reading Tree
Example
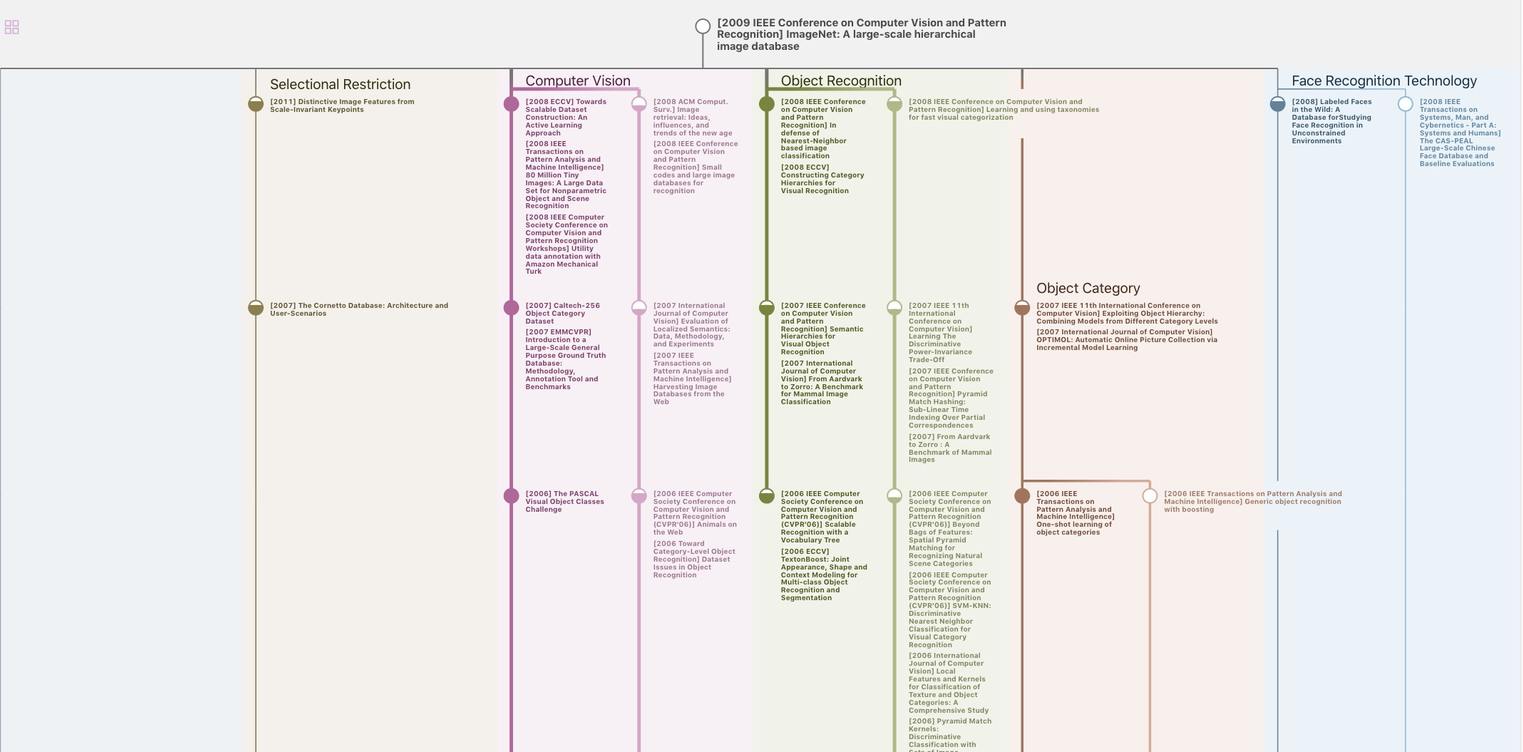
Generate MRT to find the research sequence of this paper
Chat Paper
Summary is being generated by the instructions you defined